Avoid these mistakes while managing data labeling project
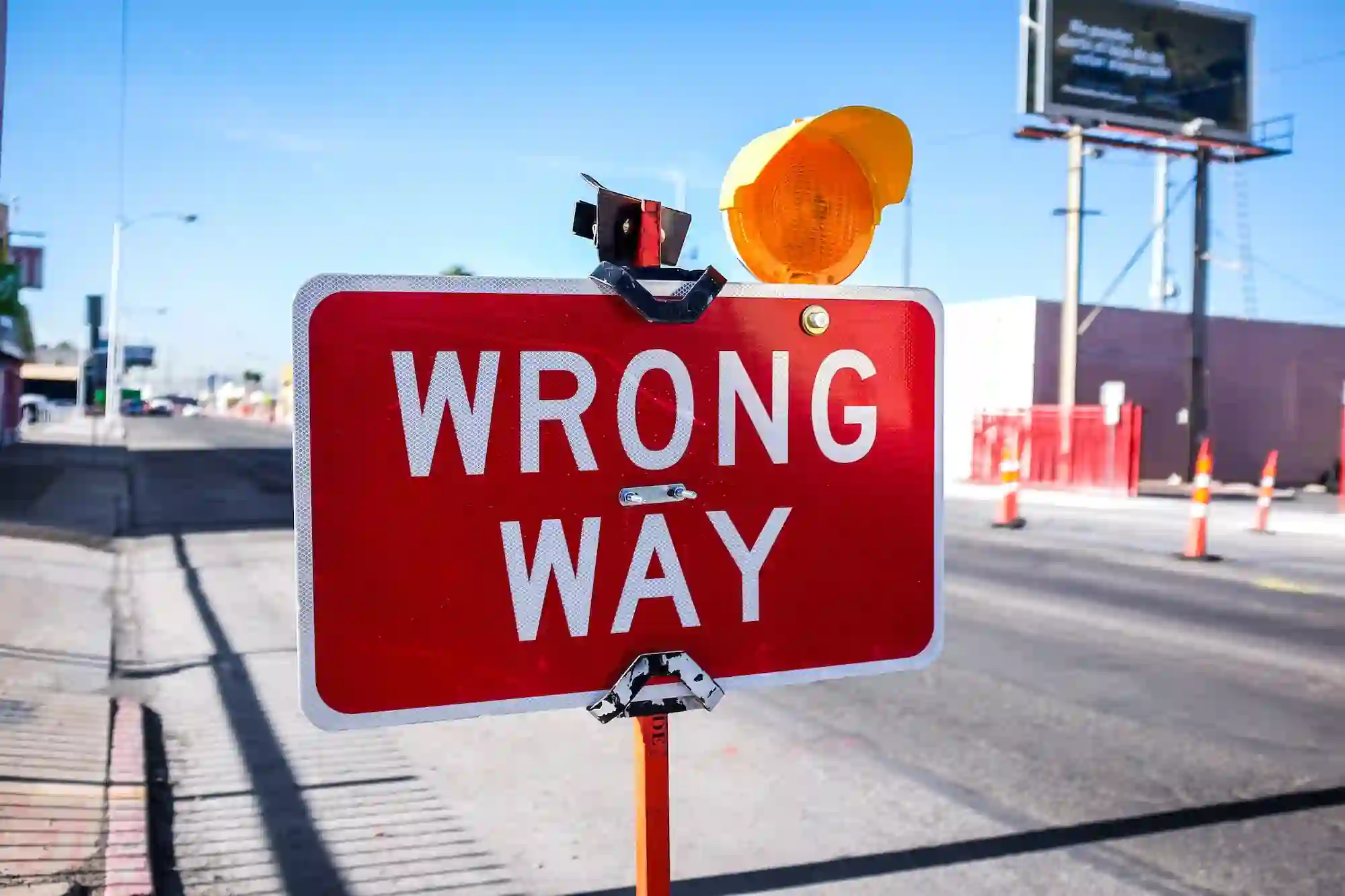
It is common that mistakes will occur if you are incorporating human force into the work. But when you are dealing with machines, it becomes hectic to undo the mistakes.
So, when you are dealing with a training machine, then it is recommended to avoid mistakes. Any error made during model training could have severe effects when your model is used to make important business choices.
The AI model is trained using a multi-stage process to make the greatest use of the training data and produce satisfying results and the ML team plays a crucial role in training them.
Here are some mistakes that should be avoided by the ML team to produce effective results while handling a data labeling project.
Table of Contents
- Lack of Data While Doing the Project
- Employing Unorganized and Unreliable Data
- Using an AI Model That Does Independent Learning
- Failure to Adhere to the Data Protection Guidelines
- Lack of Attention to Workforce Management
- Utilizing the Limited Training Data Sets
- Choosing the Incorrect Data Labeling Tools
- What is the Best Solution to Overcome These Mistakes?
Lack of data while doing the project
Data is necessary, but it must be pertinent to the objectives of the project. The data that the model is trained on needs to be labeled and accuracy-checked to produce reliable results.
You must provide an enormous amount of high-quality, pertinent data to an AI system if you want it to build a workable, dependable answer. And in order for the machine learning models to comprehend and correlate the numerous elements of data you supply, you must continuously feed them with this data.
The collection of relatively minimal data for less frequent variables is a potential problem in the data labeling process.
The deep learning AI model is not trained on some other less-common variables when you classify photos based on one frequently occurring factor in the raw documents. For deep learning models to function reasonably well, thousands of data points are required.
Employing unorganized and unreliable data
One of the most frequent errors made by machine learning engineers in the construction of AI systems is the use of unreliable and unstructured data.
Unverified data could contain flaws such as duplication, contradictory data, missing categories, inaccuracies, and other data problems that could lead to anomalies throughout the training process.
Therefore, thoroughly review your raw data collection before using it for machine learning training and remove any unnecessary or irrelevant information to assist your AI model function more accurately.
Using an AI model that does independent learning
However, you require professionals to train your AI model utilizing a huge number of training datasets. But this needs to be taken into account when training such models if AI is using a repetitive machine learning procedure.
As an ML team, you must confirm that the AI model is picking the appropriate learning approach. In order to receive the greatest results, you must routinely review the AI training phase and its outcomes at regular intervals.
However, you must constantly ask yourself crucial questions while creating a machine learning AI, such as: Are the data you are using coming from credible and trustworthy sources? Does the AI cover a diverse population, and are there any factors else that might be influencing the outcomes?
Failure to adhere to the data protection guidelines
As more businesses gather huge amounts of unstructured data, data security regulations will experience a significant uptick soon. Some of the worldwide data security regulatory requirements utilized by businesses include CCPA, DPA, and GDPR.
Because there are cases of personal data being present in the photographs when it comes to identifying unstructured data, the need for security requirements is gaining acceptance. It is crucial that the data is safe in addition to preserving the subjects' privacy.
The businesses must ensure that employees without security clearance do not have access to these data sets and are unable to move or otherwise tamper with them.
When data labeling duties are outsourced to third-party companies, security compliance emerges as a major pain point. The project becomes more complex because data security and labeling service providers must adhere to industry norms.
Lack of attention to workforce management
Large data sets of various types are necessary for machine learning models to function properly and account for every scenario. Successful picture annotation entails its own set of labor-management difficulties, though.
Managing a large staff that can manually analyze massive unstructured data collections is a significant challenge. Upholding excellent standards throughout the workforce is the next. Projects involving data annotation could run into a lot of problems.
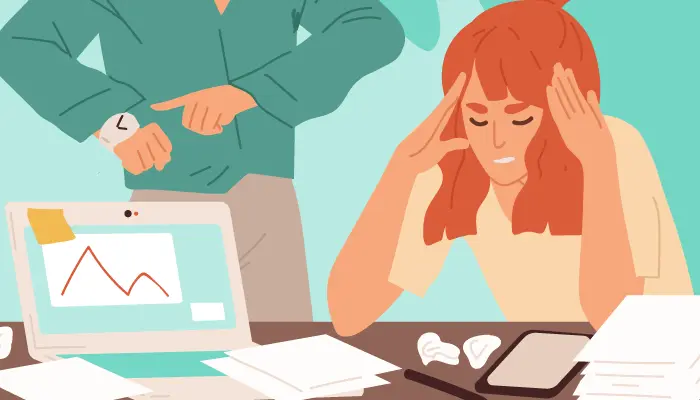
To overcome this mistake, you should provide training to the new team members, provide the instructions in documentation to make it easy for them to access, define the workflow, and help the team with technicalities.
Utilizing the limited training data sets
You must use the proper training data for your AI model for it to forecast with the best level of accuracy. One of the main causes of the model's failure is a lack of adequate data for training.
However, the fields of training data requirements differ depending on the kind of AI model or business. To ensure that deep learning can operate with high precision, you need to have more quantitative datasets in addition to qualitative datasets.
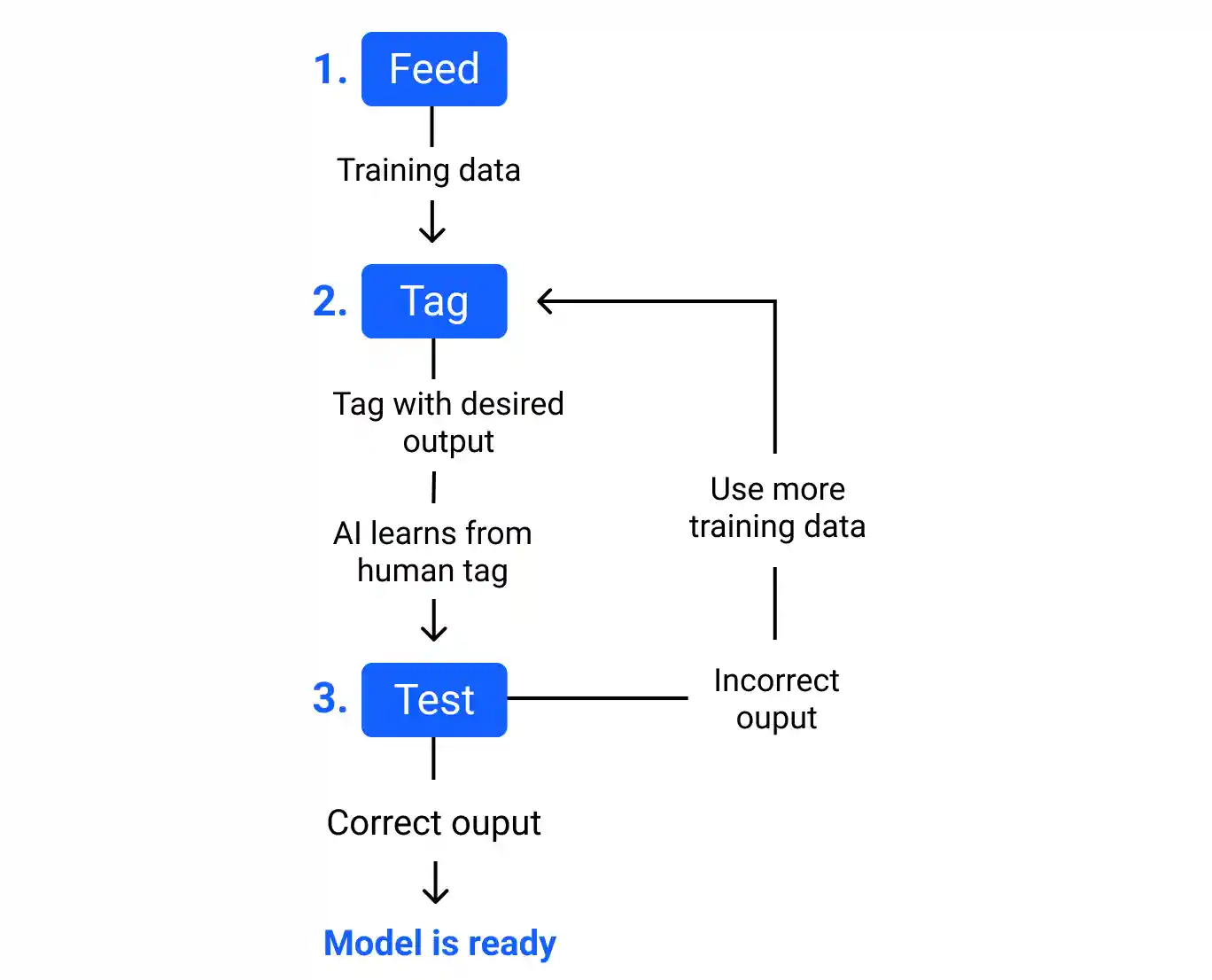
Choosing the incorrect data labeling tools
Various tooling methods are employed depending on the data set. We've seen that the majority of businesses concentrate on creating their own labeling tools as the first step in the deep learning process. But they quickly come to the realization that their tools are unable to keep up with the increased demand for annotation. Additionally, creating internal tools is costly, time-consuming, and essentially pointless.
It is wise to buy devices from a third party rather than taking the cautious route of manual labeling or spending in developing custom labeling solutions. This strategy only requires you to choose the appropriate tool depending on your requirements, the services offered, and scalability.
Well, what if we say that this mistake can be completely avoided if you go forward with us?
Labellerr is a computer vision automation tool that helps computer vision teams to simplify the manual mechanisms involved in the AI-ML product lifecycle. Platform design to make sure best practices are implemented at every stage of data preparation. You can try our tool to attain the best positive results.
What is the best solution to overcome these mistakes?
No mistake is done intentionally, if you want to avoid mistakes, then keep on looking for the right ways to do the tasks. When you know the right way, you avoid mistakes.
Keep checking the procedure and learn new methods and techniques to handle data labeling projects. So, after reading all the mistakes that are usually done by data annotators, you can check on the points in your data labeling project application.
To know further about data labeling and more, stay tuned!

Simplify Your Data Annotation Workflow With Proven Strategies
.png)
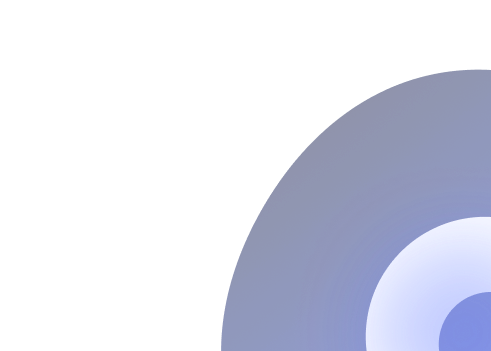