6 Encord Alternatives in 2025
In 2025, alternatives to Encord for data labeling include Labellerr, Appen, V7 Labs, Hive, Dataloop, and SuperAnnotate. These platforms offer robust solutions for AI teams with features like automated labeling, quality assurance, and fast deployment.
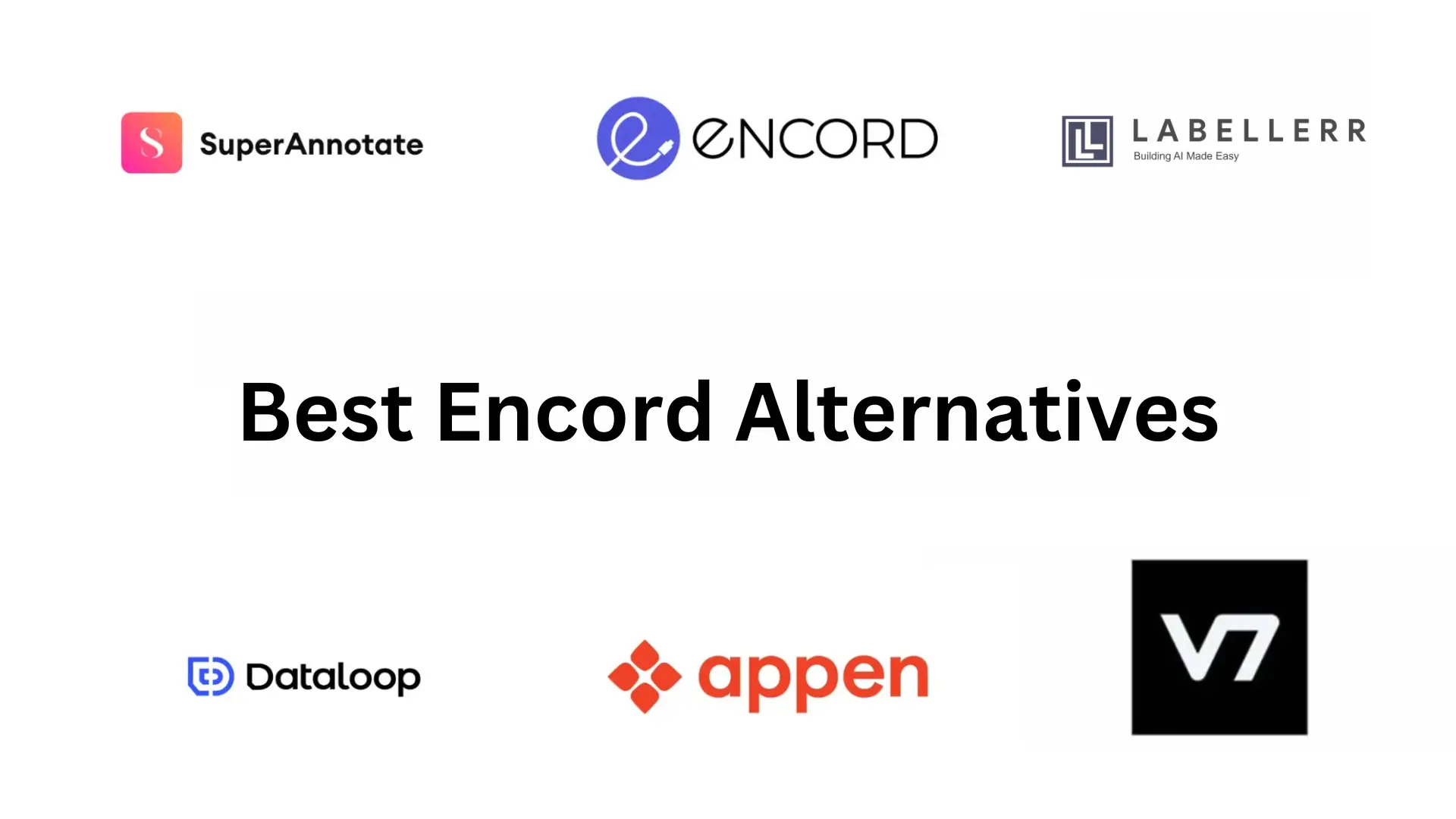
The rapid evolution of artificial intelligence necessitates a keen focus on data labeling for training advanced machine learning models.
Encord is a comprehensive data engine and software stack solution designed to enhance and streamline your machine learning (ML) workflow.
The platform comprises three key components: Annotate, Active, and Apollo.
This robust tool empowers users to establish labeling workflows and convert unlabeled data into labeled datasets.
The ability to label data through object boundaries and classification labels facilitates the review process using both manual and automatic quality assurance methods.
The platform simplifies the creation of high-quality labeled datasets which is a critical step in training ML models.
Let's see its advantages and disadvantages below:
Pros
1. User Experience
Intuitive Interface - User-friendly and easy navigation.
Hotkeys- Accelerate annotation processes.
Auto-Saving Feature- Prevents data loss.
Communication- Seamless interaction between engineers and annotators.
2. Collaboration and Productivity
Collaboration: Enhances accuracy through collaborative features.
Workflow Management System: Efficiently organizes clinical ML workflows.
Task Management and Automation: Simplifies complex workflows.
Annotation Tools
Robust Annotation Interface: Quick and efficient annotation capabilities.
Handles Large Taxonomies: Manages comprehensive labeling taxonomy.
Range of Segmentation Tools: Offers a variety, including freehand drawing.
3. Technical and Integration Capabilities
Well-documented APIs: Ensures easy integration with other systems.
DICOM File Support: Essential for radiological studies.
Streamlined Data Pipelines: Allows seamless integrations via a flexible API.
Integration with SAM: Seamless and cost-effective.
Customizable Dashboards and Reports: Enhanced reporting capabilities.
Cons
1. Limited Customization
Lacks personalization options for hotkeys and tool settings.
2. Bugs
Users encountered initial bugs with the new workflow feature (though these were sorted later).
3. Lag and Latency
Some users faced occasional slow production due to these issues.
4. Web-based Limitations
Being web-based, the platform is susceptible to lag or latency issues due to internet connection.
5. Learning Curve
Initial setup and learning might be challenging due to some missing documentation.
6. Lack of Certain Features
It does not currently support native model-assisted labeling on medical imagery.
7. No Mobile App
Users can’t access the platform on mobile devices.
8. Incomplete SDK
Some users found that a few functions were missing from the Python SDK compared to the API.
Due to these considerations, we will investigate alternative options to Encord.
We will be looking at other options to find the best fit.
Let's check out Labellerr, Appen, V7 Labs, Hive, Dataloop and SuperAnnotate to see what they offer and which one is good for different needs.
Table of Contents
1. Labellerr
Labellerr is a data annotation platform that makes it easier for AI teams to prepare high-quality labels for their Vision, NLP, and LLM models.
With Labellerr, the process becomes an astounding 99 times faster, making AI deployment-ready in a fraction of the time.
Key Highlights One should know about Labellerr
1. Data Pipeline with Ease
Labellerr's data labeling engine leverages automated annotation, advanced analytics, and intelligent quality assurance.
It processes millions of images and thousands of hours of videos in just a few weeks, ensuring a seamless and efficient data pipeline.
2. Rapid Deployment
Its commitment to speed is evident in its quick setup and the provision of a free 14-day pilot with no credit card required.
The platform empowers AI teams to kickstart their projects promptly.
3. Trusted by Industry Leaders
Labellerr boasts impressive ratings on platforms like Capterra and G2, earning the trust of renowned companies like Intuition Robotics, Perceptly, Scientiamobile, Tripscout, Wadhwani, Skintelligent, Mira_Insight, Adobe, University of Maryland, and many more.
Unmatched Performance of Labellerr
1. Train High-Quality ML Models
Labellerr guarantees 99% accurate labels, leading to a remarkable reduction in time to data preparation and development costs.
The platform is a game-changer for achieving unparalleled precision in model training.
2. User-Friendly Workflow
Labellerr simplifies the labeling process with a straightforward workflow:
-> Connect data to the engine seamlessly.
-> Create and manage projects effortlessly.
-> Export labeled data in various formats with just one click.
Advanced Features of Labellerr
1. Automated Labeling
Speed up the labeling process with prompt-based labeling, model-assisted labeling, and active learning-based automation.
2. Multiple Data Types Support
Handle images, videos, PDFs, text, or audio without switching tools.
3. Smart QA
Pre-trained model-based and ground truth-based quality assurance ensures top-notch label quality.
4. MLOps Integration
Streamline AI infrastructure with extensive integration support for platforms like GCP Vertex AI and AWS Sagemaker.
5. 24/7 Support
Enjoy round-the-clock tech support on crucial projects, ensuring the fastest response time.
Hence, Labellerr stands out as the most powerful and advanced platform for AI data labeling, offering unmatched speed, reliability, and user satisfaction.
The platform's commitment to excellence has garnered praise from industry leaders and users alike, making it an indispensable tool for any AI team.
2. Appen
Appen is a data labeling services platform that has been in operation since 1996, making it one of the pioneers in the industry.
Known for its extensive experience, Appen helps various sectors by offering data labeling services.
In 2019, the company acquired Figure Eight, enhancing its software capabilities to assist businesses in training and refining their computer vision models.
Appen's Image Annotation Tool boasts key features such as support for multiple annotation types (bounding boxes, polygons, and image segmentation), data sourcing with pre-labeled datasets, data preparation, and real-world model evaluation.
Additionally, it provides natural language processing functionalities and supports broader text-to-speech applications.
It is ideal for teams seeking image data sourcing and collection alongside annotation services. Pricing is available through their enterprise plan.
Annotation Capabilities
Appen is facilitating swift and scalable data annotation through Smart Labeling.
1.Image Annotation Capabilities
High-quality data annotation plays a pivotal role in the successful training of AI/ML models, serving as the foundation for informed decision-making.
The platform seamlessly integrates human intelligence at scale with cutting-edge models to annotate diverse raw data, including text, video, and audio, thereby establishing the accurate ground truth essential for robust model performance.
Appen offers a comprehensive suite of annotation capabilities covering image annotation, video annotation, and data labeling services, addressing various needs such as collection, classification, annotation, transcription, and translation.
2. Smart Labeling
Its Smart Labeling leverages innovative capabilities, incorporating Machine Learning assistance to enhance the efficiency, quality, and delivery of data collection and annotation projects.
Smart Labeling focuses on three key areas to drive improvements in the data annotation process:
-> Pre-Labeling
Machine Learning provides an initial 'best guess' hypothesis before contributors commence the task.
Human reviewers then refine pre-processed annotations, significantly reducing the time required for data annotation.
-> Speed Labeling
Machine Learning contributes to in-tool efficiency, quality, and accuracy, creating ergonomic conditions that enhance contributor productivity.
This results in reduced cognitive strain, enabling contributors to work faster without compromising accuracy.
-> Smart Validators
Machine Learning models verify human judgments before finalization, ensuring payment is made only for high-quality judgments.
This eliminates the need for peer reviews and mitigates the risk of paying for judgments that do not align with project requirements.
Appen's Smart Labeling not only enables rapid and scalable model deployment but also provides assurance through qualified contributors who oversee and correct judgments as needed.
Appen Annotation Tools
1. Image Annotation Tools
It supports a wide range of computer vision tools for image and video annotation, including object tracking, pixel-level semantic segmentation, and image transcription.
Their image annotation services and video annotation tools are designed to facilitate the integration of model hypotheses, expediting the labeling process and enhancing model validation.
2. Text Annotation and Translation
The platform offers large-scale text annotation and NLP labeling, encompassing named entity recognition and parts of speech labeling.
These tools support the incorporation of model hypotheses for faster annotation and improved model validation.
Additionally, they provide computer-aided translation for machine learning projects.
3. Audio Annotation and Transcription
Their scalable audio data pipeline includes collection, segmentation, event labeling, and transcription.
All of their data science tools in this domain support the integration of model hypotheses, facilitating faster annotation and robust model validation.
4. Data Collection and Enrichment
Appen supports an extensive data collection pipeline for audio, websites, text, and images.
Use cases include training data creation for ASR and text-based conversational agents.
Their ML models validate the quality of human-submitted input, ensuring the completion of broad-scale data collection projects with efficiency.
5. Data Classification
Whether conducting sentiment analysis, content moderation, or search relevance tuning, the platform offers large-scale data classification pipelines to meet diverse needs.
Proprietary quality control technologies guarantee accuracy and precision exceeding 95%, requiring minimal effort.
3. V7 Labs
V7 Labs, a UK-based platform established in 2018, enables teams to annotate training data, support human-in-the-loop processes, and connect with annotation services.
V7 provides annotation for various data types, including documents and videos, alongside its image annotation tooling.
Key features include project management, workflow automation, real-time collaboration, tagging, and model-assisted annotation for multiple types.
V7 is suitable for students or teams looking for a versatile platform to annotate different data types. Pricing options include academic, business, and pro plans.
Let's see V7's features, including Smart Labeling, AutoAnnotate, and its diverse annotation tools.
Smart Labeling
V7's Smart Labeling incorporates Machine Learning assistance to enhance productivity, quality, and project delivery.
The three key areas it focuses on are Pre-Labeling, Speed Labeling, and Smart Validators.
Pre-Labeling leverages Machine Learning for an initial 'best guess' hypothesis, reducing annotation time.
Speed Labeling improves efficiency and accuracy within the tool, enhancing contributor productivity.
Smart Validators ensure quality judgments, eliminating the need for peer reviews.
Auto-annotate Feature
V7's auto-annotate feature accelerates the annotation process by generating pixel-perfect polygon masks rapidly.
This feature, powered by enhanced auto-annotate, object recognition, and adaptive learning, ensures high-speed annotation without compromising quality.
It proves particularly useful for computer vision and generative AI tasks.
Dataset Management and CLI/SDK
V7 provides a centralized platform for gathering training data, ensuring that machine learning teams can manage their valuable assets efficiently.
The Command Line Interface (CLI) and Software Development Kit (SDK) enable users to perform actions on assets conveniently, allowing for dataset management via a single command.
Model Management and Workflows
V7's Model Management facilitates building a library of models, whether using pre-trained models or bringing your own.
The platform allows users to combine models, humans, and data in bespoke workflows, creating efficient assembly lines for training data.
Flexible routing of training data ensures maximum efficiency and accuracy.
Industry-Specific Tools and Integrations
V7 caters to various industries, including healthcare, insurance, finance, life sciences, logistics, and retail, with specialized tools like DICOM Annotation for medical imaging.
The platform seamlessly integrates with various ML-Ops platforms, cloud storage services, and deep learning frameworks.
4. Hive
Hive, founded in 2013, offers cloud-based AI solutions for content labeling across diverse data types such as images, video, audio, and text.
The Hive Image Annotation Tool includes features like integrated image annotation, support for unique image annotation use cases (such as ad targeting and semi-automated logo detection), and flexible access to model predictions with a single API call.
Teams engaged in content moderation and labeling find Hive beneficial.
Pricing is available through their enterprise plan.
How Customers Leverage Hive Enterprise Data Annotation Service
1. Data Collection for OCR Model Development
Hive plays a crucial role in supporting a client focused on developing a production Optical Character Recognition (OCR) model.
The team sourced and processed over 650,000 documents in more than 12 languages, laying the foundation for robust OCR model training.
2. Data Annotation for Autonomous Vehicles
In collaboration with a leading autonomous vehicle company, Hive successfully labeled 400,000+ images.
The result was the generation of 10 million bounding boxes and 30 million classification attributes, providing vital annotated data for training sophisticated autonomous systems.
How Clients Engage with Hive
1. High Throughput with a Global Workforce
Hive boasts a vast and globally distributed workforce, with over 5 million registered workers on their labeling platform.
This expansive network enables them to label over 10 million items daily, ensuring high throughput for your annotation needs.
2. High Accuracy through Independent Corroboration
Ensuring the accuracy of labeled data is paramount.
Every task is sent to multiple contributors for independent corroboration of results, enhancing the reliability of annotations.
Rigorous quality assurance processes are in place, with every project carefully examined before delivery.
3. Adherence to High Standards
Hive prioritizes maintaining high standards in data annotation.
Thorough instructions and safeguards are provided to the labeling contributors, ensuring a shared understanding of quality requirements.
This commitment to excellence guarantees that the labeled data meets and exceeds industry standards.
5. Dataloop
Dataloop, based in Israel, is another data labeling platform providing a comprehensive solution for data management and annotation projects.
Dataloop offers capabilities across images, text, audio, and video annotation, assisting businesses in training and enhancing their machine learning models.
The tool includes features for image annotation tasks like classification, detection, and semantic segmentation, with support for video annotations.
It is suitable for teams with diverse data annotation needs.
Dataloop offers a free trial and an enterprise plan.
Dataloop goes beyond being a data labeling service provider by offering a comprehensive set of tools designed to manage data workflows and establish (semi-) automated deployment pipelines for new models.
This capability empowers machine learning (ML) teams to iterate on models swiftly, minimizing deployment risks.
Here are essential aspects to understand about Dataloop:
Data Labeling Support
Dataloop supports annotations for both images and videos.
The platform incorporates automation annotation tools, including an AI assistant that can automatically convert four single points into multi-vertice polygons.
It also features model-assisted labeling capabilities similar to Labelbox and smart object tracking for auto-duplicating annotations between video frames and sequenced images.
Robust Data Labeling Toolkit
Dataloop's toolkit for data labeling is robust, offering a variety of features.
However, it's important to note that interpolation outside of bounding boxes for videos is not provided.
Users should be aware of this limitation, particularly if their projects involve detailed video annotation requirements.
Accelerated Data Labeling
To expedite the data labeling process, Dataloop allows users to enhance their workforce by hiring additional individuals from Dataloop's fully managed workforce network.
This approach ensures scalability and efficiency in handling large-scale annotation tasks.
Pricing Model
Dataloop operates on an on-demand pricing model, providing flexibility for users based on their specific needs.
This approach allows users to manage costs more effectively and align expenses with their project requirements.
6. SuperAnnotate
SuperAnnotate empowers enterprises to construct datasets and establish successful machine learning pipelines with annotation capabilities, data curation, and quality assurance tools.
It has automation features, native integrations, and robust data governance.
It is a leading platform dedicated to accelerating the development and management of AI models by providing state-of-the-art tools for building, fine-tuning, iterating, and overseeing high-quality training data.
SuperAnnotate's Annotation Tool
SuperAnnotate's cornerstone is the annotation tool, designed to annotate various data types such as images, videos, text, audio, and more. The platform ensures:
1. Fast Annotation without Compromising Quality
Accelerate annotation processes without sacrificing accuracy.
2. Collaborative Annotation
Collaborate with stakeholders to enhance annotation precision.
3. Automation and Streamlining
Automate and streamline annotation processes for efficiency.
Annotation Tools for Different Data Types
SuperAnnotate provides specialized annotation tools for different data types:
1. Image Annotation Tool
Enhance image annotation accuracy with tools for object detection, classification, pose estimation, OCR annotation, segmentation, and more.
2. Video Annotation Tool
Annotate videos in real-time for object tracking, classification, instance segmentation and tracking, action detection, pose estimation, and lane detection.
3. Text Annotation Tool
Extract valuable information from text data for tasks like sentiment analysis, summarization, classification, translation, question answering, and named-entity recognition.
4. Audio Annotation Tool
Transcribe and segment audio data for tasks like speech recognition, speaker recognition, sound event detection, and classification.
5. LiDAR Annotation Tool
Create accurate LiDAR annotations for 3D object detection and tracking, providing detailed views of 3D point cloud data.
6. Classification Tool
Classify PDFs, HTML files, and websites for various use cases such as topic labeling, categorization, content analysis, question answering, sentiment analysis, and summarization.
Powering Diverse Industries and Use Cases
SuperAnnotate caters to a wide array of industries and use cases, including agriculture, healthcare, insurance, sports, robotics, autonomous driving, aerial imagery, NLP, security, and surveillance.
Conclusion
Data labeling plays a crucial role in advancing artificial intelligence, especially in machine learning.
Encord, with its Annotate, Active, and Apollo components, offers a comprehensive solution to streamline ML workflows.
While it provides several advantages like user-friendly interfaces, collaboration features, and robust annotation tools, there are also considerations such as limited customization and occasional lag.
Exploring alternatives like Labellerr, Appen, V7 Labs, Hive, Dataloop, and SuperAnnotate reveals diverse options with unique strengths.
Labellerr stands out for its speed, reliability, and advanced features, making it a powerful choice for AI data labeling.
Each platform has its merits, catering to specific needs across industries, and reaffirming the importance of choosing the right tool based on project requirements.
Frequently Asked Questions
1. What is Encord?
Encord is a versatile data annotation platform that serves as a comprehensive data engine and software stack solution.
It comprises three main components: Annotate, Active, and Apollo.
The Annotate component of Encord is a robust tool designed for data annotation purposes. It empowers users to establish labeling workflows and efficiently convert unlabeled data into labeled datasets.
With Annotate, users can label data with precision, incorporating object boundaries and classification labels.
The tool also facilitates the review of labels through both manual and automatic quality assurance methods.
In essence, Encord, with its Annotate component, is a powerful data annotation platform that simplifies and enhances the creation of high-quality labeled datasets crucial for training machine learning models.
2. How can Encord help you build a computer vision model?
Bid farewell to fragmented workflows, disparate annotation tools, and the complexity of using notebooks for constructing computer vision models.
Encord emerges as a transformative active learning platform, revolutionizing each phase of the journey towards deploying a computer vision model into production.
This innovative platform transcends the challenges associated with disjointed processes and cumbersome tools, offering a seamless and accelerated approach.
Encord's active learning capabilities play a pivotal role in optimizing the entire lifecycle of a computer vision model.
By intelligently selecting and annotating data, it ensures an iterative enhancement of the model's performance, leading to efficiency and precision at every stage.
3. What is Encord's DICOM annotation tool?
Encord's DICOM annotation tool provides an integrated and comprehensive solution for managing medical imaging data and assessing model performance.
This tool is designed specifically for DICOM files, which are common in medical imaging.
With this DICOM annotation tool, users can capture the shape of pathological features in medical images.
Notably, the tool employs interpolation to accurately label slices that annotators may have skipped during the annotation process.
This feature ensures a thorough and precise representation of pathology in the annotated data.
Overall, Encord's DICOM annotation tool serves as a one-stop solution, offering efficient data management and insights into model performance, enabling the establishment of valuable feedback mechanisms for continuous improvement of both data and machine learning models.

Simplify Your Data Annotation Workflow With Proven Strategies
.png)
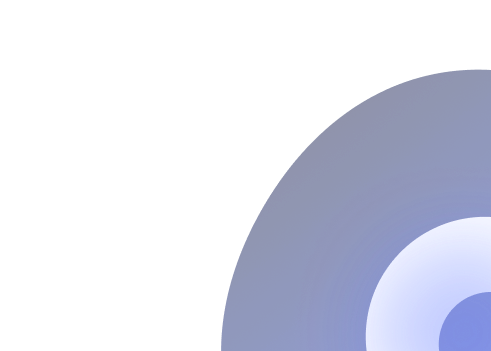