Future Prospects Of Image Annotation
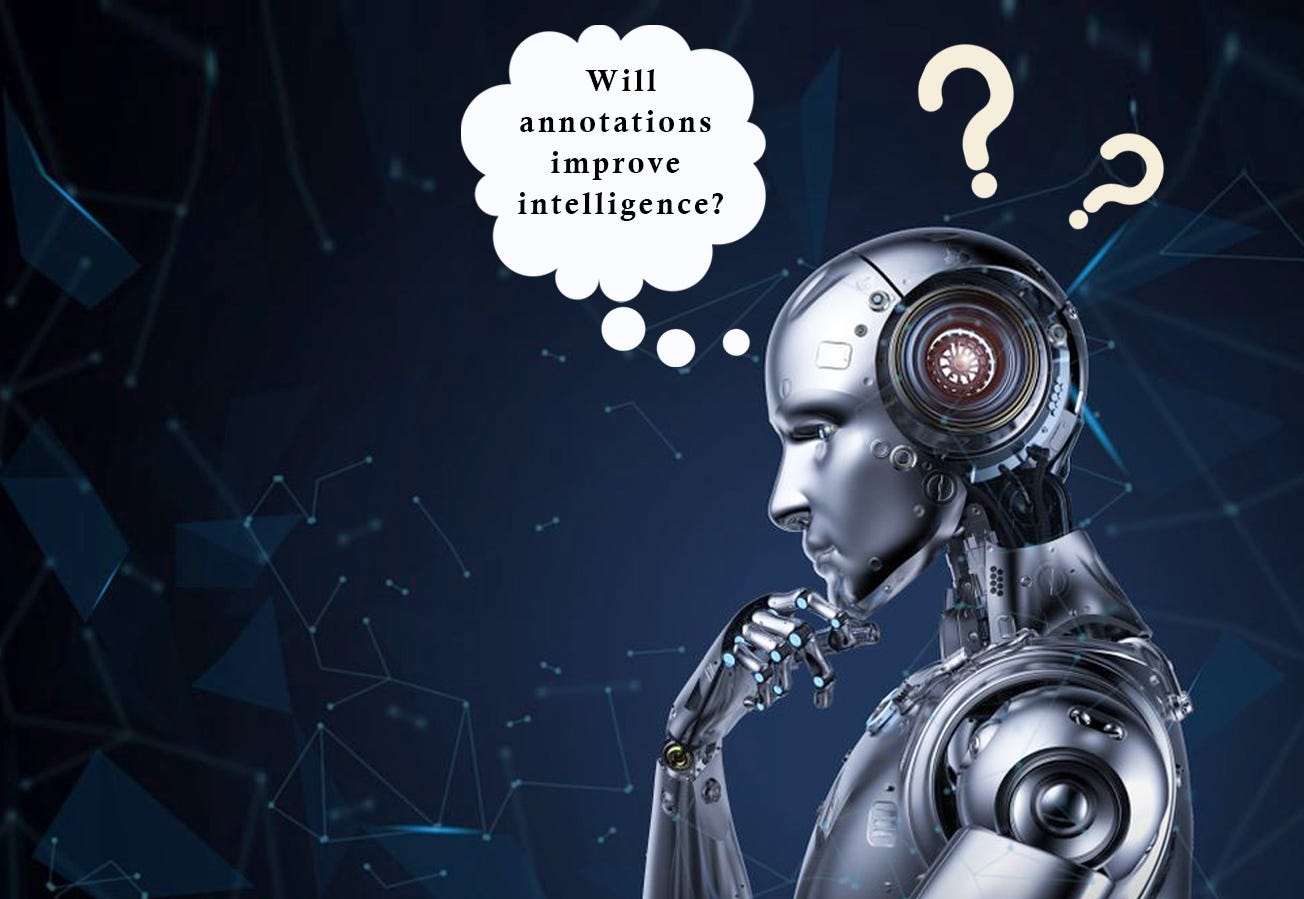
Image annotation involves labeling images within a dataset to train machine learning models. Once manual annotation is done, a machine learning or deep learning model processes the labeled images to replicate the annotations autonomously.
This sets the standards for the model, making precise image annotation crucial for training neural networks in computer vision. The autonomous labeling process is often referred to as model-assisted labeling.
Image annotations can be performed manually or using automated annotation tools. Auto annotation tools, pre-trained algorithms, are particularly useful for complex tasks like creating segment masks.
They assist with manual annotation by providing a starting point. Annotated data is essential for training supervised AI models, influencing how AI performs based on the quality of annotations. For unique problems and new domains, annotated data is crucial, as pre-trained models might not be readily available.
In this blog, we discuss the central role of Image Annotation in AI and its future prospects. We will summarise the information in short and precise forms, for people who would like to learn more about this can refer here.
Table of Contents
- The Central Role of Image Annotation in AI
- The Evolving Landscape of Image Annotation
- Future Trends and Innovations in AI Image Annotation
- Conclusion
- Frequently Asked Questions
The Central Role of Image Annotation in AI
Artificial Intelligence (AI) and Machine Learning (ML) are becoming increasingly fascinating for computer engineers, pushing the boundaries of this advancing technology into new areas and enhancing the efficiency of existing fields.
Figure: Image annotation in Computer Vision
Importance of Training Data
Having sufficient machine learning training data is essential to enhance AI performance. Image annotation, a technique in AI and ML, is crucial for creating training data, especially for visual perception models.
Understanding Image Annotation
To delve into unexplored AI applications, it's important to grasp the significance of image annotation. Annotated images are fundamental for training ML algorithms to recognize and predict objects in their surroundings.
Detecting Objects
In the realm of machine learning and AI, training machines to detect various objects in their natural environment is crucial. Annotated images enable objects of interest to be discernible to machines, aiding in developing AI models for industries like automotive and retail.
Classifying Objects
Image annotation facilitates object detection and plays a role in object classification. When different types of objects coexist in an image, annotation helps classify them, ensuring that the AI model can differentiate between similar objects.
Recognizing Object Classes
When an image contains diverse objects with similar dimensions, recognition becomes challenging. Object recognition and classification are necessary, and sem
antic segmentation is a valuable technique for classifying objects in a single class, aiding machines in distinguishing between various object types.
Supervised Machine Learning
Image annotation is crucial for creating labeled datasets in supervised machine learning. Annotated images assist algorithms in easily detecting and classifying objects, supporting both classification and regression algorithms for predicting values based on past data.
Figure: Supervised Learning
Model Validation Training Data
Another key role of image annotation is in model validation during AI or ML development. Annotated images are used to check whether a model can accurately detect, recognize, and classify objects.
This process involves validation by experienced annotators and engineers, ensuring accurate predictions, and evaluating the quality of data labeling services.
The Evolving Landscape of Image Annotation
In the domain of Artificial Intelligence and Machine Learning, data serves as the driving force behind innovation. Organizations often grapple with the challenge of analyzing vast amounts of raw and unstructured data, especially with the integration of AI-powered automation.
Labeled data emerges as a crucial component, laying the foundation for the development of successful models capable of accurate predictions, pattern identification, and correlation revelation.
Importance of Annotated Data
Machine Learning and Deep Learning models derive insights from previously annotated image and video datasets. These insights enable them to label or classify segments of new images and videos independently.
The efficacy of these algorithms directly correlates with the quality of their training data, emphasizing the pivotal role of annotated data as the cornerstone on which AI/ML models are constructed.
AI Image Annotation Services
Industries such as healthcare, automotive, and e-commerce highlight the significance of trends in the image annotation sector. For instance, in healthcare, precise labeling of medical images, including blood vessels and tumor boundaries, is critical for accurate diagnoses and subsequent treatments.
Figure: Image Annotation in the medical/healthcare sector
Properly tagged data enhances product recommendations and search engine results in e-commerce, while the automotive industry relies on labeled datasets for the development of self-driving cars.
Labellerr is one such AI-Automated Image Labelling Tool I use to gather labeled data.
Future Trends and Innovations in AI Image Annotation
In the section below, we discuss the future of the image annotation industry from various perspectives.
Automation Power
As the demand for annotated datasets grows, image annotation outsourcing companies increasingly embrace automation to scale their labeling operations.
Machine Learning algorithms, trained to recognize patterns independently, offer real-time and accurate labels. Automation reduces the time and resources required for manual annotation, enabling businesses to process more data with enhanced accuracy.
Multi-modal Data Annotation
Image annotation has expanded beyond visual data, incorporating other modalities like text, audio, and sensor data. Multi-modal annotation contributes to a more comprehensive understanding of content, context, and semantics, enhancing performance in applications such as image captioning, sentiment analysis, and image retrieval.
Transfer Learning
Transfer learning allows AI/ML models trained on one dataset to be reused for similar tasks or domains, minimizing the need for extensive manual annotation.
This approach reduces the labeled data required for training while increasing annotation accuracy, making it more feasible and cost-effective for businesses to adapt and customize image annotation solutions.
Figure: Transfer Learning
Synthetic Data Generation
The necessity for a diverse dataset for training Machine Learning models underscores the importance of synthetic data generation. AI algorithms are employed to create new data, substantially reducing the time and cost associated with manual annotation while providing ample high-quality data.
Conclusion
Image annotation is the cornerstone of AI and ML, driving innovation and efficiency across various industries. Precise annotation is pivotal for training neural networks and establishing standards for model accuracy.
Whether performed manually or with automated tools, annotated data is indispensable for training supervised AI models, especially in unique domains. This blog explores the central role of image annotation, emphasizing its significance in training models for object detection, classification, and recognition.
As the industry evolves, trends like automation of multi-modal data annotation are shaping the future. Annotated data's importance remains paramount, driving successful models and innovations in AI image annotation services.
Frequently Asked Questions
1. What is the future of Image Annotation?
What lies ahead for image annotation involves the emergence of sophisticated tools for annotating videos and 3D images, along with a growing prevalence of these formats across diverse industries.
The application of image annotation spans various sectors, including healthcare, retail, and autonomous vehicles, pointing towards increased usage in the future.
2. What is the use of Image Annotation?
The primary purpose of image annotation in computer vision is to identify objects, delineate boundaries, and segment images for tasks such as recognition, interpretation, or holistic image understanding.
Substantial datasets are essential to train, validate, and test machine learning models effectively for the desired outcomes to accomplish these objectives.

Simplify Your Data Annotation Workflow With Proven Strategies
.png)
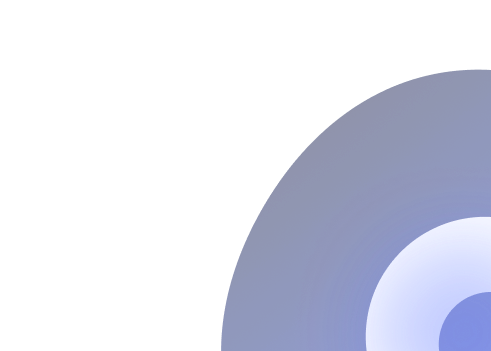