How Labellerr Accelerates Sports Image Annotation
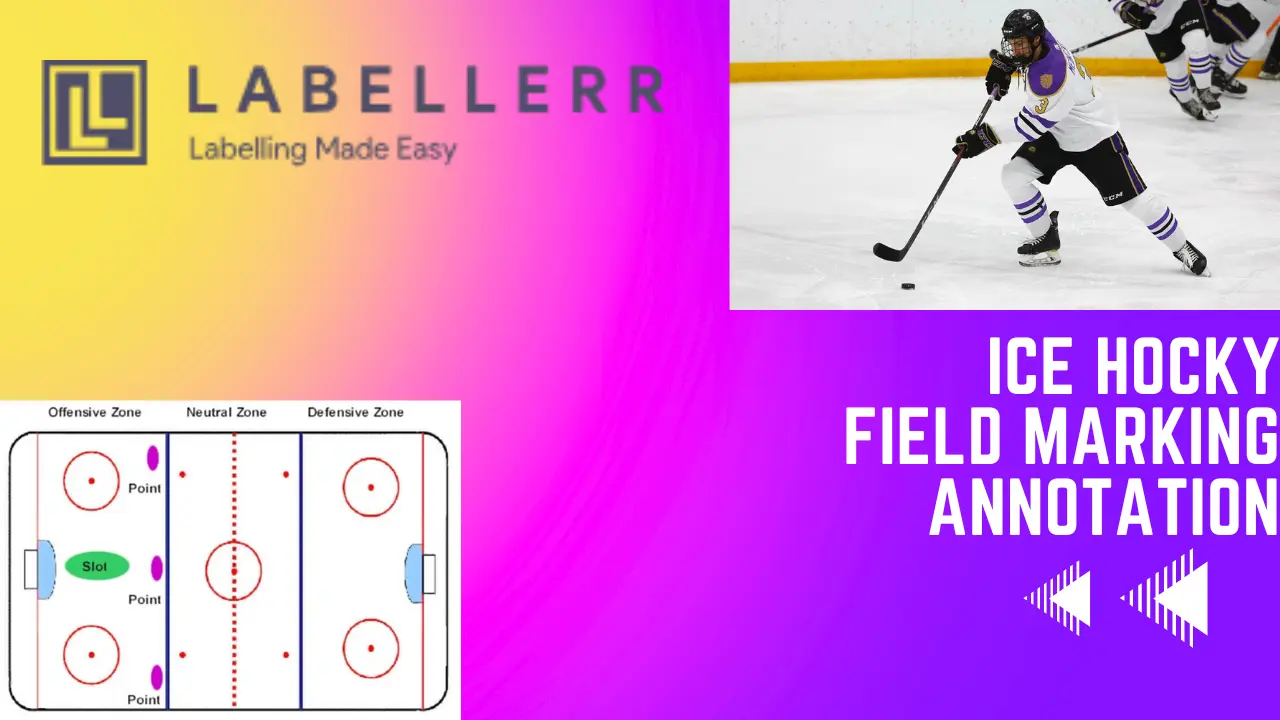
Table of Contents
- Introduction
- How Labellerr Helped Provide Rapid, High-Quality Annotations?
- Advanced Features of Labellerr Platform
- Conclusion
- Frequently Asked Questions
Introduction
Sports image annotation is very high in demand computer vision use case because of its diverse use cases in game analytics, player training and improving viewer experience of the game.
One of the sports for which Labellerr has been used is Ice Hockey.
Ice hockey is a high-speed, precision-driven sport where accuracy and clarity in field markings are crucial.
Whether it's for game officials, players, or viewers, clear outlining of various zones on the rink can impact game play and decisions.
However, identifying and annotating these critical field markings for machine learning applications can be a challenging task due to the fast-paced nature of the sport, variable lighting conditions, and the often complex designs on the ice.
This creates a unique challenge for data annotation platforms, where precision and accuracy are key.
At Labellerr, we specialize in creating robust data annotation solutions for a variety of use cases, and our recent project with ice hockey field marking detection exemplifies this.
Our team was tasked with accurately annotating various rink features, including the center circle, defending zones, attacking zones, and other key elements.
This annotation process served as the foundation for our client's machine learning models, enabling them to enhance their automated systems for game play analysis, player tracking, and officiating support.
Through innovative techniques and a commitment to precision, we successfully delivered high-quality annotated data that met the demands of our client and the fast-paced world of ice hockey.
How Labellerr Helped Provide Rapid, High-Quality Annotations?
Labellerr's platform is designed to deliver high-quality annotations with high speed and accuracy, making it the perfect solution for the complex task of annotating thousands of ice hockey rinks.
Our client needed precise segmentation of key rink elements like center circles, defending zones, attacking zones, and more, as shown in the above image.
Traditional annotation methods would have been time-consuming and prone to errors, but Labellerr's advanced features allowed us to complete the task within weeks without compromising quality.
Through our custom workflows, we streamlined the annotation process, ensuring that each element of the ice hockey rink was accurately labeled.
The robust segmentation features enabled our team to work efficiently, focusing on fine details while maintaining consistency across the entire dataset.
This attention to detail was critical in achieving the high level of accuracy required for our client's machine learning applications.
Furthermore, Labellerr's active learning-based labeling continuously improved the accuracy of our annotations, allowing our platform to adapt and refine its approach as more data was processed.
By leveraging the collaborative annotation pipeline, our team could work in parallel, ensuring that quality control was maintained while accelerating the overall annotation process.
This approach, combined with automated import and export of data, meant that the client could receive high-quality annotations quickly, reducing project turnaround times and costs.
With Labellerr, our client was able to advance their machine learning models for ice hockey analysis, providing them with the precision and accuracy they needed to succeed.
Advance Features of Labellerr Platform
Labellerr provides a comprehensive solution to the challenges of annotating ice hockey field markings, offering tools and features designed to streamline the process and improve accuracy.
Intuitive Interface
Labellerr's user-friendly interface simplifies the annotation process, allowing users to quickly mark and categorize different areas of the ice hockey rink.
This intuitive design reduces the learning curve for annotators, enabling them to focus on precise labeling without being hindered by complex software.
Cost Savings
By automating many aspects of the annotation process, Labellerr reduces the time and resources required to create high-quality training datasets.
This cost-effective approach allows organizations to allocate resources more efficiently, ensuring that they can meet tight deadlines without compromising on quality.
Robust Segmentation Features
Labellerr's robust segmentation capabilities, including the Segment Anything Model (SAM), provide the accuracy and precision needed to detect and annotate various rink markings.
This feature is essential for differentiating between complex elements like the center circle, blue lines, red lines, and other critical areas.
Custom Workflows
Labellerr's custom workflows allow users to create tailored processes for their specific annotation needs.
In the context of ice hockey field marking detection, these workflows can be designed to handle the unique challenges of annotating dynamic and reflective surfaces.
This flexibility ensures consistent and accurate labeling across large datasets.
Active Learning-Based Labeling
Labellerr incorporates active learning into its annotation workflow, enabling the platform to focus on uncertain or ambiguous areas within the dataset.
This approach is particularly useful when annotating ice hockey field markings, where dynamic conditions and player movement can make consistent labeling challenging.
By directing annotators' attention to the most critical parts of the data, active learning helps optimize accuracy and efficiency.
Automated Import and Export of Data
With automated import and export features, Labellerr simplifies the integration of various data sources, whether they are images or videos from different ice hockey arenas.
This automation streamlines the workflow by allowing annotators to import large datasets with ease and export the annotated data for further analysis or model training without manual intervention.
The flexibility to work with multiple formats and sources is especially valuable in the sports industry, where data can come from various cameras and systems.
Collaborative Annotation Pipeline
Labellerr's collaborative annotation pipeline supports teamwork, allowing multiple users to work on the same project simultaneously.
This feature is invaluable when dealing with large-scale ice hockey annotation projects, where consistency and efficiency are paramount.
Annotators can communicate and coordinate their work in real-time, ensuring uniformity across the dataset.
The collaborative pipeline also helps maintain quality and reduces the risk of errors during the annotation process.
Conclusion
In conclusion, Labellerr offers a robust and efficient solution for annotating ice hockey field markings, addressing the unique challenges posed by reflective ice surfaces, dynamic player movement, and varying lighting conditions.
With an intuitive interface, robust segmentation features, and custom workflows, Labellerr streamlines the annotation process, reducing costs and improving accuracy.
By using Labellerr for annotating ice hockey rink markings, organizations can achieve precise and reliable results, leading to more accurate computer vision applications in the sports industry.
Whether it's for coaching, broadcasting, or game strategy, Labellerr provides the tools necessary to make data annotation a seamless and cost-effective process.
Frequently Asked Questions
Q1) What is the purpose of annotating ice hockey field markings?
Annotating ice hockey field markings is essential for creating accurate training datasets for computer vision models.
These models can be used for game analysis, strategic planning, broadcasting, and even rule enforcement during matches. Properly annotated field markings help ensure fair play and provide crucial visual references for various stakeholders.
Q2) How does Labellerr help in annotating ice hockey rink markings with high accuracy?
Labellerr offers a combination of intuitive interface, robust segmentation features, and custom workflows, which streamline the annotation process.
Additionally, advanced features like active learning-based labeling, automated import and export, and collaborative annotation pipeline enhance productivity and accuracy.

Simplify Your Data Annotation Workflow With Proven Strategies
.png)
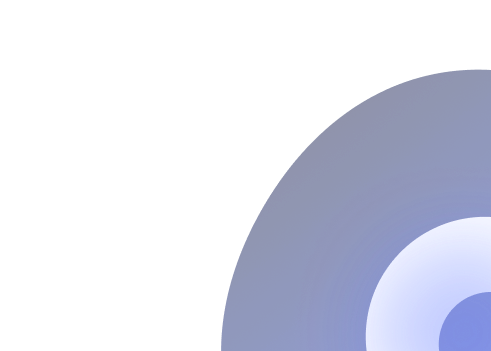