A Detailed Guide To Best Practices In Image Annotation
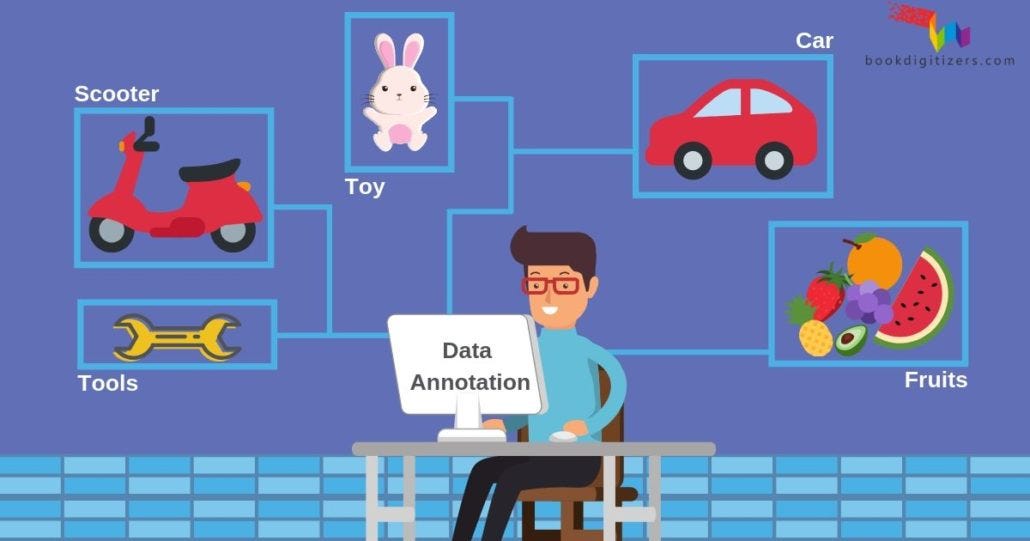
In our previous blogs, we learn about what image annotation is and saw an overview of where Image Annotation can be applied.
Image annotation plays a pivotal role in the training of machine learning models, as the accuracy of annotations is essential for your model's ability to identify objects in images and videos correctly.
To ensure the smooth and efficient execution of your image annotation projects, it is important to adhere to the following practices. These guidelines will contribute to the development of machine learning models that are not only reliable but also diverse in their capabilities.
Table of Contents
- Best Practices to Follow During Image Annotations
- Establish a Well-defined Labeling Strategy
- Ensuring Data Quality and Accuracy
- Standardization and Consistency in Annotation
- Training Annotators for High-Quality Results
- Ensuring Data Security and Privacy Guidelines
- What Exactly is Data Security and Data Privacy?
Best Practices to Follow During Image Annotations
Establishing a well-defined labeling strategy is imperative for optimizing image annotation projects.
Without a structured plan, valuable time might be wasted on repetitive tasks or the chance to improve model performance could be missed. This strategy should be customized to match your labeling objectives, the dataset at hand, and the specific labels required.
Consistency is key when labeling data, from maintaining a uniform format and terminology to ensuring capitalization and punctuation are consistent throughout.
In complex cases where clear labels are hard to obtain, a semi-supervised approach can be explored using a combination of labeled and unlabeled data. This strategic approach lays the foundation for accuracy and precision in data labeling, thereby enhancing the quality of machine-learning models.
Below, we discuss each important aspect in detail.
Establish a well-defined labeling strategy
In the absence of a structured plan, you risk investing time in monotonous tasks or overlooking labeling opportunities that could enhance your model's performance. An effective labeling strategy should be tailored to your labeling objectives, the available dataset, and the nature of the labels you intend to assign.
Maintain clarity and consistency throughout the image annotation projects when labeling your data. Employ a uniform format and terminology for all labels. For instance, if a location is referred to as a "city" in one instance, refrain from using a different term like "town" elsewhere.
Consistency in capitalization and punctuation is crucial. Decide whether it's "New York," "New York City," "state legislature," or "State Legislature," and adopt a consistent convention that aligns with your project documents.
In cases where obtaining clear labels for all classes proves challenging, you might explore a semi-supervised approach. This entails utilizing a combination of labeled and unlabeled data in your analysis.
Figure: Ensuring Accuracy and Consistency are the keys to Optimal Data
Ensuring Data Quality and Accuracy
The potential of your annotations has a direct bearing on your model's accuracy, underscoring the importance of investing in top-tier training datasets. For instance, when tasked with labeling a collection of images, it is imperative that the dataset encompasses a comprehensive array of diverse instances related to the labeling objective.
Try training data that encompasses every conceivable usage scenario. This entails utilizing high-quality images captured from various perspectives, distances, and lighting conditions. These variables are instrumental in equipping models with greater resilience and precision.
Furthermore, it is of utmost importance to ensure that the annotators possess a high degree of expertise and domain knowledge in their respective fields.
Standardization and Consistency in Annotation
To check how well your machine learning model works, you need to test it with different tasks. Make a set of examples, with some that should be recognized and some that shouldn't. Then, use your annotation tool to label these examples and see how often it gets them right.
Figure: Data Quality Metrics
For instance, if you want to see how well a face recognition system works, you'd create pictures with faces (the ones it should recognize) and pictures without faces (the ones it shouldn't recognize). Then, you'd use the labeled pictures to see how often the system gets it right or wrong.
Training Annotators for High-Quality Results
Human annotators play a crucial role in data preparation, even when automated labeling processes are employed. Their importance lies in their ability to comprehend the unique requirements and nuances of each client's project.
While automated systems can certainly assist in handling large volumes of data, human annotators bring an invaluable level of understanding and context to the process.
They possess the capacity to interpret specific guidelines, adapt to project intricacies, and discern intricate details that automated tools might miss. Human annotators bridge the gap between the client's vision and the technical execution of data labeling, ensuring that the labeled data aligns with the client's precise needs and objectives.
Their expertise, adaptability, and attention to detail remain indispensable in delivering high-quality, tailored results that meet the client's expectations. For instances where some ambiguity may arise, for instance, in the case of vehicle damage detection, one also has to detect the percentage of Damage done.
Now, this could be subjective as what we label it as 20%; the client may say 25% damage. So, there to grasp client/user understanding, Human annotators play a crucial role.
Check out LabelGPT, one of the best AI Powered Annotation Tools.
Ensuring Data Security and Privacy Guidelines
Incorporating data security and data privacy into your evaluation is essential when outsourcing data annotation projects. Presently, more than 90 percent of business leaders have invested in AI and machine learning.
However, this technological progress comes with a cost, as over 62 percent of companies face challenges in complying with data regulations such as GDPR and CCPA.
Figure: Data Privacy and Data Security
With our increasing reliance on technology, there is a growing concern for the protection of data privacy and security, which is entirely justified, especially considering significant data breaches in recent years.
Data security involves safeguarding electronic information from unauthorized access. This encompasses protective measures to prevent data from being tampered with, stolen, or used without authorization.
Regarding data annotation, several compelling reasons underscore the significance of data security:
- Safeguarding the privacy of individuals whose data is being utilized.
- Preventing fraudulent or malicious exploitation of the data.
- Maintaining data accuracy and currency.
What exactly is Data Security and Data Privacy?
Data security and data privacy are often used interchangeably, but they encompass separate concepts. Data security involves safeguarding electronic data from unauthorized access, whereas data privacy pertains to individuals' rights to control the collection and utilization of their personal information.
The nexus between data privacy and data security has intensified with the emergence of significant regulations such as the General Data Protection Regulation (GDPR) and the California Consumer Privacy Act (CCPA).
Why ensure Data Privacy and Data Security?
If you're thinking about getting someone else to help with your data labeling, make sure they have good security in place. How do they protect your data from the time they get it to when they give it back to you? You should also ask how they stop bad things from happening with your data.
Sometimes, people use a method called crowdsourcing to get data labeled quickly and cheaply. But there are big problems with this:
- Quality: When you use crowdsourcing, you can't control who does the work, so the quality might not be good. You don't know if the people doing it are experienced or good at it.
- Security: Crowdsourcing can be risky because you're basically giving a lot of people access to your private data, and they might need better security. Also, if you're working with sensitive data, there's no way to make sure the people doing the work keep it a secret.
- Cost: Even though crowdsourcing might seem cheap, it can end up costing more if your data gets leaked, if the data is not good quality, or if the results are not fair.
Conclusion
In our previous blogs, we delved into the concept of image annotation and explored its diverse applications. Image annotation plays a fundamental role in training machine learning models, as the precision of annotations significantly impacts a model's ability to identify objects in images and videos correctly.
To ensure the efficiency and effectiveness of image annotation projects, it is crucial to adhere to best practices. These guidelines contribute to the development of machine learning models that are both reliable and versatile. Here are some key best practices:
- Establish a Clear Labeling Strategy: Having a well-defined plan is essential to avoid wasting time on tedious tasks and to capture labeling opportunities that enhance your model's performance. Maintain consistency in format and terminology.
- Ensure Data Quality and Accuracy: Invest in high-quality training datasets that cover a wide range of variations. Train annotators with expertise in their domains.
- Measure Data Accuracy, Reliability, and Diversity: Evaluate your machine learning model's performance by testing it on various tasks with labeled data.
- Train Annotators for High-Quality Results: Choose annotation tools that align with your project's goals and workflows. Ensure annotators are well-versed in using these tools.
- Ensure Data Security and Privacy: Incorporate data security and privacy into your evaluation, especially when outsourcing data annotation. Protect sensitive data and comply with regulations.
Data security and data privacy are distinct but intertwined concepts. With the rise of regulations like GDPR and CCPA, ensuring the safety and privacy of data has become increasingly important.
By following these best practices, you can enhance the accuracy and efficiency of your image annotation projects, ultimately leading to more reliable and diverse machine learning models.
Frequently Asked Questions
1. What are Different types of Image Annotations?
Image annotation encompasses the utilization of various methods, including but not limited to bounding boxes, masking, polygons, polylines, tracking, and transcription.
2. What is the most straightforward method for image annotation?
You can opt for an open-source or free data annotation tool, with the Computer Vision Annotation Tool (CVAT) being an open-source tool, or you can go for Labellerr, which is slightly priced but provides excellent features that simplify the ML Pipeline.

Simplify Your Data Annotation Workflow With Proven Strategies
.png)
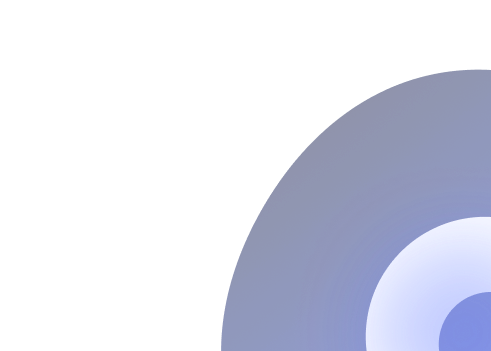