5 Best Generative AI Fine Tuning Tools in 2025
Top fine-tuning tools for generative AI in 2025 include Labellerr, Kili, Label Studio, Databricks Lakehouse, and Labelbox. These platforms streamline annotation, support multi-format data, integrate with ML models, and offer collaborative features to enhance model performance and scalability.
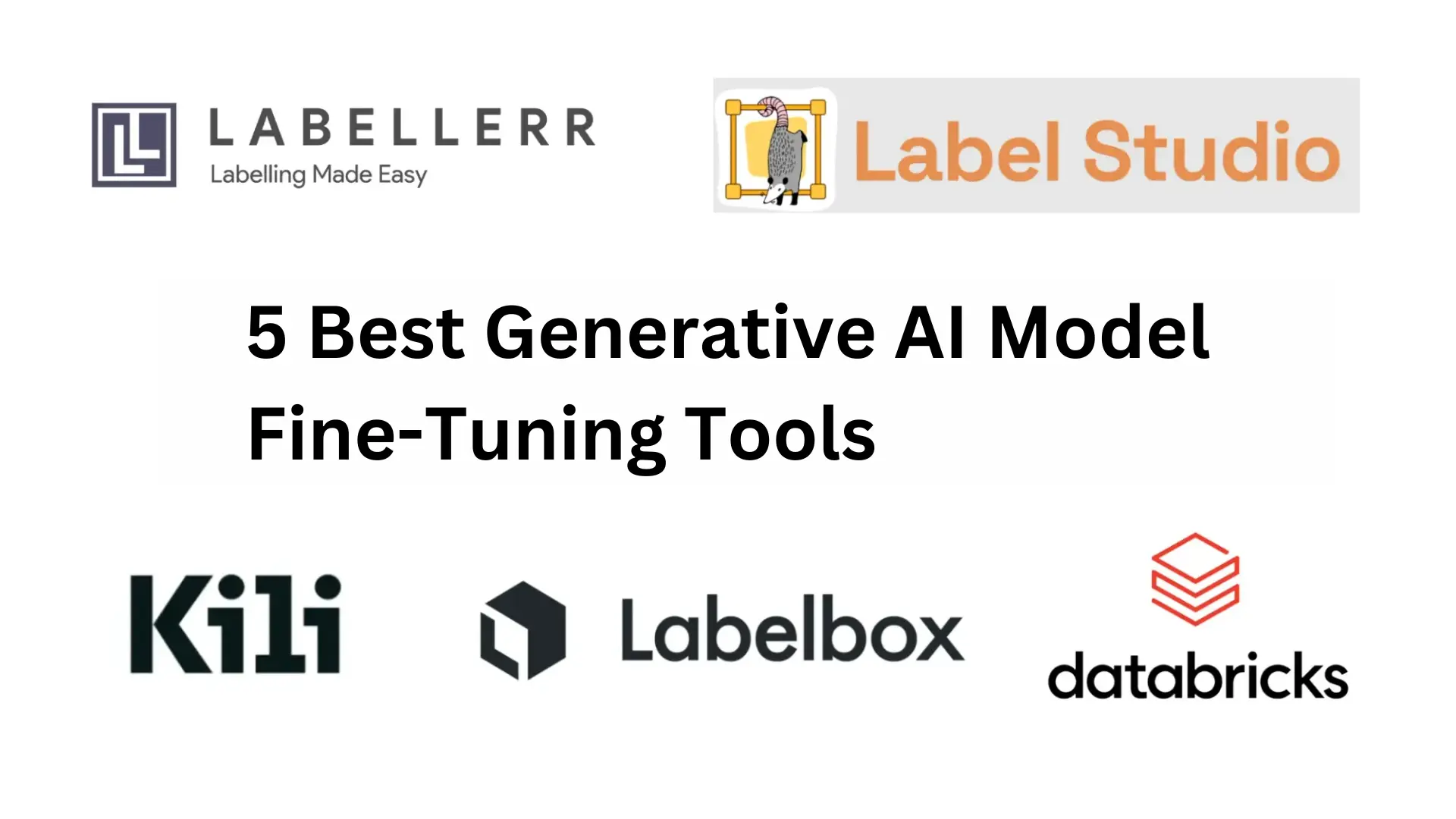
Introduction
Fine-tuning tools for Generative AI Models (GAMs) are pivotal in optimizing their performance across various natural language processing tasks.
Explore five top tools – Labellerr, Kili, Label Studio, Databricks Lakehouse, and Labelbox – each offering distinct features to elevate the fine-tuning process.
Read this extensive blog to learn about the features, benefits, and capabilities of these tools, whether you're a machine learning enthusiast or a professional trying to improve GAM performance.
Here's what we will explore:
1. Labellerr
Labellerr stands out as a cutting-edge platform precisely designed to speed up the fine-tuning process for Generative AI models.
Crafted with the specific needs of machine learning teams in mind, Labellerr expedites the preparation of high-quality training data for optimal fine-tuning outcomes in record time.
This platform boasts a myriad of features aimed at enhancing the efficiency and effectiveness of the fine-tuning journey, presenting a smart, intuitive, and rapid solution for ML teams.
Key Features
1. Customizable Workflow Configuration
Labellerr empowers users to design bespoke annotation tasks, ensuring alignment with the precise requirements of Generative AI fine-tuning, spanning tasks like text generation, sentiment analysis, and semantic understanding.
2. Versatile Data Format Support
Labellerr supports a wide array of data formats, encompassing text, images, audio, and video.
This versatility proves invaluable for Generative AI models engaged in multi-modal tasks that demand handling diverse data types.
3. Collaborative Annotation for Enhanced Productivity
Labellerr facilitates seamless collaboration among team members, particularly through its Enterprise version, fostering multiple annotators to work concurrently on the same dataset.
This collaborative feature streamlines annotation efforts distributes workload efficiently, and ensures coherence in annotations.
4. Robust Quality Control Mechanisms
Labellerr offers a suite of quality control tools, including annotation history tracking and disagreement analysis.
These tools play a pivotal role in upholding the accuracy and integrity of annotations, vital for achieving superior fine-tuning outcomes.
5. Integration with Machine Learning Models
Labellerr seamlessly integrates with machine learning models, enabling the implementation of active learning workflows.
This entails leveraging Generative AI models for pre-annotation, followed by human correction, thereby amplifying the efficiency of the annotation process and elevating fine-tuning results to new heights.
Generative AI Fine-Tuning Assistant positions itself as an all-encompassing platform empowering machine learning teams to efficiently curate high-quality datasets for the fine-tuning of Generative AI models.
With its user-centric design and emphasis on customization and collaboration, Labellerr delivers a potent solution for unlocking the full potential of Generative AI models in the dynamic realm of natural language processing and comprehension.
2. Kili
Kili emerges as a leader in the field of fine-tuning generative AI models, specifically tailored for Language Model Models (LLMs).
Its user-friendly platform addresses critical aspects of fine-tuning, including clear evaluation, high-quality data labeling, feedback conversion, seamless LLM integration, and expert annotator access.
Kili's strength lies in its ability to facilitate custom evaluation criteria, combining automated LLM assessments with human reviews for precise evaluation.
Key Features
1. Clear Evaluation for Effective Fine-Tuning
Custom Evaluation Criteria: Users can establish criteria such as following instructions, creativity, reasoning, and factuality.
Automated LLM Assessments: Kili combines automated assessments with human reviews for both scalability and precision.
2. High-Quality Data Labeling
Diverse Task Handling: Kili's platform covers a mix of tasks, including classification, ranking, transcription, and dialogue utterances.
Advanced QA Workflows: Users can set up advanced QA workflows, implement QA scripts, and detect errors in machine learning datasets.
3. Feedback Conversion for Actionable Insights
Advanced Filtering System: Kili overcomes noise and information scarcity in user feedback through an advanced filtering system.
Efficient Targeting: Users can swiftly identify significant conversations, converting user insights into actionable training data.
4. Seamless Integration with Leading LLMs
Native Copilot LLM-Powered System: Users can natively use a Copilot LLM-powered system for annotation.
Plug-and-Play Integrations: Kili offers plug-and-play integrations with market-leading LLMs like GPT, eliminating unnecessary 'glue' code.
5. Expert Annotator Access for Industry-Relevant Excellence
Qualified Data Labelers: Kili provides qualified annotators with industry-specific expertise.
Handpicked Labelers: Annotators are handpicked to ensure high-quality standards, delivering labeled datasets swiftly, often within days.
6. Positive User Testimonials
User-Friendly Interface: Testimonials highlight Kili's user-friendly platform and easy navigation.
Efficient Tools: Users praise the efficiency of Kili's tools for data labeling and LLM fine-tuning.
3. Labelbox
Labelbox's Generative AI Fine-Tuning Tool is a comprehensive solution designed to facilitate the fine-tuning process of Generative AI models.
Generative AI models leverage deep learning techniques for tasks such as text generation, analysis, and prediction, making them pivotal in various applications including natural language processing (NLP), creative writing, and content generation.
Labelbox's tool specifically targets the enhancement of Generative AI models by providing a structured framework for fine-tuning.
Key Features and Workflow
1. Customizable Ontology Setup
Define a relevant classification ontology aligned with your specific Generative AI use case, ensuring the model is finely tuned to understand and generate content specific to your domain.
2. Project Creation and Annotation in Labelbox Annotate
Create a project in Labelbox, matching the defined ontology for the data you want to generate with the Generative AI model.
Utilize Labelbox Annotate to generate labeled training data, allowing for efficient and accurate annotation.
3. Iterative Model Runs
Leverage iterative model runs to rapidly fine-tune the Generative AI model.
Labelbox supports the diagnosis of performance, identification of high-impact data, labeling of data, and creation of subsequent model runs for the next iteration of fine-tuning.
4. Google Colab Notebook Integration
The integration with Google Colab Notebook streamlines the process, allowing for the importation of necessary packages, including Labelbox, directly within the notebook.
API keys connect to instances seamlessly.
5. Adaptive Training Data Generation
The tool guides users in generating training data based on the defined ontology.
This step is crucial for adapting the Generative AI model to the specific use case, ensuring that the model captures nuances relevant to the targeted domain.
Cloud-Agnostic Platform: Labelbox's cloud-agnostic platform ensures compatibility with various model training environments and cloud service providers (CSPs).
The platform seamlessly connects to the model training environment, enhancing flexibility and accessibility.
Advantages
1. Time and Cost Efficiency
Leveraging a foundational model saves significant time and costs compared to training models from scratch.
2. Reinforcement Learning from Human Preferences (RLHP)
The tool provides a framework for incorporating RLHP, a key aspect in significantly improving the performance of Generative AI models.
3. Iterative Improvement
The iterative workflow empowers users to continuously fine-tune the Generative AI model based on real-world data priorities, ensuring ongoing improvement and adaptability.
4. Performance Evaluation
Labelbox Model allows users to measure the performance of the model, identify areas of weakness, and iteratively improve by feeding relevant data back into the fine-tuning process.
5. Catalog Integration for Priority Data
Utilizing Labelbox Catalog features, users can prioritize data that will have the highest impact on the next training iteration, enhancing the model's ability to address edge cases effectively.
Labelbox's Generative AI Fine-Tuning Tool combines flexibility, efficiency, and iterative improvement, providing a structured approach for optimizing Generative AI models in diverse applications.
The integration with Google Colab Notebook and the cloud-agnostic platform further enhances the user experience, making it a valuable resource for machine learning teams seeking to fine-tune Generative AI models for their specific use cases.
4. Label Studio
Label Studio's Generative AI Fine-Tuning Tool is a versatile and powerful platform specifically designed to enhance the process of fine-tuning Generative AI models.
This tool plays a crucial role in preparing the data essential for refining Generative AI models by offering a range of features tailored to the intricacies of model optimization.
With Label Studio, users can create customized annotation tasks, allowing for the precise labeling of data relevant to the specific requirements of Generative AI fine-tuning, including tasks such as text generation, content classification, and style adaptation.
Key Features
1. Custom Data Annotation Tasks
Tailored Annotation: Label Studio allows the creation of custom annotation tasks specific to fine-tuning Generative AI models, accommodating needs such as text generation, content classification, and style adaptation.
2. Versatile Multi-Format Support
Adaptability Across Data Types: Label Studio supports diverse data types, including text, images, audio, and video, catering to the multi-modal nature of Generative AI tasks involving different data formats.
3. Collaborative Annotation Capabilities
Enhanced Teamwork: Label Studio Enterprise facilitates collaborative annotation, streamlining the efforts of multiple annotators on the same dataset.
This collaborative feature aids in workload distribution and ensures consistency in annotations, crucial for preparing large datasets for Generative AI fine-tuning.
4. Quality Control Features
Ensuring Annotation Precision: Label Studio Enterprise provides tools for quality control, including annotation history and disagreement analysis.
These features contribute to maintaining the accuracy and quality of annotations, essential for the success of the fine-tuning process.
5. Seamless Integration with ML Models
Active Learning Workflows: Label Studio seamlessly integrates with machine learning models, enabling active learning workflows.
Leveraging Generative AI models to pre-annotate data, followed by human correction, enhances the efficiency of the annotation process and contributes to improved fine-tuning results.
Label Studio emerges as a powerful tool for optimizing the fine-tuning process of Generative AI models.
Through tailored annotation tasks, versatile support for multiple data formats, collaborative annotation features, robust quality control, and integration with machine learning models, Label Studio empowers users to efficiently prepare high-quality datasets, unlocking the full potential of Generative AI models.
5. Databricks Lakehouse
Databricks Lakehouse stands out as a comprehensive platform tailored for fine-tuning Generative AI models, prioritizing distributed training and real-time serving endpoints to optimize model performance.
Key Features
1. Ray AIR Integration
Utilizes Ray AIR Runtime for distributed fine-tuning of Generative AI models, enabling efficient scaling across multiple nodes.
Integration with Spark data frames and leveraging Hugging Face for data loading enhances the platform's versatility.
2. Model Tuning with RayTune
Allows for model hyperparameter tuning using RayTune, ensuring optimal performance for specific use cases through distributed fine-tuning.
3. MLFlow Integration for Model Tracking
Integrates with MLFlow for comprehensive model version tracking and logging, ensuring standardized storage format for models and their checkpoints.
4. Real-Time Model Endpoints
Enables deployment of Generative AI models with real-time serving endpoints on Databricks, supporting both CPU and GPU serving options.
Upcoming features include optimized serving for large Generative AI models.
5. Efficient Batch Scoring with Ray
Demonstrates efficient batch scoring using Ray BatchPredictor, ideal for distributing scoring tasks across instances with GPUs.
6. Low-Latency Model Serving Endpoints
Introduces Databricks Model Serving Endpoints, offering low-latency and managed services for Generative AI deployments.
GPU serving options are available, with upcoming features focusing on optimized serving for large models.
Advantages
1. Unified Framework
Ray AIR serves as a unifying framework, seamlessly orchestrating various tools like Spark, Hugging Face, and MLFlow, streamlining the fine-tuning process.
2. Distributed Scalability
Leverages distributed computing capabilities for both training and inference, ensuring efficient scaling of Generative AI models across clusters.
3. Flexible Model Tuning
Provides flexibility in model hyperparameter tuning through RayTune, allowing adaptation to specific performance requirements.
4. MLFlow for Model Management
MLFlow integration enhances model versioning, tracking, and logging, facilitating comprehensive model management.
5. Real-Time Serving
Real-time serving endpoints enable immediate deployment of fine-tuned Generative AI models in applications, reducing latency and enhancing user experience.
6. Support for Large Models:
Addresses challenges related to GPU memory constraints, offering solutions and recommendations for optimizing resources to accommodate large Generative AI models.
Databricks Lakehouse emerges as a versatile platform for fine-tuning Generative AI models, offering a range of features and advantages tailored to meet the evolving needs of machine learning teams in optimizing models for various applications.
Read some of our other listicles:
Conclusion
Generative AI model fine-tuning is rapidly evolving, with innovative platforms like Labellerr, Kili, Label Studio, and Databricks Lakehouse leading the charge toward more efficient and effective processes.
Labellerr and Kili offer tailored solutions with customizable annotation tasks, versatile data format support, collaborative annotation capabilities, and robust quality control mechanisms, catering to the specific needs of machine learning teams.
Label Studio and Databricks Lakehouse provide comprehensive frameworks for fine-tuning Generative AI models, emphasizing flexibility, scalability, integration with machine learning models, and real-time serving capabilities.
As the demand for high-quality datasets and optimized models continues to grow, these platforms stand as invaluable tools empowering users to unlock the full potential of Generative AI in diverse applications, from natural language processing to creative writing and content generation.
Frequently Asked Questions
1. What are the benefits of fine-tuning a pre-trained model for generative AI?
Fine-tuning a pre-trained model for generative AI offers several benefits.
Firstly, it accelerates the training process by leveraging the knowledge encoded in the pre-trained model, reducing the need for extensive training data and computation resources.
Secondly, fine-tuning allows the model to adapt to specific tasks or domains, enhancing its performance and accuracy in generating content relevant to the targeted application.
Additionally, fine-tuning enables customization of the model's output, allowing users to control aspects such as style, tone, and content specificity, thereby tailoring the model to meet diverse requirements.
Overall, fine-tuning pre-trained models for generative AI empowers users to achieve superior performance and flexibility in various natural language processing tasks.
2. What are generative AI learning methods?
Generative AI learning methods encompass a range of techniques aimed at training models to generate novel content, such as text, images, audio, and more.
These methods often include approaches like autoregressive models, where the model predicts the next token in a sequence based on previous tokens; variational autoencoders (VAEs), which learn a latent representation of data and generate new samples by sampling from this latent space; and generative adversarial networks (GANs), which consist of two neural networks, a generator and a discriminator, trained adversarially to produce realistic samples and distinguish between real and generated data.
Each method has its strengths and applications, contributing to the diverse landscape of generative AI learning.

Simplify Your Data Annotation Workflow With Proven Strategies
.png)
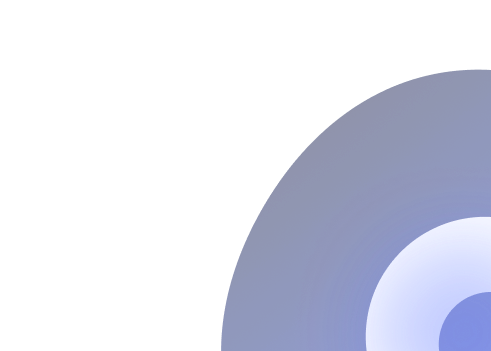