How AI and Machine learning is powering self-driving cars?
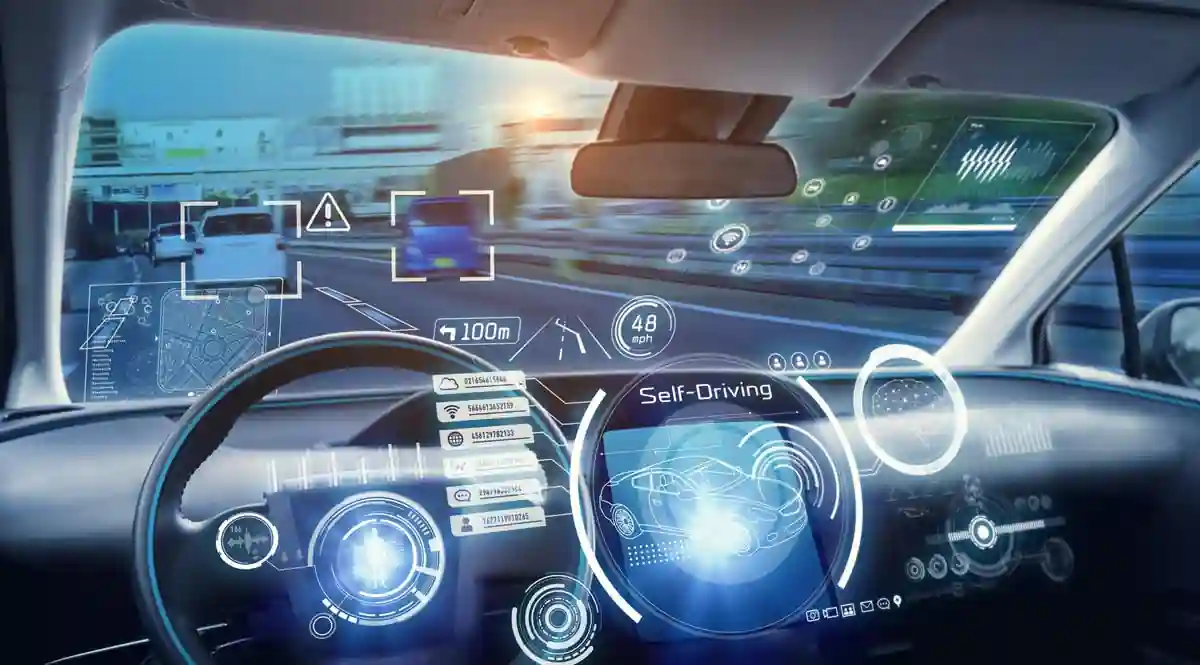
One of the visible and most well-liked trends in machine learning and AI is the creation of self-driving cars. The employment of AI in the automobile industry is now a contentious topic, despite the fact that it is being adopted quickly in a number of industries. Future transportation will be dominated by autonomous cars, which are being created and operated in a variety of methods that incorporate AI and machine learning. In this blog, we have discussed how self-driving cars are powered by AI and Machine learning.
What are self-driving cars/autonomous vehicles?
A vehicle that can sense its surroundings and function without human intervention is an autonomous vehicle. There is no requirement for a human passenger to operate the car at any moment or even to be inside the vehicle in any way. An autonomous vehicle can travel anywhere a traditional vehicle can go and can perform any task that a skilled human driver can.
There are now 6 levels of driving automation, ranging from Level 0 (completely manual) to Level 5, according to the Society of Automotive Engineers (SAE) (fully autonomous). The US Department of Transportation has approved these levels.
How Machine Learning Can Be Used in Autonomous Vehicles
There are many machine learning factors that are being considered in autonomous vehicles
Driving analysis
In order to track the operator and their behavior in self-driving cars, neural networks can identify trends. For instance, facial recognition technology can be used to identify a driver and determine whether they are permitted to operate the vehicle, helping to prevent theft and unauthorized use.
Algorithms for occupancy detection can also be used by the autonomous vehicle system to enhance the ride for other passengers. According to the quantity and location of passengers, the air conditioner may automatically adjust.
Automobile Power trains
Power trains for vehicles often produce a temporal collection of data points. This data can be subjected to machine learning to enhance battery management and motor control.
With Machine Learning, a vehicle is not constrained to boundary constraints that are pre-configured and fixed in place. Instead, the system may adjust over time to the vehicle's deterioration and react to modifications as they occur. With AI and machine learning boundary conditions can be changed as the vehicle ages, its power train evolves, and it is eventually broken in. The vehicle is capable of performing more effectively with flexible boundary conditions.
Safety in Self-Driving Cars
The safety of self-driving cars' propulsion and their ability to avoid causing traffic accidents are without a doubt the most crucial factors to take into account with autonomous cars. This entails guaranteeing the innate protection of the network and technologies that power it, in addition to the operative safety of the vehicle's system and its gadgets.
Security
Automotive cybersecurity is more clearly emphasized as more automobiles use computer systems as well as networking capabilities. However, ML can be applied here to improve security. It can be used, for example, to identify assaults and abnormalities and subsequently deal with them.
A particular car is at risk from a hostile attacker accessing its system or using its data. In order to keep the car, its occupants, and the roadways safe, ML models must be able to recognize various types of threats and anomalies.
Driving support
Driver-less cars can be taught to recognize the specific shape of numerous objects using machine learning. They are able to discriminate between, among other things, automobiles, bicycles, lampposts, and animals.
The assessment of an object's closeness as well as its speed as well as the direction of motion is done through visualization. For better maneuvering, an autonomous car may utilize ML, for instance, to determine the spacing around it before changing lanes.
High-level driving assistance systems, such as those that can perceive and comprehend the environment around a car, also use machine learning. For object recognition and classification, this primarily uses camera-based systems, although there have also been advancements in LiDAR as well as radar technologies, which will be covered in a later section of the article.
How automotive Artificial Intelligence algorithms are used for self-driving cars
The development of self-driving cars presents a number of obstacles that are solved in large part by machine learning techniques. Enhancing the use of machine learning to complete new jobs is crucial with the integration of sensor processing data in an ECU (Electronic Control Unit) in an automobile. Potential uses include the classification of driving scenarios or assessment of driver conditions using data fusion from various internal and external sensors, such as lidar, radar, cameras, or the Internet of Things (IoT). There are many machine learning algorithms that are being utilized in the automation of vehicles.
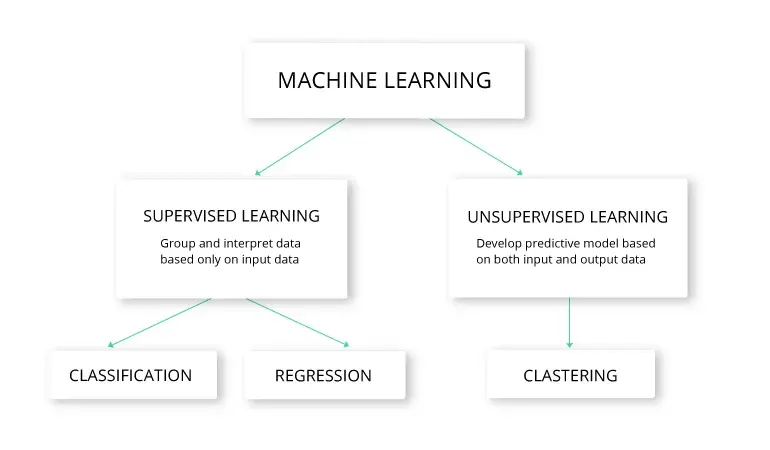
The supervised algorithms utilize a training datasets to learn, and they keep learning until they reach the desired degree of confidence (the minimization of the probability of error). Regression, classification, anomaly detection, and dimension reduction are subcategories of supervised algorithms.
The algorithms that are unsupervised attempt to extract value from the supplied data. This indicates that an algorithm builds a relation within the supplied data to find patterns or separates the set of data into different groups based on how similar they are to one another. A significant portion of the unsupervised algorithms can be further divided into association rule training and clustering.
Another group of machine-learning algorithms that fall between supervised and unsupervised learning is the reinforcement learning algorithms. In supervised learning, there is a goal label for each training example; in unsupervised learning, there are not many labels; and in reinforcement learning, the time-delayed and fragmentary labels represent the future rewards.
The importance of Data Annotation in Automotive AI Projects
All of the basic training data is now prepared using different annotating techniques to help the AI system comprehend what it must be learning. The most popular data annotation techniques for the automotive industry are 3D Point Cloud labeling, video labeling, full scene segmentation, and many others.
The precision and capability of the vehicles to traverse their surroundings will ultimately depend on the level of the data annotation, and let's not forget that lives are on the line. Since human error accounts for 94% of serious crashes, better safety is one of the main objectives of self-driving automobiles. The objective is to make the vehicle as precise and secure as possible while minimizing the human role in driving.
If you are looking for data annotation for your autonomous vehicle project, then reach out to Labellerr.
Labellerr is a computer vision data annotation workflow automation tool that helps data science teams to simplify the manual mechanisms involved in the AI and machine learning product lifecycle. We are highly skilled at providing training data for a variety of use cases with various domain authorities.
To know more such interesting information, stay with us!
Book our demo with one of our product specialist
Book a Demo