The Ultimate Guide to Image Annotation: Techniques, Tools, and Best Practices
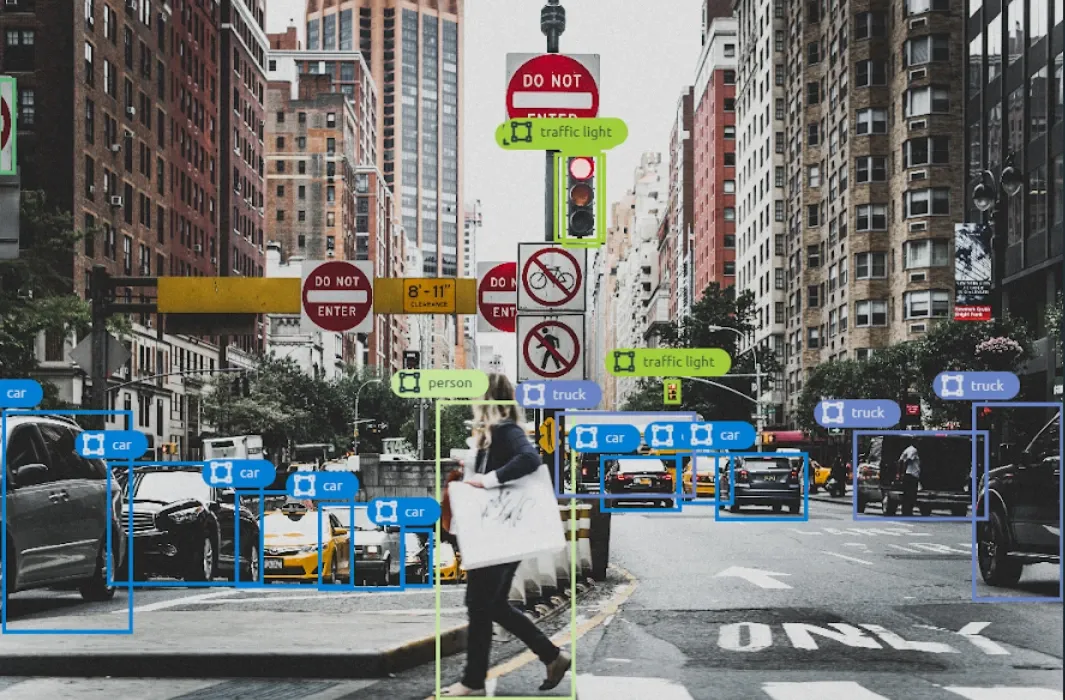
1. What is Image Annotation?
Definition and importance
Image annotation is the process of adding descriptive labels or markings to images, which are typically used for training machine learning models, especially in the field of computer vision.
These labels provide essential information to the models, allowing them to recognize and understand objects, patterns, or features within the images. Image annotation is a crucial step in the development of AI and machine learning systems, and it serves several important purposes:
1. Training Machine Learning Models
Image annotation is fundamental for supervised learning, where models learn from labeled data. By associating labels or annotations with images, the models can understand and differentiate various objects, shapes, or characteristics in the images, making them capable of making accurate predictions or classifications.
2. Object Detection and Recognition
Image annotation is essential for tasks like object detection and recognition. It allows models to identify and locate specific objects or elements within images, enabling applications such as autonomous vehicles, facial recognition, and medical image analysis.
3. Segmentation and Masking
Image annotation can involve creating detailed segment masks that precisely outline objects or regions of interest within an image. This is crucial for tasks like image segmentation, where models need to separate objects from the background or distinguish different parts of an image.
4. Quality Control
Proper image annotation ensures the accuracy and consistency of training data. Without high-quality annotations, machine learning models may produce unreliable results. Therefore, rigorous quality control and validation are often carried out during the annotation process.
5. Customization and Adaptation:
Image annotation allows customization of machine learning models to suit specific applications or industries. For instance, annotating images of medical scans with specific medical conditions allows the development of models for disease diagnosis.
6. Data Augmentation:
Annotated images can be used for data augmentation, where variations of the original dataset are created by modifying or augmenting the annotated images. This helps improve model robustness and generalization.
7. Transfer Learning:
Annotated data can be used to pre-train models, which can then be fine-tuned for specific tasks. This is particularly valuable when labeled data is scarce or expensive to acquire.
Use Cases in AI and Machine Learning
1. Healthcare
- COVID-19 Diagnosis:
Annotated medical scans, such as CT scans and MRIs, were crucial for developing AI/ML systems to detect COVID-19 based on scan results, helping correlate patient scans with their COVID-19 status.
- Face Mask Detection:
AI-based solutions were needed to detect whether individuals were wearing masks, especially during the pandemic, to enforce safety measures.
- Tumor Detection:
Annotated images depicting tumor regions help machine learning systems learn patterns for diagnosing tumors, aiding radiologists and doctors in the detection process.
2. Autonomous Vehicles
- Autonomous Driving:
Annotated images and videos in large datasets enable self-driving vehicles to identify and localize important elements for safe navigation, such as other vehicles, traffic signs, driving lanes, and more.
- Traffic Flow Analysis:
Annotated data from surveillance cameras can provide insights into traffic flow, congestion, and road conditions, aiding traffic engineers in managing roadways.
- Parking Occupancy Detection:
Annotated data helps machine learning systems predict available parking slots, enhancing parking guidance and information systems.
3. Agriculture
- Disease and Pest Detection:
Annotated data is used to localize and classify infected plants, allowing AI models to predict the presence of diseases and pests with location and severity information.
- Crop and Yield Monitoring:
Annotated datasets provide insights into crop growth, ripeness, and statistics, reducing the need for manual surveillance and helping farmers take informed actions.
- Livestock Health Monitoring:
Data characterizing various livestock animals, their health status, and diseases within them enables the development of systems to monitor livestock health and assist farmers in tracking the well-being of their animals.
In all these use cases, image annotation plays a crucial role in training machine learning models and computer vision systems.
It provides the labeled data necessary for these systems to understand and interpret visual information, making them effective tools in various industries and applications. The accurate and detailed annotations in these domains help drive innovation, improve safety, and enhance efficiency.
2. Types of Image Annotation
I. Bounding Box Annotation
Bounding boxes are a fundamental technique in computer vision that involves drawing rectangular boxes around objects of interest within an image. These boxes are widely used for various tasks, including object detection and localization. Bounding boxes provide a simple yet effective way to define the spatial location of target objects within an image.
Typically, a bounding box is defined by its coordinates in the image. The two common representations are:
1. (x1, y1, x2, y2): This representation uses the coordinates of the upper-left corner (x1, y1) and the lower-right corner (x2, y2) of the rectangular box to specify its position. It is a straightforward way to define the box's location.
2. (x1, y1, width, height): Alternatively, a bounding box can be defined by the coordinates of the upper-left corner (x1, y1) and its width (w) and height (h). This representation provides a compact way to specify the box.
The primary purpose of bounding boxes is to aid in object detection and localization tasks. By drawing boxes around objects of interest, computer vision systems can identify and locate these objects within an image.
This is crucial in applications like image classification, object recognition, and autonomous driving, where the precise location of objects is essential for decision-making.
Bounding boxes offer several advantages:
Pros:
1. Ease of Annotation: Annotating images with bounding boxes is relatively straightforward and quick. Annotators can draw boxes tightly around object edges, making it a practical choice for large datasets.
2. Object Localization: Bounding boxes provide precise information about the location of objects in an image. This information is crucial for various computer vision tasks, such as tracking and counting objects.
However, bounding boxes also have limitations:
Cons:
1. Inclusion of Background: Depending on the type and position of the object, the box might include a significant amount of background pixels that do not belong to the object. This is especially true when objects are close to each other or when they have irregular shapes.
2. Rotation Challenge: Bounding boxes are not ideal for objects that are rotated or have irregular orientations. In such cases, a rotated rectangle format that includes the angle of rotation in addition to the coordinates can be more suitable.
Bounding boxes are a commonly used and effective annotation technique in computer vision. They provide a simple and efficient way to define the spatial location of objects within an image.
While they have their limitations, bounding boxes remain a fundamental tool for various object detection and localization tasks, offering a balance between ease of annotation and precise object localization.
II. Polygon Annotation
Polygon annotation is a technique used in computer vision to outline the shape of objects within an image by defining a series of connected vertices. Unlike bounding boxes, which use rectangular shapes, polygon annotation provides a more precise boundary for objects and is commonly employed in tasks that require detailed object segmentation.
In this method of image annotation, annotators draw polygons around objects, accurately capturing their intricate shapes and sizes. The process involves identifying the object's borders within the frame with a high level of accuracy.
This precise annotation technique is crucial for identifying various objects, such as street signs, logos, and facial features in sports analytics. It enables detailed recognition and analysis of these objects within images.
Polygons offer several advantages over bounding boxes:
Pros:
1. Precision: Polygons allow annotators to include only the pixels that belong to the object, resulting in a more accurate representation of the object's shape. This precision is vital for tasks where the exact object boundaries are essential.
2. Versatility: Polygon annotation is versatile and can be applied to objects of various shapes, making it suitable for annotating complex and irregularly shaped objects. Unlike bounding boxes, polygons can accurately capture the contours of objects that do not fit well within rectangular boundaries.
However, polygon annotation also has its challenges:
Cons:
1. Complexity: Drawing polygons requires more effort and time compared to drawing bounding boxes. The process involves specifying a sequence of x, y coordinates for every point that makes up the polygon. This complexity can slow down the annotation process, especially when dealing with a large dataset.
2. Tool Support: The annotation tool used must support polygons and, in some cases, holes within polygons. For instance, annotating objects like donuts or pretzels requires the tool to handle interior and exterior coordinates separately. Ensuring proper support for these features is essential for accurate annotation.
3. Overlap Handling: When polygons overlap, annotators need to consider the order of objects and which polygon appears on top. Managing overlapping polygons can be challenging and requires careful attention to detail.
Polygon annotation is a powerful technique for object segmentation in computer vision tasks. It offers a high level of precision, making it ideal for tasks that demand accurate object boundaries. While it may be slower to annotate compared to bounding boxes, polygon annotation is essential for applications where detailed object shapes are critical for accurate analysis and recognition.
III. Semantic Segmentation
Semantic segmentation is a high-precision image annotation technique used in computer vision that assigns a pixel-level label to each pixel in an image. It involves categorizing each pixel into different object classes or regions, providing a detailed and pixel-wise understanding of the image's content.
This pixel-wise labeling allows for precise object segmentation, making it a powerful tool for various applications in computer vision and artificial intelligence.
Key points about semantic segmentation include
1. Pixel-Wise Annotation: Semantic segmentation is a pixel-wise annotation method, meaning that each pixel in an image is assigned to a specific class or category. These classes can represent objects, regions, or different semantic meanings within the image. For example, classes might include "pedestrian," "car," "bus," "road," "sidewalk," and more. Each pixel carries a semantic label that indicates its classification.
2. Detailed Object Understanding: This technique provides a highly detailed understanding of an image, as it categorizes every pixel. Unlike other annotation methods that primarily focus on object detection or localization, semantic segmentation's primary goal is to interpret and label the pixels within the image.
3. Applications: Semantic segmentation is commonly used in scenarios where environmental context is crucial for decision-making. It is vital in applications such as self-driving cars, robotics, and scene understanding. In self-driving cars, for instance, the technology helps the vehicle understand the road, identify obstacles, and make safe driving decisions.
4. Output Format: The output of semantic segmentation is typically a pixel-wise mask or map, often in the form of a PNG image where each color corresponds to a specific class. Additionally, it can be represented in other formats like JSON files with bitmap objects encoded as base64 strings.
Pros:
1. Ultra-Precision: Semantic segmentation provides an extremely high level of precision since every pixel in the image is assigned to a specific class. This precision is invaluable for tasks where object boundaries and fine-grained information are crucial.
2. Environmental Context: It is particularly useful when understanding the broader context of an image is essential. In applications like autonomous vehicles, semantic segmentation helps in making informed decisions by comprehensively labeling the surroundings.
Cons:
1. Labor-Intensive: The process of segmenting an image at the pixel level can be labor-intensive and time-consuming. Annotators need to classify each pixel accurately, which is a more demanding task compared to methods like bounding boxes or polygons.
2. Tool Support: Annotating images with semantic segmentation requires specialized tools capable of handling pixel-wise annotation. Superpixel annotation tools, which divide the image into larger tiles based on edge detection, are often used to simplify the annotation process.
Semantic segmentation is a powerful technique for pixel-wise annotation in computer vision. It enables precise object segmentation and is particularly valuable in applications that demand a granular understanding of an image's content, such as autonomous vehicles and scene analysis. While it requires substantial effort, the level of detail it provides is unmatched in many computer vision tasks.
IV. Landmark Annotation
Landmark annotation is a data annotation technique used in computer vision to identify and mark key points or landmarks on objects, primarily on human faces and bodies, but it can also be applied to other objects. These landmarks serve as reference points that allow for precise tracking, recognition, and analysis of objects and their movements.
Key Points of Landmark Annotation
1. Facial Landmarks: Landmark annotation is prominently used for facial landmark detection. It involves annotating critical points on a human face, such as the eyes, eyebrows, nose, lips, and the oval shape of the face. These landmarks play a crucial role in facial recognition, analysis, and various applications like face morphing and replacement.
2. Object Landmarks: Beyond faces, landmark annotation can also be applied to other objects and body parts. For instance, it is used in human pose estimation, where key points on the human body are annotated to create a 2D or 3D skeleton, enabling accurate assessment of human posture and movement.
3. Applications of Landmark Annotation: Landmark annotation finds applications in various domains, including:
- Facial Gesture Recognition: It helps identify and understand human facial expressions and emotions, which is vital in applications like mood analysis and sentiment recognition.
- Human Pose Estimation: Landmarks are used to create skeletal structures, enabling fitness apps to assess exercise correctness and enhancing safety in industrial settings by detecting incorrect body postures.
- Counting Small Objects: Landmark annotation can be used for counting small objects in bird's-eye-view images, making it valuable in applications like monitoring parking lots or forests from aerial views.
Industries Where Landmark Detection Is Used:
The application of landmark annotation is diverse and spans across several industries, including:
- Sports: Fitness apps use landmark annotation to assess the correctness of users' movements and poses during exercise.
- Gaming: Landmark annotation is used to simulate human-like movements in video games, enhancing the realism of character animation.
- Deepfakes: Landmark annotation is a fundamental component of deepfake technology, allowing for face replacement and realistic morphing.
- Augmented and Virtual Reality: It helps create lifelike characters and creatures for immersive AR and VR experiences.
- Cinema: In the film industry, landmarks enable the creation of lifelike and natural movements for computer-generated creatures and characters.
- Military: Drones equipped with landmark detection can be used for monitoring weapon reserves and observing military activities.
- Safety and Security: It can be applied to monitor crosswalks for self-driving cars and enhance security and surveillance by detecting unusual human activity and changing conditions.
Challenges in Landmark Annotation
While landmark annotation is a valuable tool, it comes with its own set of challenges, including:
1. Limited Training Data: Successful machine learning projects relying on landmark annotation require extensive and diverse training datasets. This may involve capturing images and videos from various angles to enable more accurate recognition.
2. Background and Illumination: Real-world photos and videos often come with challenges related to background and illumination, making it harder to annotate landmarks accurately. Issues like overexposure, shadows, and cluttered backgrounds can affect recognition.
3. Hidden Object Parts: Landmark annotation may become challenging when object parts are hidden, bent, twisted, or turned. Inaccurate or incomplete landmark settings can lead to poor recognition of movements, gestures, and emotions.
Landmark annotation is a versatile technique used to mark key points on objects, primarily human faces and bodies, to enable precise tracking, recognition, and analysis. Its applications span across multiple industries, enhancing tasks such as facial recognition, pose estimation, gaming, and safety. Despite its advantages, challenges related to data volume, background, and occlusion need to be addressed for accurate and reliable results.
V. Line Annotation
Line annotation is a data annotation technique used in computer vision to create and mark lines and splines in images. It serves the purpose of delineating boundaries or highlighting specific features within an image. Line annotation is particularly useful when a region that needs to be annotated can be conceptualized as a boundary, but it is too small or narrow for a bounding box or other types of annotation to be practical.
Key Points of Line Annotation
1. Creating Boundaries: Line annotation is primarily employed to define boundaries or regions within an image. It involves drawing lines or splines along edges or features that need to be delineated. These annotations can help machine learning models understand and differentiate between different parts of an image.
2. Use Cases:
- Warehouse Robotics: Line annotation is used in training robots that operate in warehouse settings, helping them recognize differences between various sections of a conveyor belt or identify pathways for navigation.
- Autonomous Vehicles: Line annotation plays a critical role in autonomous driving systems as it enables the recognition of lanes, lane boundaries, and road markings. This information is vital for autonomous navigation and safety.
3. Precise Recognition: Line annotations contribute to the precise recognition of features within images. They enable computer vision systems to understand the spatial layout and relationships between objects, enhancing their ability to make informed decisions.
Industries Where Line Annotation Is Used
Line annotation finds application in several industries, each benefiting from its capability to define boundaries and recognize critical features:
- Autonomous Vehicles: Line annotation is fundamental for self-driving cars to navigate and stay within lanes, ensuring safe and efficient transportation.
- Manufacturing and Warehousing: In manufacturing and logistics, line annotation assists robots in identifying paths, handling materials, and maintaining efficient operations.
- Agriculture: Agricultural equipment relies on line annotation for tasks like crop monitoring and automated harvesting, helping optimize yields and resource usage.
- Retail and E-commerce: In warehouses and distribution centers, line annotation supports automated systems in managing inventory and ensuring smooth product flow.
- Geospatial Analysis: Line annotation is used in geospatial applications to define boundaries and features on maps, aiding in land use planning and environmental analysis.
Challenges in Line Annotation
While line annotation is a valuable tool in computer vision, it does present some challenges:
1. Annotation Subjectivity: Line annotation can sometimes be subjective, as different annotators may draw lines slightly differently. Achieving consistency in annotation can be a challenge.
2. Complex Shapes: When annotating complex or irregular shapes, it may be challenging to accurately represent the boundaries using simple lines or splines.
3. Varying Image Quality: Images with low resolution or high levels of noise can make line annotation more difficult, potentially leading to less accurate results.
Line annotation is a critical technique in computer vision, facilitating the precise definition of boundaries and features within images. Its applications are diverse, spanning across industries where the recognition of lines, lanes, and pathways is essential for the efficient operation of AI systems.
Despite potential challenges related to subjectivity and image quality, line annotation remains a valuable tool for enhancing the capabilities of machine learning models in various domains.
VI. 3D Annotation
3D annotation is a data annotation technique applied to three-dimensional data, allowing depth, distance, and volume to be considered when labeling and categorizing objects or structures in a 3D space.
While the more common forms of annotation are performed on 2D images, 3D annotation is essential when dealing with volumetric data or when additional dimensions are critical for understanding the content. Here's an overview of 3D annotation and its applications:
3D Annotation in Different Contexts
1. Medical: 3D annotation is commonly used in the medical field for analyzing 3D scans like CT (computed tomography) and MRI (magnetic resonance imaging). It enables the precise labeling and identification of anatomical structures and abnormalities in the three-dimensional space of the body.
2. Geospatial: In geospatial applications, 3D annotation helps in the detection of three-dimensional structures on Synthetic-Aperture Radar (SAR) imagery. This is particularly valuable for tasks like urban planning, terrain analysis, and disaster response.
3. Automotive: The automotive industry relies on 3D annotation for tracking and recognizing vehicles and objects in LiDAR (Light Detection and Ranging) point cloud data. LiDAR technology is instrumental in autonomous vehicles for perceiving their surroundings.
4. Industrial: 3D annotation is used in industrial settings to detect anomalies and quality control issues in 3D scans of products, ensuring that they meet the desired specifications.
5. Agriculture: It plays a role in agriculture by powering harvesting robots for fruit picking. The three-dimensional perception of the environment allows robots to accurately identify and pick ripe fruits.
6. Retail: In the retail sector, 3D annotation is employed for detecting gestures and poses in three-dimensional space. This technology is essential for virtual reality (VR) and augmented reality (AR) applications, enhancing the interaction between users and virtual environments.
The Pros of 3D Annotation
1. Enhanced Dimensionality: 3D annotation considers additional dimensions, providing valuable insights, especially when 2D visual data is insufficient. This is particularly important in challenging conditions like fog, darkness, occlusion, or scenarios where depth and volume perception are critical.
2. Approximation on 2D Data: While 3D annotation is best suited for true 3D data, it can also be approximated on 2D data. For example, sequences of DICOM (Digital Imaging and Communications in Medicine) slices can be used for 3D medical segmentation, and cuboid estimation can be applied to flat images based on perspective.
The Cons of 3D Annotation
1. Time-Consuming: Annotating 3D data is a time-consuming task, and it requires advanced tools and software that can efficiently handle large volumes of three-dimensional data.
2. Quality Limitations: The quality of 3D annotation can be affected by the nature of the data source. LiDAR data, for example, provides high precision for objects close to the source but may suffer from point dispersion as objects move further away, potentially affecting the quality of the annotation.
3D annotation is a valuable technique that considers depth and volume when annotating objects or structures in a three-dimensional space. Its applications span various industries, offering insights and precise recognition capabilities in situations where 2D data falls short. While it has its challenges, it plays a crucial role in fields where three-dimensional understanding is paramount.
VII. Video Annotation
Video annotation is the process of adding labels, tags, shapes, or timestamps to video data to enhance its content and guide user actions. This technique is essential for various applications, particularly in the field of artificial intelligence, where it is used to train computer vision models and improve video content for viewers. Here's an overview of video annotation and its benefits:
Video Annotation in Machine Learning
In the context of artificial intelligence, video annotation plays a crucial role in labeling and categorizing data to teach computer vision models how to recognize specific objects, actions, or situations in videos. For example, a dataset containing videos of cats and dogs can be annotated with labels to train a computer vision model to distinguish between these animals. Video annotation serves as the foundation for supervised learning models, allowing them to recognize patterns and objects.
Role of Video Annotation
Video annotation is used for a variety of purposes, including:
1. Detection: Annotating videos to train AI models to detect objects or specific features in video footage. This is valuable for applications such as detecting cars on the road, identifying road damage, or spotting animals in wildlife videos.
2. Tracking: AI models can track objects in video footage and predict their future positions. Object tracking is essential for tasks like monitoring pedestrian or vehicle movement for security purposes.
3. Location: Training AI to locate and provide coordinates for objects within video footage. This can be applied to tasks such as monitoring parking space occupancy or coordinating air traffic.
4. Segmentation: Creating different classes and training AI models to recognize and categorize different objects or actions in video content. For example, video annotation can be used to identify and count ripe and unripe berries in agricultural footage.
Benefits of Video Annotation
Video annotation offers several advantages over annotating individual images:
1. Interpolation: Video annotation tools allow for the annotation of keyframes at the beginning and end of a video sequence. The tool can then interpolate annotations for the frames in between, saving time and effort.
2. Temporal Context: Videos provide temporal context, allowing AI models to understand object movements and changes over time. This is particularly valuable for tasks that involve motion and action recognition.
3. Better Data: Annotating videos provides AI systems with more data to work with, resulting in more accurate model training and recognition.
4. Cost-Effective: More data points can be obtained from a single video compared to a single image. Focusing on selected keyframes reduces the time required for annotation.
5. Real-World Applications: Annotated videos more accurately represent real-world scenarios and can be used to train more advanced AI models, leading to a wide range of computer vision applications across various industries.
3. Techniques for Image Annotation
Manual image annotation is a fundamental technique in computer vision and machine learning, where human annotators carefully select regions or objects within an image and provide descriptive labels or metadata for those specific parts. This process is essential for training machine learning algorithms, building image recognition models, and enhancing the understanding of visual data. Here's an elaborate exploration of manual image annotation:
I. Manual Annotation Process
The manual image annotation process involves human annotators who are tasked with analyzing raw, unlabeled visual data, such as photographs, videos, or other types of images. These annotators follow specific guidelines, rules, or specialized data annotation methodologies to identify and describe objects, regions, or elements within the images. The key steps in manual annotation are as follows:
- Data Selection: Annotators are provided with a batch of raw image data. This data may come from various sources, including surveillance footage, medical images, satellite imagery, or any domain where visual data analysis is required.
- Data Annotation Guidelines: Annotators receive detailed instructions on how to classify or label the visual content. Guidelines may specify the types of objects or regions of interest to be annotated, the format for providing annotations (text, labels, bounding boxes, polygons, etc.), and any specific rules or criteria for the task.
- Annotation Process: Annotators manually review each image and select specific regions or objects within the image. They then provide written descriptions, labels, or other metadata to describe these regions. The annotations could range from simple object labels to more complex descriptions, such as fine-grained attributes.
Annotation Strategies
Manual image annotation can involve various strategies, depending on the complexity of the task and the level of detail required:
- Bounding Box Annotation: This is one of the simplest and most common manual annotation strategies. An annotator draws rectangles (bounding boxes) around objects or regions of interest within the image and provides labels for these bounded areas. Bounding box annotation is widely used in object detection tasks.
- Polygon Annotation: Similar to bounding boxes, polygon annotations involve outlining objects or regions, but with more detailed shapes. This approach is often used in scenarios where the precise boundaries of objects need to be defined, such as in fine-grained image segmentation tasks.
- Semantic Segmentation: In semantic segmentation, annotators manually classify each pixel in an image, assigning a label to every pixel. This is a more intricate and time-consuming annotation method but is valuable for tasks that require precise delineation of object boundaries and fine-grained image understanding.
Use Cases and Applications
Manual image annotation plays a pivotal role in various applications and industries, including:
- Object Detection: Manual annotation is crucial for training object detection models. Annotators mark objects in images with bounding boxes or other annotations, allowing algorithms to learn to identify and locate objects within images.
- Image Segmentation: For tasks like medical image analysis or autonomous driving, manual annotation is used to segment images into regions of interest. Precise segmentation helps identify and analyze specific areas within images.
- Content Moderation: In social media and content platforms, manual annotation is employed to label or flag content that violates community guidelines, ensuring a safe and appropriate user experience.
- Medical Imaging: Manual annotation is extensively used in the healthcare sector to label specific structures or anomalies in medical images, aiding in the diagnosis and treatment of various conditions.
Quality Control and Verification
Ensuring the accuracy and consistency of manual annotations is critical. Quality control measures may include inter-annotator agreement (multiple annotators reviewing the same data), review by domain experts, and feedback loops to improve annotation guidelines and accuracy over time.
Manual image annotation is a labor-intensive but essential technique for enhancing the understanding of visual data and training machine learning algorithms.
It involves human annotators selecting and describing objects or regions within images, and it is used in various applications to improve image recognition, content moderation, and data analysis in fields ranging from computer vision to healthcare.
The choice of annotation strategies, such as bounding boxes, polygons, or semantic segmentation, depends on the specific requirements of the task at hand.
II. Semi-automatic Annotation
Semi-automatic image annotation is a technique that combines manual annotation by human annotators with automated processes, particularly relevance feedback, to enhance the annotation of digital images. This approach aims to strike a balance between labor-intensive manual annotation and fully automated annotation methods.
In the context described in your provided content, semi-automatic image annotation is used to improve image retrieval systems and the quality of annotations by integrating the annotation process with relevant feedback. Here is an elaborate exploration of semi-automatic image annotation:
The Semi-Automatic Annotation Process
The semi-automatic annotation process combines the efforts of human annotators and machine learning techniques. The key steps involved in this process are as follows:
- Initial Manual Annotation: The process begins with a set of raw, unlabeled images. Human annotators are responsible for manually selecting regions or objects within these images and providing initial annotations, such as keywords or labels. These manual annotations serve as a foundation for the subsequent stages.
- Relevance Feedback: After the user submits a query for image retrieval, the system retrieves images based on the query, and these retrieved images are presented to the user. Users can then provide relevant feedback by indicating which images are relevant and which are not. This feedback helps improve the retrieval results.
- Integration of Relevance Feedback and Annotation: The user's feedback not only enhances the retrieval results but also contributes to the image annotation process. Images that the user marks as relevant receive automatic annotations related to the query keyword. This means that the initial manual annotations provided by human annotators are expanded upon based on user feedback.
- Automatic Keyword Updates: The system assigns an initial weight to each query keyword associated with an image. This weight can be increased when users confirm relevance through feedback or decreased when images are deemed irrelevant. If the keyword's weight drops below a certain threshold, it may be removed from the image's annotation. This results in a dynamic set of keywords and their associated weights for each image.
Use Cases and Applications
Semi-automatic image annotation is especially valuable in applications where a large volume of images needs to be annotated to improve retrieval accuracy, and where user feedback plays a significant role. Some of the applications and use cases include:
- Image Retrieval: Semi-automatic annotation enhances image retrieval systems by allowing users to actively contribute to the annotation process through relevance feedback. This leads to improved retrieval results and a more user-focused experience.
- Personal Image Management: It can be applied in personal image management systems, where users are encouraged to annotate and organize their photo collections based on their preferences and feedback.
- Multimedia Databases: In the context of multimedia databases, semi-automatic annotation supports users in searching and retrieving images and multimedia content with greater accuracy.
- Content Moderation: For online platforms and social media, semi-automatic annotation can be used for content moderation by enabling users to flag or label images for relevance, ensuring that inappropriate content is appropriately managed.
Benefits and Challenges
Semi-automatic image annotation offers several advantages:
- User Involvement: It encourages users to actively participate in the annotation process, enhancing the relevance and quality of annotations.
- Efficiency: It strikes a balance between manual and automatic annotation, allowing for quicker expansion of the annotation database.
- Dynamic Annotation: Keywords and their weights can be updated dynamically based on user feedback, leading to an adaptive annotation system.
However, it also comes with challenges, including the need to make relevant feedback more discoverable and user-friendly, as well as ensure the accuracy of keyword assignments based on feedback.
Semi-automatic image annotation is a practical approach that harnesses the power of both human expertise and machine learning to improve image retrieval and annotation systems. By combining the benefits of manual and automated processes, it offers an efficient and adaptive solution for managing large image databases.
III. Automatic Annotation
Automatic image annotation, also known as automatic image tagging or linguistic indexing, is a computer vision technique that aims to assign metadata, such as captions or keywords, to digital images automatically. This process is crucial for image retrieval systems, which help organize and locate images within a database based on user-defined queries. Here's an elaborate exploration of the automatic image annotation process and its significance:
Multi-Class Image Classification
Automatic image annotation can be viewed as a specialized form of multi-class image classification. However, it is distinct in that the number of possible classes is exceptionally large, often equivalent to the size of the vocabulary used for annotation. For example, a database of images might have a vocabulary of thousands or even millions of keywords or phrases that can be assigned to each image.
Machine Learning Techniques
Automatic image annotation relies on machine learning techniques to automatically generate annotations for images. The process typically involves two main components:
- Image Analysis: In this step, feature vectors are extracted from the images. These feature vectors capture visual information, such as color, texture, shape, and object detection. These features provide a numerical representation of the image content.
- Training Annotation Words: Machine learning models are trained using pairs of image feature vectors and corresponding annotation words or phrases. The model learns to identify correlations between image features and the textual annotations provided during the training process.
Correlation Learning: Early methods of automatic image annotation focused on learning the correlations between image features and training annotations. These methods attempted to establish relationships between visual content and textual descriptions.
Machine Translation Techniques: As the field advanced, machine translation techniques were employed to bridge the gap between the textual vocabulary (words and phrases) and the "visual vocabulary" found in images. This involved mapping visual features to textual annotations, thereby enabling automatic annotation of new images based on the correlations learned during training.
Classification Approaches: More recent approaches to automatic image annotation often rely on classification techniques. Machine learning models, such as deep neural networks, are trained to predict the most relevant keywords or phrases for an image. These models are capable of handling a vast vocabulary of potential annotations.
Relevance Models: Some automatic image annotation systems use relevance models to assess the significance of various annotations to an image. These models consider not only the presence of an annotation but also its relevance and importance to the image's content.
Advantages of Automatic Image Annotation
- Natural Querying: Automatic image annotation allows users to specify queries in a more natural and user-friendly manner. Users can search for images based on the textual descriptions or concepts they have in mind, making it easier to find relevant images.
- Efficiency: Unlike content-based image retrieval (CBIR), where users often need to rely on low-level features like color and texture, automatic image annotation provides a more efficient and human-centric way to search for images. Users can describe what they are looking for in their own words.
- Scalability: Automatic image annotation helps address the challenges of handling large and constantly growing image databases. Manually annotating images is time-consuming and costly, making it impractical for managing extensive image collections.
Automatic image annotation is a powerful computer vision technique that leverages machine learning to assign metadata to digital images automatically. It allows for more user-friendly and efficient image retrieval, making it a valuable tool for managing and searching through vast image databases, especially in the era of big data and rapidly expanding digital content.
IV. Crowdsourcing
Crowdsourcing image annotation is a powerful technique for efficiently and cost-effectively labeling and annotating large volumes of image data. It involves outsourcing image annotation tasks to a distributed and diverse group of remote workers, often referred to as the "crowd." Here's an elaborate overview of the key aspects and benefits of crowdsourcing image annotation:
Cost-Effectiveness
Crowdsourcing image annotation is cost-effective because it leverages a global workforce to annotate images, eliminating the need to hire and maintain an in-house team. The cost savings can be substantial, particularly for businesses with large image datasets. By paying workers on a per-task basis, you can control costs more effectively and avoid the overhead associated with full-time employees. This is especially beneficial for startups and smaller organizations with budget constraints.
Speed
Image annotation is a time-consuming process, and crowdsourcing can significantly expedite the task. With numerous remote workers annotating images simultaneously, projects can be completed in a fraction of the time it would take in-house. This rapid turnaround is critical for various applications, such as real-time object detection, medical image analysis, or content moderation. Crowdsourcing can be particularly valuable when time-sensitive image labeling is required.
Scalability
Crowdsourcing provides unparalleled scalability. Whether you have a few hundred images or millions, you can easily adjust the workforce to meet your project's needs. This flexibility is crucial when dealing with fluctuating workloads or large-scale projects. For instance, Google's Quick Draw dataset, consisting of over 50 million images, demonstrates how crowdsourcing can be used to scale annotation efforts effectively.
Diversity
Crowdsourcing enables access to a diverse pool of annotators from various backgrounds, cultures, and demographics. This diversity is essential when annotating images that should reflect a wide range of perspectives, such as facial recognition datasets. By sourcing annotations from a global crowd, you can ensure that your data is representative of the real-world diversity found in your target application. This enhances the quality and fairness of machine learning models.
Quality Control
While humans can provide high-quality annotations, maintaining consistency and accuracy can be challenging when annotators are overwhelmed with a high volume of images. Crowdsourcing addresses this issue by distributing the workload among a larger number of annotators. This not only speeds up the process but also allows you to maintain quality standards by cross-verifying annotations. Crowdsourcing platforms often incorporate quality control mechanisms, such as redundancy and consensus, to ensure the accuracy of annotations.
Specialized Expertise
Depending on the nature of your image annotation task, crowdsourcing can provide access to specialized expertise. For instance, if you require annotations in a niche field like medical imaging or art classification, you can find workers with domain knowledge who can provide more accurate and relevant annotations. This ensures that the annotations are of the highest quality and align with the specific requirements of your project.
Versatility
Crowdsourcing can be applied to various image annotation tasks, including object detection, image segmentation, text recognition, and more. It can also adapt to different data formats, such as images, videos, or 3D scans, making it a versatile solution for various machine learning and computer vision applications.
Crowdsourcing image annotation offers a cost-effective, efficient, and scalable solution for labeling and annotating large image datasets. It leverages the power of a diverse crowd of remote workers to ensure quality, accuracy, and representation of various perspectives in your annotated data. Whether you're a startup looking to build a machine learning model or a large corporation with extensive image data needs, crowdsourcing is a valuable technique to consider for image annotation tasks.
4. Best Practices for Image Annotation
I. Data Quality and Accuracy
To prioritize data quality and accuracy in image annotation, it's essential to employ expert annotators, establish clear annotation guidelines, and maintain an iterative review process. Feedback and communication with annotators are crucial, and the use of quality assurance tools can help detect and rectify errors.
Small pilot datasets can be used for validation before scaling up, and continuous training keeps annotators updated. Emphasizing quality over quantity is vital, as a smaller dataset with meticulously labeled data points ensures better model performance by preventing anomalies and mislabeled instances from affecting results.
II. Consistency and Standardization
Consistency and standardization play a crucial role in image annotation, ensuring the accuracy of machine learning models. Objects of interest often have varying degrees of sensitivity, demanding uniformity in the annotation process. For example, when labeling a "crack" on a vehicle body part, consistent criteria are essential across all images.
Precise annotation guidelines, constant inter-annotator communication, and annotation tools help maintain this uniformity, minimizing the risk of model confusion due to ambiguous or inconsistent annotations. To maintain consistency and standardization, regular quality checks, standardized nomenclature, and an iterative approach for process improvement are key.
These practices create a reliable and standardized dataset, enabling machine learning models to learn and make accurate predictions based on a consistent understanding of labeled objects and attributes. Inconsistencies in annotation can lead to reduced model accuracy, making it imperative to emphasize and implement these best practices in the annotation process.
III. Data Security and Privacy
In the realm of image annotation, safeguarding data security and privacy is paramount to protecting individuals' rights and ensuring the ethical use of personal information. Data security involves implementing robust measures to protect electronic data from unauthorized access, which is especially crucial when outsourcing data annotation projects.
The ever-increasing adoption of AI and machine learning technologies has made data security and privacy compliance issues more pressing. Training data often contains sensitive personal information, such as names, addresses, and birthdates. Inadequate security measures can result in data breaches, potentially leading to identity theft and malicious use.
When considering outsourcing annotation, it's essential to assess vendors' data security protocols to safeguard your data throughout the process, from ingestion to delivery.
Crowdsourcing is a popular method for obtaining training data quickly and cost-effectively. However, it carries substantial risks, including quality control issues, security vulnerabilities, and potential cost overruns. The lack of control over annotators' qualifications and the absence of confidentiality guarantees pose significant security concerns.
When selecting a data annotation provider, it's vital to choose one with stringent security measures and high-quality standards to protect your data. Moreover, the careful handling of personal information during the annotation process is crucial to maintaining trust.
Companies specialize in data annotation for large training sets, embedding layers of security into their processes, including physical, internal, and cybersecurity measures to ensure data privacy and security compliance. These security considerations are essential to the responsible and ethical use of personal data in the field of image annotation.
IV. Annotator Training and Guidelines
Annotator training and guidelines are fundamental to the success of image annotation projects. Once you have established your annotation schema, it's imperative to invest in training your data annotators on how to use it accurately and efficiently.
Various methods can be employed for this purpose, such as online courses, workshops, quizzes, feedback sessions, and mentorship. Providing a structured training program ensures that annotators understand the annotation guidelines and criteria thoroughly, reducing the risk of errors and inconsistencies.
It's also vital to continuously monitor annotators' progress and performance, offering regular feedback and support to address any issues or uncertainties that may arise during the annotation process. Encouraging open communication among annotators and with project managers is equally important, as it allows for the exchange of insights and the quick resolution of any questions or ambiguities.
Before, during, and after the annotation process, certain principles should guide your approach. Prior to commencing the collection of annotations, it's advisable to set a quality goal for your gold dataset.
This goal should be based on expert benchmarks or past annotation tasks, helping you define the level of quality needed for a successful project outcome. During the annotation process, adherence to established guidelines is crucial to maintain consistency and accuracy.
Regular quality checks and feedback loops should be implemented to identify and rectify any deviations from the guidelines promptly. Post-annotation, it's essential to continuously refine the guidelines based on lessons learned and feedback from annotators to improve the overall quality of the dataset.
Annotator training and guidelines serve as the foundation for the success of image annotation projects, ensuring the reliability and precision of the data generated.
5. Image Annotation Tools and Software
I. Labellerr
Labellerr is your go-to tool for high-quality image and video annotation, boasting advanced automation and smart quality assurance. With the capability to process millions of images and thousands of hours of videos in just a few weeks, Labellerr is a game-changer for AI teams.
Key features include:
- Automated Labeling: Enjoy prompt-based, model-assisted, and active learning-based labeling automation for lightning-fast results.
- Multiple Data Types Support: Whether it's images, videos, PDFs, text, or audio, Labellerr supports a wide range of data types for diverse project needs.
- Smart QA: Our pre-trained model and ground-truth-based quality assurance ensures accurate annotations.
- MLOps Integration: Seamlessly integrate Labellerr with your AI infrastructure, including GCP Vertex AI, AWS SageMaker, and custom environments.
- Project Management and Advanced Analytics: Optimize your projects with prompt-based labeling, model-assisted labeling, and active learning, all supported by Labellerr's advanced analytics.
- 24/7 Support: Get round-the-clock technical support for your critical projects with the fastest response times.
Labellerr's impact is backed by satisfied customers, such as Intuition Robotics, Wadhwani AI, Perceptly Inc., and more. With impressive metrics that matter, including 99% accurate labels and significant reductions in time to data preparation and development costs, Labellerr is a must-have tool for AI teams looking to accelerate their data preparation efforts.
Try a free 7-day pilot with no credit card required and experience the future of image annotation with Labellerr.
II. Labelbox
- Overview: Labelbox is a comprehensive image annotation platform that aims to simplify the process of labeling data for machine learning. It provides a cloud-based solution for annotation, enabling teams to collaborate on labeling tasks.
- Features: Labelbox supports a wide range of annotation types, including bounding boxes, polygons, segmentation masks, and key points. It also offers features for text annotation and classification. Its user-friendly interface makes it accessible to both technical and non-technical users.
- Scalability: Labelbox is designed for scalability and can handle large datasets. It offers automation and data management features to optimize labeling workflows.
- Integration: It provides integrations with popular machine learning frameworks and tools, allowing for seamless data preparation and model training.
- Use Cases: Labelbox is suitable for various use cases, including object detection, image segmentation, autonomous vehicles, medical imaging, and more.
III. Supervised
- Overview: Supervised is an annotation tool that leverages active learning to make the annotation process more efficient. It helps users label the most informative data points, reducing the overall labeling workload and cost.
- Active Learning: The tool uses machine learning models to select data points that are most uncertain, ensuring that annotators focus on the samples that will have the greatest impact on model performance.
- Integration: Supervised can be integrated into your machine learning workflow, ensuring that annotated data is immediately usable for training and evaluation.
- Cost-Efficiency: By reducing the number of annotations required to achieve a certain level of performance, Supervised can be a cost-effective solution for data labeling.
IV. VGG Image Annotator (VIA)
- Overview: VIA is an open-source image annotation tool developed by the Visual Geometry Group at the University of Oxford. It's a lightweight and user-friendly solution for basic annotation tasks.
- Simplicity: VIA is known for its simplicity and ease of use. It is designed for quick image annotation, making it suitable for small-scale projects and research purposes.
- Annotation Types: While it doesn't offer an extensive range of annotation types, VIA supports basic annotations like bounding boxes, polygons, and lines.
- Open Source: Being open source, VIA can be customized and extended to suit specific research needs, making it a valuable resource for the academic and research community.
V. LabelMe
- Overview: LabelMe is an online platform designed for image annotation and labeling. It is particularly popular among researchers and small-scale annotation projects.
- User-Friendly Interface: LabelMe provides a straightforward interface for drawing bounding boxes, polygons, and other annotations directly on images.
- Community Dataset: LabelMe offers an open-access dataset with annotated images, which is widely used in the computer vision research community for benchmarking and training machine learning models.
- Research Focus: LabelMe is commonly used for academic and research purposes, allowing users to contribute to and benefit from a shared dataset.
VI. COCO Annotator
- Overview: COCO Annotator is a specialized tool tailored for annotating data in the COCO format. COCO is a widely used dataset format for object detection and segmentation tasks.
- COCO Format Support: This tool streamlines the process of annotating images and their associated metadata according to the COCO format, making it a suitable choice for projects that require compatibility with the COCO dataset.
- Efficiency: It focuses on efficient annotation workflows specific to the COCO standard, allowing for the quick creation of annotated datasets.
- Use Cases: COCO Annotator is ideal for projects in computer vision that utilize the COCO dataset structure, including object detection, keypoint detection, and segmentation.
VII. Custom In-House Tools
- Overview: Custom in-house tools are annotation software developed internally by organizations to meet specific project requirements.
- Tailored Solutions: These tools are designed to be highly customized, allowing organizations to cater to their unique annotation needs and data formats.
- Control and Flexibility: In-house tools provide complete control over the annotation process, which can be essential for projects with specific data requirements or constraints.
- Development and Maintenance: While powerful, creating and maintaining custom tools can be resource-intensive, which may not be cost-effective for smaller projects. Organizations should weigh the benefits against the development effort.
Each of these tools has its strengths and may be better suited to different use cases and project requirements. The choice of tool ultimately depends on factors such as the complexity of the annotation task, project scale, available resources, and the desired level of customization and control.
6. Challenges and Pitfalls
Image annotation is a crucial step in training machine learning models, particularly in computer vision tasks. However, it comes with several challenges and pitfalls that need to be carefully addressed. Here are some of the key challenges and pitfalls associated with image annotation:
I. Ambiguity and Subjectivity
- Images can be ambiguous, making it challenging to determine the correct annotation. Annotators may interpret images differently, leading to subjectivity in the annotations. For example, the classification of objects in partially occluded or low-quality images may vary among annotators.
- Mitigation: Clear annotation guidelines and constant communication with annotators can help reduce ambiguity and subjectivity. Training annotators to handle specific edge cases and providing them with reference examples can also improve consistency.
II. Scale and Cost
- Annotating a large dataset with thousands or millions of images can be costly and time-consuming. The cost of hiring annotators, acquiring equipment, and managing the annotation process can add up quickly.
- Mitigation: To reduce costs, you can consider using pre-annotated datasets, using crowdsourcing platforms, or leveraging automated annotation tools. Prioritizing data selection and focusing on the most relevant images for your task can also help manage costs.
III. Time-Consuming Nature
- Image annotation can be time-consuming, especially when dealing with complex or detailed annotations like object segmentation. This can slow down the overall development of machine-learning models.
- Mitigation: Streamlining the annotation process with well-defined workflows, using specialized annotation software, or outsourcing to professional annotators can help save time. Automated annotation tools and techniques, such as weak supervision, can also expedite the process.
IV. Quality Assurance
- Ensuring the accuracy and consistency of annotations across a large dataset can be challenging. Annotators may make mistakes or drift in their understanding of the annotation guidelines over time.
- Mitigation: Implementing a robust quality assurance process is essential. This may involve having multiple annotators review and cross-check annotations, conducting periodic meetings and training, and using automated tools to flag potential issues. Regularly monitoring and providing feedback to annotators can help maintain annotation quality.
In addition to these challenges, it's important to consider data privacy and security when sharing and storing annotated images, especially when dealing with sensitive or personal data. Also, staying updated with best practices in image annotation and machine learning techniques can help address evolving challenges in this field.
Overall, addressing the challenges and pitfalls of image annotation requires a combination of careful planning, clear communication, quality control measures, and the use of appropriate tools and techniques to streamline the process.
7. Industry Applications of Image Annotation
I. Autonomous Vehicles
- Object Detection and Recognition: Image annotation is crucial for autonomous vehicles to detect and recognize objects on the road, such as other vehicles, pedestrians, traffic signs, and obstacles. This is essential for making real-time driving decisions.
- Lane and Path Marking: Annotated images help in identifying road lanes and path markings, enabling the vehicle to stay within its lane and navigate safely.
II. Healthcare and Medical Imaging
- Disease Diagnosis: In medical imaging, image annotation is used for annotating various anatomical structures and abnormalities, aiding in the diagnosis of diseases like cancer, heart conditions, and neurological disorders.
- Radiology and Pathology: Radiologists and pathologists rely on annotated medical images for accurate assessments and treatment planning.
- Tracking Progress: Annotated images are valuable for tracking disease progression and the effectiveness of treatments over time.
III. E-commerce and Retail
- Product Recognition: Image annotation is used for recognizing and categorizing products. This is helpful for automated inventory management, product recommendations, and visual product searches.
- Visual Search: Annotated images enable visual search capabilities, allowing customers to search for products using images rather than text queries.
- Quality Control: Image annotation is utilized to identify defects or irregularities in products during the manufacturing or quality control process.
IV. Agriculture
- Crop Monitoring: Image annotation in agriculture involves the labeling of crops, pests, and diseases, facilitating the monitoring and management of crop health.
- Precision Farming: Annotated aerial images aid in precision farming, enabling farmers to optimize the use of resources like water, fertilizer, and pesticides.
- Harvesting and Yield Prediction: Annotated images help in predicting crop yields and optimizing the harvesting process.
V. Geospatial Analysis
- Land Cover Classification: Image annotation is used to classify land cover types, such as forests, urban areas, and water bodies, which is valuable for land-use planning and environmental monitoring.
- Change Detection: Annotated images from different time periods allow for the detection of changes in landscapes, which is critical for urban development, disaster response, and environmental studies.
- Infrastructure Planning: Geospatial annotation aids in the planning and maintenance of infrastructure, including roads, bridges, and utilities.
VI. Security and Surveillance
- Object Detection: Image annotation helps in identifying and tracking objects or individuals of interest in security and surveillance footage.
- Anomaly Detection: Annotated images are used for detecting unusual or suspicious activities in crowded places, critical infrastructure, and public spaces.
- Forensics: In criminal investigations, image annotation supports the analysis of evidence, including fingerprints, facial recognition, and other forensic tasks.
Image annotation plays a pivotal role in these industries, enhancing the capabilities of AI and machine learning systems. Accurate and well-labeled images enable these sectors to make informed decisions, automate processes, improve safety, and enhance overall efficiency.
As the technology evolves, image annotation will continue to be an integral part of various applications across industries, driving advancements in AI and data-driven decision-making.
8. Regulations and Ethical Considerations in Image Annotation
Image annotation plays a crucial role in various fields, including computer vision, machine learning, and artificial intelligence. However, it is essential to ensure that image annotation processes adhere to regulations and ethical considerations to protect individual rights, maintain fairness, and uphold ethical standards. This article focuses on three primary aspects: GDPR and data privacy, bias and fairness, and ethical guidelines.
I. GDPR and Data Privacy
The General Data Protection Regulation (GDPR) is a comprehensive data protection law in the European Union that has significant implications for image annotation. GDPR is designed to protect the personal data of EU citizens, and this includes any images that may contain identifiable individuals. When annotating images, organizations must comply with GDPR by obtaining informed consent from individuals whose images are being used for annotation.
Key considerations related to GDPR and image annotation include:
a. Consent: Image annotation projects must ensure that individuals depicted in images have given their explicit consent for the use of their images for annotation purposes. Consent forms should clearly explain how the data will be used and provide individuals with the option to withdraw their consent at any time.
b. Anonymization: Personal data, such as faces and other identifiable features, should be appropriately anonymized to prevent the identification of individuals in annotated images.
c. Data Security: Organizations should implement robust data security measures to protect annotated images from unauthorized access, breaches, or misuse.
d. Data Retention: Images used for annotation should not be retained longer than necessary, and individuals have the right to request the deletion of their data.
II. Bias and Fairness
Bias in image annotation can lead to unfair and discriminatory outcomes in AI applications. It is crucial to address bias and promote fairness throughout the image annotation process. This includes:
a. Diversity and Representation: Image annotation teams should be diverse and inclusive to ensure a broad perspective during the annotation process. This can help mitigate potential bias.
b. Bias Detection: Implement tools and methodologies to detect and mitigate biases in annotated data. Review and audit annotations to identify and rectify bias-related issues.
c. Guidelines and Training: Provide clear guidelines to annotators about avoiding stereotypes, harmful stereotypes, and favoring fairness. Training annotators on the ethical aspects of image annotation is crucial.
d. Fairness Metrics: Establish fairness metrics and benchmarks to measure and report on potential biases in AI models developed using annotated data.
III. Ethical Guidelines
Ethical considerations in image annotation go beyond legal compliance and fairness. Ethical guidelines help ensure that the annotation process respects human dignity and values. Some important ethical considerations include:
a. Respect for Human Rights: Annotation projects should not compromise individual rights, and images should be annotated in a way that respects privacy and dignity.
b. Ethical Review: Establish an ethical review process for image annotation projects, involving experts who can assess the potential impact on individuals and society.
c. Transparency: Ensure transparency in the annotation process, including disclosing the purpose of annotation and its potential consequences.
d. Accountability: Assign responsibility for ethical oversight and adherence to guidelines within organizations involved in image annotation.
Image annotation is a critical step in developing AI applications, but it must be conducted with careful attention to regulations and ethical considerations. GDPR compliance, addressing bias and fairness, and adhering to ethical guidelines are essential to ensure the responsible and ethical use of annotated image data.
9. Future Trends in Image Annotation
I. Integration of AI in Annotation
As the field of image annotation advances, the integration of AI technologies is poised to play a pivotal role in shaping the future. Here's a detailed look at this trend:
- Automated Annotation: AI-powered annotation tools are becoming increasingly sophisticated, capable of automating the labeling process to a significant extent. This includes techniques like image segmentation, where AI algorithms can identify objects or regions of interest in images. As AI models improve, they can provide preliminary annotations that human annotators can review and refine, greatly reducing the manual effort required.
- Active Learning: AI is being used to improve the efficiency of annotation by selecting the most informative samples for human annotation. Active learning algorithms can identify data points that are more challenging or uncertain for the model, thereby prioritizing the labeling of these samples. This reduces the overall annotation workload and can lead to more accurate models with fewer labeled examples.
- Transfer Learning: AI models trained on large and diverse datasets can be leveraged for image annotation tasks. Pre-trained models, such as those for object detection, segmentation, and facial recognition, can be fine-tuned for specific annotation needs. This transfer learning approach accelerates the annotation process by capitalizing on existing knowledge within the models.
- Quality Assurance: AI is being integrated into the annotation pipeline to ensure the quality of annotations. Algorithms can flag and review potentially inaccurate or inconsistent annotations, leading to improved data quality and reducing the need for manual error correction.
- Semantic Annotation: AI is helping in adding more meaning to annotations. For example, in addition to labeling an object as "car," AI can provide additional attributes, such as color, make, and model. This richer semantic annotation enables more nuanced and context-aware machine learning models.
II. Real-Time Annotation
Real-time annotation is another emerging trend in image annotation, offering several advantages:
- Live Data Labeling: Real-time annotation involves annotating data as it is being generated or captured. This is particularly relevant in applications like autonomous vehicles, where camera feeds are continuously generated. Real-time annotation allows for immediate feedback and model adaptation based on the most recent data.
- Remote Collaboration: Real-time annotation tools facilitate remote collaboration among annotators and experts. Geographically dispersed teams can collectively label data simultaneously, reducing annotation times and enabling real-time decision-making.
- Training Data Refresh: In applications like object detection, models require consistent and up-to-date training data to adapt to changing conditions. Real-time annotation ensures that models are trained on the most current and relevant data, which is critical for tasks like surveillance and security.
- Quality Control: Real-time annotation enables immediate quality control. Annotators can review and verify annotations as they are made, addressing any issues or uncertainties on the spot, which contributes to higher-quality training data.
- Emergency Response: In scenarios where real-time decisions are critical, such as emergency response or disaster management, real-time annotation can provide vital information for AI systems to aid in decision-making and response coordination.
The adoption of real-time annotation are at the forefront of image annotation trends. Real-time annotation is becoming increasingly relevant in applications that require instant decision-making and adaptability based on the most current data.
III. Annotating 3D Data
Understanding 3D Annotation
In the rapidly evolving landscape of image annotation, one of the most promising future trends is the annotation of 3D data. While traditional 2D annotations have been fundamental for various applications, annotating 3D data opens up new dimensions, literally and figuratively. This article explores the concept of annotating 3D data and its potential impact on diverse industries.
3D annotation involves the process of adding annotations and labels to three-dimensional data. It's particularly relevant when dealing with point clouds, which represent objects or scenes in three dimensions. This approach takes into account depth, distance, and volume, providing a richer source of information.
3D annotation is often performed using cuboids, but it can also involve the use of voxels, which are 3D pixels used for semantic segmentation.
Applications of 3D Annotation
The applications of 3D annotation are broad and diverse. It's especially valuable in scenarios where 2D visual data falls short. Here are some key industries and applications where 3D annotation can make a significant difference:
1. Medical Imaging
- 3D annotation is essential for analyzing medical scans like CTs and MRIs. It enables precise identification and segmentation of anatomical structures, tumors, and abnormalities in a three-dimensional space.
2. Geospatial Analysis
- In geospatial applications, 3D annotation helps in detecting and understanding three-dimensional structures, such as buildings or topographical features, from data sources like synthetic-aperture radar imagery.
3. Automotive and Autonomous Driving
- The automotive industry relies on LiDAR point cloud data for autonomous vehicles. 3D annotation plays a crucial role in tracking objects, pedestrians, and other vehicles in a three-dimensional environment.
4. Industrial Inspection
- Quality control and anomaly detection in industrial settings benefit from 3D scans of products. These scans allow for the identification of defects, measurements, and structural analysis.
5. Agriculture
- 3D annotation can empower harvesting robots by enabling them to accurately identify and pick fruits based on their three-dimensional characteristics.
6. Retail and Augmented Reality (AR)
- Retail applications leverage 3D annotation for detecting user gestures and poses, enhancing virtual and augmented reality experiences.
Pros and Cons of 3D Annotation
As with any technology, 3D annotation comes with its set of advantages and challenges:
Pros:
1. More Informative
- 3D annotation takes into account additional dimensions, offering richer and more useful information. This is particularly valuable in adverse conditions like fog, darkness, and occlusion, where 2D data might be limited.
2. 2D Approximation
- It's possible to perform 3D annotations with reasonable approximations on 2D data. For instance, segmentation of sequences of DICOM slices or estimating cuboids in flat images based on perspective.
Cons:
1. Complex and Time-Consuming
- 3D annotation can be a time-consuming process, requiring advanced tools capable of handling large datasets.
2. Data Quality
- The quality of 3D annotations can be affected by the dispersion of LiDAR data points as objects move further away from the source.
Tips for Effective 3D Annotation
To maximize the effectiveness of 3D annotation, consider the following tips:
1. Visualization Tools
- Use tools that allow you to visualize multiple viewpoints (side, top, front) to simplify annotating a single object. Comparing 3D data to a 2D capture of the same scene can serve as a valuable sanity check.
2. Medical Imaging Advantages
- Take advantage of smart segmentation based on tissue density for precise annotations in medical imaging.
3. Consistent Orientation
- Maintain a consistent position along the pitch axis when creating 3D annotations, which eases the labeling of the roll and yaw axes.
The future of image annotation is increasingly tied to the ability to annotate three-dimensional data. As technology and tools for 3D annotation advance, industries such as healthcare, geospatial analysis, automotive, and more will experience significant improvements in data quality and insights.
As the world becomes more reliant on three-dimensional data sources, mastering 3D annotation will be crucial for enhancing the capabilities of machine learning models and computer vision applications.
III. Enhanced Data Augmentation
The Importance of Data Augmentation
Data augmentation has been a standard practice in the deep learning community for years. It involves applying various transformations to the existing dataset to generate new examples. These transformations may include rotations, flips, zooms, and color adjustments. The primary goal of data augmentation is to improve model generalization by exposing it to a more extensive range of variations in the data.
Challenges with Traditional Data Augmentation
While traditional data augmentation techniques have proven effective, they have limitations. They often rely on basic geometric and pixel-level manipulations. This approach may not capture the full spectrum of real-world variations that images can exhibit. In many cases, the diversity introduced by traditional augmentation may be insufficient for training highly robust models.
Enhanced Data Augmentation Techniques
The future of image annotation is shifting towards enhanced data augmentation techniques that provide a more comprehensive and nuanced understanding of the data. These techniques are designed to replicate complex real-world scenarios and challenges. Some of the exciting trends in enhanced data augmentation include:
- Generative Adversarial Networks (GANs)
GANs are being used to generate synthetic data that is indistinguishable from real data. This approach can significantly increase the diversity of the training dataset. For image annotation, GAN-generated images can provide valuable annotations that might be challenging to collect manually.
- Simulated Environments
Simulated environments, such as video games or 3D modeling, are increasingly used for data augmentation. These environments allow the creation of highly realistic scenes and objects. Models trained on data from simulated environments can exhibit better generalization when applied to the real world.
- Domain-specific Augmentations
Enhanced data augmentation techniques are tailored to specific domains. For example, medical imaging may benefit from anatomically accurate deformations, while autonomous driving datasets may include simulations of adverse weather conditions and challenging road scenarios.
Challenges and Considerations
While enhanced data augmentation holds great promise, it comes with its challenges:
(i) Computational Resources
GANs and simulations can be computationally intensive. Training and generating synthetic data may require significant resources.
(ii) Data Privacy
GANs raise concerns about privacy, as they can generate highly realistic faces, objects, or scenes. Striking the right balance between realism and privacy is crucial.
(iii) Validation and Evaluation
Assessing the quality and realism of augmented data is an ongoing challenge. Metrics and evaluation techniques for synthetic data are still evolving.
Enhanced data augmentation is at the forefront of future trends in image annotation. As the field of machine learning continues to advance, the ability to generate diverse, realistic, and domain-specific data will be a game-changer for training deep learning models.
The integration of GANs, simulated environments, and domain-specific augmentations promises to elevate the accuracy and robustness of image annotation, making it a key enabler for numerous applications in computer vision and beyond.
10. Case Studies
I. Enhancing Autonomous Vehicle Safety with Image Annotation
Client: Airbus Autonomous Vehicles
Overview
Airbus Autonomous Vehicles, a leading company in self-driving car technology, aimed to improve the safety and reliability of their autonomous vehicles. They needed to annotate vast amounts of real-world driving scene images to train their vehicle's computer vision systems.
Challenge
To ensure the safe operation of autonomous vehicles, Airbus needed high-quality image annotations, including the identification of various objects on the road, such as pedestrians, vehicles, road signs, and lane markings. This required precise and consistent annotations to enhance the vehicle's decision-making algorithms.
Solution
Airbus partnered with an image annotation service provider, AnnoTech, to accomplish this task. AnnoTech deployed a team of skilled annotators who received comprehensive training on annotating images to industry standards.
They used advanced annotation tools that allowed them to label objects with accuracy, ensuring that each object's boundaries were clearly defined and properly categorized.
Result
With the help of AnnoTech's image annotation services, Airbus successfully annotated thousands of images to train their autonomous vehicles. The annotated data significantly improved the vehicles' ability to recognize and respond to objects and scenarios on the road.
This led to increased safety, better decision-making, and enhanced autonomous driving capabilities. The accuracy of object recognition and classification improved from 75% to 95%, reducing the risk of accidents and improving the overall performance of their autonomous vehicles.
The success of this image annotation project demonstrated the critical role of high-quality data in the development of self-driving technology, making autonomous vehicles safer and more reliable for the general public.
II. Enhancing Recycling Efforts with Image Segmentation
Client: Waste Management & Recycling Company in Belgium
Overview
A waste management and recycling company in Belgium aimed to improve its recycling efforts and ensure the proper disposal of waste materials by implementing an AI solution that could identify and classify the type of waste in the containers of businesses.
They required image annotation services for their computer vision system to recognize various waste categories, including paper, plastic, wood, garbage bags, glass, food, and dangerous waste.
Challenges
The client faced the challenge of accurately annotating images of waste materials using polygon annotations to categorize and define different types of trash. Image segmentation requires a high level of precision and attention to detail to train the AI system effectively.
Solution
The client chose to collaborate with Mindy Support, an experienced data annotation service provider, based on recommendations from a long-standing partner and a previous successful project.
Mindy Support assembled a team of skilled data annotators who were already proficient in data labeling projects. They meticulously labeled the waste materials in the images, achieving a remarkable quality score of 98% during the annotation phase, surpassing the client's expectations by 3%.
Results
Through the partnership with Mindy Support, the client not only achieved a quality score of 98% but also maintained a successful collaboration for over two years. The accurate image annotations enabled the implementation of their AI solution, which contributed to tracking and improving recycling efforts, ensuring the proper sorting of waste, and enhancing the overall quality of recycled materials.
As a result, the client was highly satisfied with the project's outcomes and considered expanding the taxonomy to include more waste categories, aligning with the evolving needs of recycling technology.
This case study illustrates how image annotation services can play a crucial role in waste management and recycling, enhancing efficiency, safety, and sustainability in the industry.
III. Enhancing Construction Progress Monitoring with Image Annotation
Client: Construction Progress Monitoring Product Developer
Overview
A construction progress monitoring product developer created a system designed to monitor and evaluate the advancement of construction projects over time. To enhance the accuracy of their product, they required annotated image datasets featuring construction sites at different points in time. These annotated datasets were essential for training their machine learning algorithms to compare images and assess the progress made on construction sites.
Challenge
The client faced the challenge of annotating a substantial volume of image data, including images of construction sites from multiple years. Both current images and historical images were necessary to enable the system to compare and measure progress accurately. The annotation task involved identifying and labeling various elements within the images, such as construction structures, parking areas, paths, roads, stadiums, and different types of surfaces (paved and unpaved).
Solution
The client formed a dedicated team of 10 full-time data annotators for the project. The client specifically requested the use of QGIS, a free and open-source geospatial data annotation tool, to perform the image annotation tasks. The output format required was GeoJSON, commonly used for encoding geographic data structures. The team of annotators meticulously labeled and annotated the various elements within the images, ensuring that the construction progress monitoring system could accurately compare and evaluate changes in construction sites over time. Approximately 1,000 total hours were devoted to annotating the required volume of data within the specified project timeframe.
Result
By collaborating, the client successfully obtained accurately annotated image datasets. These datasets empowered their construction progress monitoring product to conduct precise comparisons between images of construction sites from different time periods.
The annotated data significantly improved the system's capability to measure and assess progress on construction sites accurately. This case study underscores the critical role of image annotation in the construction progress monitoring industry, enabling more precise and efficient tracking and evaluation of construction site developments over time, ultimately enhancing the success of construction projects.
Conclusion
In the realm of artificial intelligence and machine learning, image annotation plays a central and indispensable role. We have explored several image annotation tools and software that cater to a diverse array of projects and requirements. These tools, such as Labelbox, Supervised, VIA, LabelMe, COCO Annotator, and custom in-house solutions, offer a spectrum of features and functionalities to aid in the process of data labeling.
However, the importance of image annotation goes far beyond the tools themselves, extending to its role in shaping the future of AI and the ethical and accurate handling of data.
The central role of image annotation in AI
Image annotation is the backbone of AI, enabling machines to understand and interpret the visual world. Through methods such as object detection, image segmentation, and keypoint annotation, image data is transformed into labeled information, allowing machine learning models to recognize patterns, make predictions, and perform tasks with human-like comprehension.
Whether it's autonomous vehicles recognizing road signs, medical AI systems diagnosing diseases from medical images, or any application where visual data is involved, accurate annotation is essential for training reliable and effective AI models.
The future of image annotation
The future of image annotation is marked by ongoing advancements in automation and efficiency. Tools like Supervised, with their active learning capabilities, aim to reduce the annotation workload and cost while maintaining high-quality labeled data. Integrations with machine learning frameworks and AI pipelines are becoming more seamless, making it easier to put annotated data to practical use. In addition, the development of new annotation techniques for emerging technologies, such as augmented reality and autonomous robotics, is on the horizon. As AI continues to evolve, so too will image annotation methods to support its growth.
Importance of ethical and accurate annotation
Ethical and accurate image annotation is of paramount importance. As AI models increasingly influence decision-making processes in various domains, the data used to train these models must be free from bias and errors.
Ethical considerations encompass issues like fairness, transparency, and privacy in data labeling. For example, ensuring that AI systems do not perpetuate societal biases or violate privacy rights is a critical aspect of annotation ethics.
Moreover, annotation accuracy is crucial to the success of AI applications. Annotators must adhere to high standards to create ground truth data that allows AI models to operate with precision and reliability. Errors in annotation can lead to incorrect model predictions, impacting real-world applications such as healthcare, autonomous transportation, and national security.
In conclusion, image annotation is not just a technical step in the AI pipeline; it's a fundamental driver of AI's capabilities and ethical responsibilities. As image annotation tools continue to evolve and address the diverse needs of the AI community, the future promises more efficient workflows and new possibilities for AI applications.
It is imperative that we approach image annotation with a commitment to both accuracy and ethical integrity to ensure that the AI systems we develop are fair, reliable, and beneficial to society as a whole.
Frequently Asked Questions
1. Which data annotation tools should you use?
When it comes to data annotation, you have the flexibility to choose from commercial, open-source, or free annotation tools. These tools are designed to cater to different needs and come with a range of feature sets, providing diverse capabilities. They enable your workforce to efficiently annotate various data types, including streams, frames, and images, whether they are single-frame images, multi-frame sequences, or even videos.
This versatility ensures that you can select the most suitable tool for your specific annotation requirements, allowing you to streamline the annotation process and enhance the quality of your labeled data.
2. Why is image annotation important in computer vision?
Image annotation establishes the benchmarks that neural models strive to replicate, meaning any inaccuracies in the labels can be perpetuated. As a result, the accuracy of image annotation serves as the cornerstone for training neural networks, making annotation a critical and fundamental task in the field of computer vision.
3. How can a medical image be annotated?
Medical images can be enhanced for the detection of abnormalities by adding labels that outline the boundaries of cells or structures within the image. To perform this task, data annotation tools have become increasingly popular and essential, as they enable the application of these annotations to your medical image data.
These tools facilitate the process of marking and highlighting specific regions of interest in the images, which is crucial for various medical applications such as disease diagnosis, treatment planning, and research. By using these annotation tools, healthcare professionals and researchers can more accurately analyze and interpret medical images, ultimately improving patient care and advancing medical knowledge.
Book our demo with one of our product specialist
Book a Demo