10 Best Computer Vision Datasets Published In CVPR 2022-Part 1
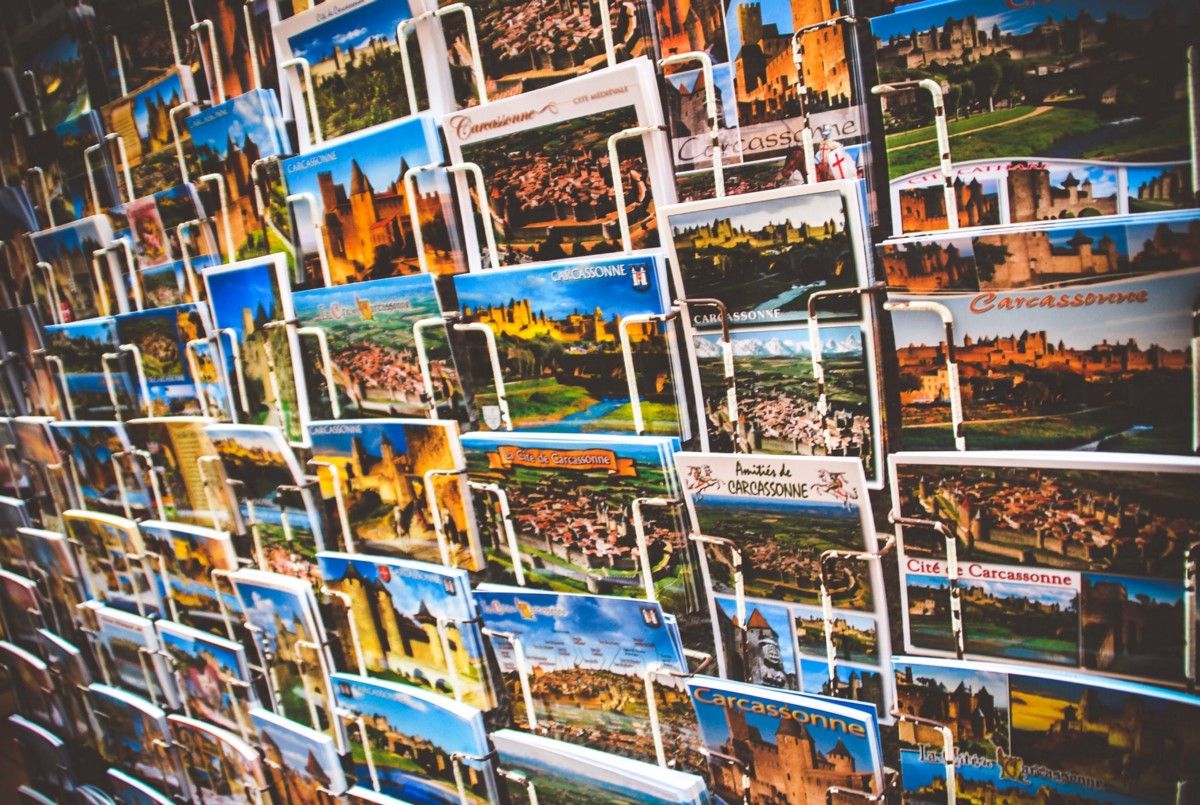
Are you someone who deals with datasets every new day and are always looking for a new variety of datasets? If you are a data enthusiast, then CVPR ( IEEE/CVF Computer Vision and Pattern Recognition Conference (CVPR)) is something that is extremely important for you. Recently, they have published a list of computer vision datasets that might be of your interest if you are related to the Computer Vision industry.
Here we have listed some of the top computer vision datasets that might be of use to you. But first, let’s understand CVPR in detail.
About CVPR
The major annual computer vision conference, known as the IEEE/CVF Computer Vision and Pattern Recognition Conference (CVPR), includes both the leading panel and a number of concurrent workshops and short courses. It offers amazing value for students, scholars, and business researchers due to its great quality and low price.
In 2022, CVPR will offer a hybrid conference both in-person and online attendance options. Only attendees who have registered for the CVPR will have access to conference content that is available on the virtual network. The CVF website will host the conference proceedings, and following the conference, the final copy will be posted to IEEE Xplore.
Recent computer vision datasets published in CVPR 2022 are (not any particular order)
1. 360MonoDepth: High-Resolution 360° Monocular Depth Estimation
Author: Manuel Rey-Area, Mingze Yuan, Christian Richardt
360° imagery is appealing for several computer vision tasks because 360° cameras can record entire scenes in a single picture. Monocular depth estimation for 360-degree data is still difficult, especially at high dimensions like 2K (20481024) and more, which are crucial for novel view generation and applications in virtual reality. Due to a lack of GPU RAM, current CNN-based techniques cannot support such high resolutions. In this research, they have provided a flexible model for tangent pictures to estimate monocular depth from high-resolution 360° photos.
They created perspective views by projecting the 360° image representation onto a series of tangent planes, which are appropriate for the most recent, most precise, state-of-the-art point-of-view monocular depth estimators. They integrated the individual depth estimations again using deformable multi-scale alignment and gradient-domain blending to provide globally consistent disparity estimates. The end result is a detailed, dense 360° depth map that supports outdoor scenarios that are not currently handled by existing techniques.
They have provided two datasets:
- Matterport3D 360º RGBD Dataset that consists of 9684 RGB-D pairs from the original Matterport3D. The resolution is 2048x1024. All the details and use instructions are located at this README.md
- Replica 360º 2k/4k RGBD: consists of 130 RGB-D pairs render at 2048x1024 and 4096x2048.
You can download the dataset from here
Related Papers for your reference
Deep learning methods for 360 monocular depth estimation and point cloud semantic segmentation that is provided by: University of Missouri: MOspace | Publisher: 'University of Missouri Libraries' | Year: 2022 by Li Yuyan
360MonoDepth: High-Resolution 360° Monocular Depth Estimation that is provided by: University of Bath Research Portal | Publisher: 'Institute of Electrical and Electronics Engineers (IEEE)' | Year: 2022 by Rey-Area Manuel, Yuan Mingze, Richardt Christian
Neural Contourlet Network for Monocular 360 Depth Estimation that is provided by: arXiv.org e-Print Archive | Publisher: 'Institute of Electrical and Electronics Engineers (IEEE)' | Year: 2022 by Shen Zhijie, Lin Chunyu, Nie Lang, Liao Kang, Zhao Yao
Estimation of Depth Maps from Monocular Images using Deep Neural Networks that is provided by: Universidad Carlos III de Madrid e-Archivo | Year: 2019 by Sarmiento Rocha Daniel
2. De-Rendering 3D Objects in the Wild
Author: Felix Wimbauer, Shangzhe Wu, Christian Rupprecht
The need for algorithms that can transform objects from pictures and videos into depictions appropriate for a wide range of related 3D tasks has increased as attention has turned to virtual and augmented reality (XR) applications. We cannot exclusively rely on supervised learning due to the large-scale deployment of XR applications and devices because it is not practical to collect and annotate data for the infinite diversity of real-world objects. A single image of an object can be broken down into its geometry (depth and randoms), material (albedo, reflectivity, and shininess), and global illumination characteristics using a weakly supervised method that we provide. The strategy bootstraps the learning process for training just using a crude initial shape approximation of the training objects.
This research explores the challenge of teaching to de-render pictures of common items "in the wild," which resides at the nexus of numerous computer vision and visual effects subfields. We will first go over pertinent research on intrinsic image decomposition, inverse rendering from multiple pictures, direct supervision, and current unsupervised techniques in this part.
The dataset:
- ShapeNet: The repository contains over 300M models with 220,000 classified into 3,135 classes arranged using WordNet hypernym-hyponym relationships. ShapeNet Parts subset contains 31,693 meshes categorised into 16 common object classes (i.e. table, chair, plane etc.). Each shape ground truth contains 2-5 parts (with a total of 50 part classes).
- The CelebA-HQ dataset is a high-quality version of CelebA that consists of 30,000 images at 1024×1024 resolution.
You can download this dataset from here
3. Self-Supervised Material and Texture Representation Learning for Remote Sensing Task
Author: Peri Akiva, Matthew Purri, Matthew Leotta
The goal of self-supervised learning is to acquire representations of visual features without the aid of human-annotated labels. In order to get relevant initial network weights that help with faster convergence and better performance of downstream tasks, it is frequently utilised as a precursor step. Even though self-supervision eliminates the need for labels and bridges the domain gap between supervised and unsupervised learning, the self-supervised objective still necessitates a strong inductive bias toward downstream tasks for efficient transfer learning. They have presented our MATTER (MATerial and TExture Representation Learning) material and texture-based self-supervision approach, which draws inspiration from traditional material and texture methodologies.
Any surface's material and texture, as well as its tactile characteristics, colour, and specularity, can be used to properly characterise it. Effective depiction of material and texture can, therefore, also describe various semantic classes that are closely related to the material and texture in question. In order to establish consistency in the portrayal of materials and textures, MATTER uses multi-temporal, spatially aligned remote sensing imagery across unchanging regions to learn invariance to illumination and viewing angle. They have demonstrated that on change detection, land cover classification, and semantic segmentation tasks, our self-supervision pre-training strategy enables up to 24.22% and 6.33% performance increases in unsupervised and fine-tuned setups, as well as up to 76% faster convergence.
The dataset:
BigEarthNet consists of 590,326 Sentinel-2 image patches, each of which is a section of i) 120x120 pixels for 10m bands; ii) 60x60 pixels for 20m bands, and iii) 20x20 pixels for 60m bands.
You can download this dataset from here
Related Papers for your reference
Cloud Segmentation and Classification from All-Sky Images Using Deep Learning Provided by: Institute of Transport Research: Publications | Year: 2020 by Fabel Yann
Self-supervised Learning in Remote Sensing: A Review Provided by: arXiv.org e-Print Archive | Year: 2022 by Wang Yi, Albrecht Conrad M, Braham Nassim Ait Ali, Mou Lichao, Zhu Xiao Xiang
When Does Contrastive Visual Representation Learning Work?
Provided by: Edinburgh Research Explorer | Publisher: 'Institute of Electrical and Electronics Engineers (IEEE)' | Year: 2022 by Cole Elijah, Yang Xuan, Wilber Kimberly, Aodha Oisin Mac, Belongie Serge
Learning Generalizable Visual Patterns Without Human Supervision Provided by: BORIS Theses | Publisher: Universität Bern by Jenni Simon
4. FERV39k: A Large-Scale Multi-Scene Dataset for Facial Expression Recognition in Videos
Author: Yan Wang, Yixuan Sun, Yiwen Huang, Zhongying Liu, Shuyong Gao, Wei Zhang, Weifeng Ge, Wenqiang Zhang
There are few datasets available for FER in movies, and current benchmarks for FER primarily focus on static images. Evaluating whether current approaches continue to function satisfactorily in application-oriented real-world settings is still unclear. The "Happy" expression, for instance, is more discriminating when it is high intensity in a talk show than when it is low intensity in an official event. They have created the FERV39k dataset, a sizable multi-scene dataset, to close this gap. In three different ways, we examine the crucial components of building such a novel dataset: (1) multi-scene hierarchies and expression class; (2) production of candidate video clips; and (3) a reliable hand labelling procedure.
They chose 4 scenarios that were each divided into 22 scenes based on these rules, annotated 86k samples automatically extracted from 4k movies using the well-designed methodology, and then created 38,935 video clips that were each annotated with 7 iconic expressions. Experiment benchmarks for four alternative baseline framework types were also offered, along with additional analysis of their performance in various settings and significant difficulties for future research. Additionally, using ablation studies, we thoroughly analyse important DFER components. Both our project and the fundamental framework will be accessible.
The Dataset:
Aff-Wild is a dataset for emotion recognition from facial images in a variety of head poses, illumination conditions and occlusions.
You can download this dataset from here
Related Papers for your reference
Coarse-to-Fine Cascaded Networks with Smooth Predicting for Video Facial Expression Recognition published on 24 Mar 2022 by Fanglei Xue, Zichang Tan, Yu Zhu, Zhongsong Ma, Guodong Guo
Video-Based Frame-Level Facial Analysis of Affective Behavior on Mobile Devices Using EfficientNets by Savchenko A.V.
Frame-level Prediction of Facial Expressions, Valence, Arousal and Action Units for Mobile Devices published on 25 Mar 2022 by Andrey V. Savchenko
5. Weakly Supervised Semantic Segmentation Using Out-of-Distribution Data
Author: Jungbeom Lee, Seong Joon Oh, Sangdoo Yun, Junsuk Choe, Eunji Kim, Sungroh Yoon
The foundation of weakly supervised semantic segmentation (WSSS) techniques is frequently a classifier's pixel-level localization maps. The performance of WSSS is fundamentally constrained when classifiers are trained solely on class labels because of the erroneous association between frontal and background stimuli (such as train and rail). There have been prior attempts to solve this problem with more oversight. They have suggested Out-of-Distribution (OoD) data, or photos lacking foreground item classes, as a fresh source of information to identify the foreground from the background. They have used the hard OoDs for which the classifier is most likely to predict false positives.
These samples generally contain prominent background visual cues (such as a rail) that classifiers frequently mistake for the foreground (such as a train). As a result, these signals enable classifiers to appropriately suppress erroneous background cues. Obtaining such hard OoDs simply adds a little amount of image-level labelling expenses on top of the initial efforts to gather class labels, and does not necessitate a significant amount of annotation work. We offer a technique for making use of the hard OoDs called W-OoD. W-OoD performs at the cutting edge on Pascal VOC 2012.
The dataset:
The PASCAL Visual Object Classes (VOC) 2012 dataset includes 20 object categories, such as household items, domestic animals, and others: an aeroplane, a bicycle, a boat, a bus, a car, a motorbike, a train, a bottle, a chair, a dining table, a potted plant, a sofa, a TV/monitor, a bird, a cat, a cow, a dog, a horse, a sheep, This dataset includes bounding box, object class, and pixel-level segmentation annotations for each image. For tasks including object detection, semantic segmentation, and classification, this dataset has been frequently utilised as a standard. Three subsets of the PASCAL VOC dataset were created: a private testing set, 1,449 images for validation, and 1,464 images for training.
You can download this dataset from here
Related papers for your reference
Searching for Efficient Multi-Scale Architectures for Dense Image Prediction Published on NeurIPS 2018 by Liang-Chieh Chen, Maxwell D. Collins, Yukun Zhu, George Papandreou, Barret Zoph, Florian Schroff, Hartwig Adam, Jonathon Shlens
Rethinking Atrous Convolution for Semantic Image Segmentation Published by Liang-Chieh Chen, George Papandreou, Florian Schroff, Hartwig Adam
Auto-DeepLab: Hierarchical Neural Architecture Search for Semantic Image Segmentation published on CVPR 2019 by Chenxi Liu, Liang-Chieh Chen, Florian Schroff, Hartwig Adam, Wei Hua, Alan Yuille, Li Fei-Fei
Encoder-Decoder with Atrous Separable Convolution for Semantic Image Segmentation Published on ECCV 2018 by Liang-Chieh Chen, Yukun Zhu, George Papandreou, Florian Schroff, Hartwig Adam
For next 5 datasets of the list continue reading our next blog - https://www.labellerr.com/blog/top-10-new-computer-vision-datasets-published-in-cvpr-2022-part-2/

Simplify Your Data Annotation Workflow With Proven Strategies
.png)
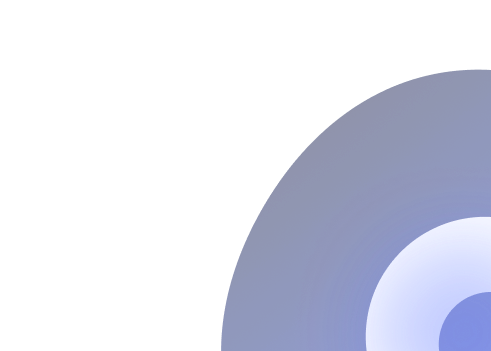