AI Models: Introduction, Uses, and Types
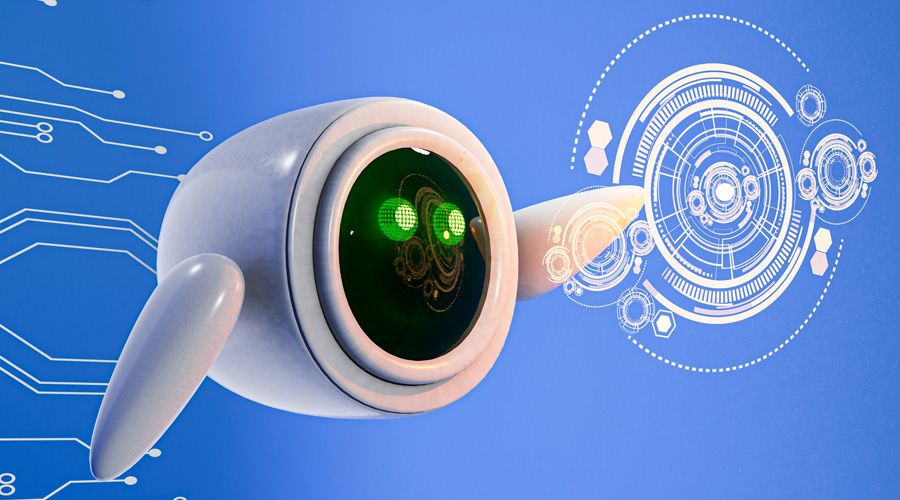
AI models are gradually becoming more valuable in practically every industry due to their versatility in problem-solving. An AI model is, in the most straightforward words, a software or algorithm that uses a specific data set to enable it to conclude without the involvement of a human decision-maker.
The artificial intelligence (AI) model uses machine learning techniques as well as artificial neural networks to simulate logical decision-making utilizing the resources and input data sets that are readily available.
These models are the foundation of contemporary intelligence technologies and are adept at information analysis, generating judgments and predictions, and offering insightful information.
Let's explore the AI model and discover what they are. And also, take a look at some of the popular AI models currently available in the market.
Table of Contents
- What is an AI Model?
- The Importance of AI Models
- Common Uses of AI Models
- Most Popular AI Models
- Choosing the Right AI Model for Your Needs
- Conclusion
- FAQs
What is an AI Model?
A branch of computer science that is artificial intelligence, or AI, focuses on creating machines that can mimic human intelligence.
A program or algorithm that uses a set of data to recognize particular patterns is known as an artificial intelligence (AI) model. This enables it to draw a judgment or make a forecast when given enough data.
To identify various patterns, AI models rely on machine learning, Natural language processing (NLP), and computer vision. Decision-making algorithms are another way that AI models understand from their training, gather and analyze data, and finally use what they've learned to accomplish their predetermined goals.
With the use of one or more algorithms, AI models attempt to grasp the link between several inputs of various types to anticipate outcomes or make judgments.
The Importance of AI Models
AI models are computer programs or algorithms that use data to identify patterns and form hypotheses or judgments. They are created to emulate human thought processes, enabling computers to carry out certain of our activities more precisely and effectively. AI models may be used for a variety of tasks, including natural language processing and picture identification.
Some of the major advantages of AI models are as follows:
Efficiency: AI models have the ability to dependably and without becoming tired conduct frequent, high-volume, computerized activities that are repetitive. Businesses may become more effective and spend less money as a result of this.
Accuracy: AI algorithms are able to analyze vast volumes of data and spot patterns that people would overlook, making predictions and judgments more accurate.
Complex Problem-Solving Capability: AI models are particularly effective at tackling problems that call for extensive data processing.
Reduced Biases: AI/ML model evaluations can spot chances to improve model performance and minimize biases in current and next iterations.
Overall, by enabling organizations to make better decisions based on data analysis, AI models may help firms become more effective, competitive, and lucrative. As more businesses embrace AI models to gain a competitive edge, they are expected to become increasingly more significant in the business world in the future.
Common Uses of AI Models
Artificial intelligence (AI) models are utilized in a variety of fields to streamline operations, automate procedures, and resolve challenging issues. Here are a few typical applications for AI models:
- Image Recognition: AI models can be used to identify and categorize photos, which has uses in e-commerce, security, and the healthcare industries.
- Natural Language Processing (NLP): AI models may be used to decipher and comprehend human language, which has uses in sentiment analysis, chatbots, and virtual assistants.
- Recommender systems: Systems that make product, service, or content recommendations to users based on their interests and behavior are known as recommender systems.
- Generative AI: AI models can be used to create results in situations when there isn't a single correct response, such as writing creatively, creating marketing material, or creating software code.
- Personalization: AI models may be used to provide customized user experiences, such as customized purchasing recommendations, customized lesson plans for students, and customized marketing material.
- Predictive analytics: Using AI models, data can be analyzed to create predictions about the future, which has applications in industries including finance, healthcare, and marketing.
- Fraud detection: Artificial intelligence (AI) models may be used to identify fraudulent behavior, including identity theft, insurance fraud, and credit card fraud.
- Autonomous Vehicles: AI models may be used to create self-driving cars, which have uses in logistics and transportation.
- Chatbots: AI models can be used to fuel chatbots, which can answer frequent questions, provide customer care, and enhance conversational commerce capabilities.
- Control of the Manufacturing Process: AI models can be used to streamline production procedures and enhance quality assurance, which has applications in the automobile, welding, and semiconductor industries.
These are just a handful of the numerous uses for AI models. We can anticipate witnessing even more cutting-edge applications of AI as the field develops.
Most popular AI models
There are numerous AI models, and each one functions slightly differently. An AI model collection may contain a number of the most well-known models, including:
1. Deep neural networks
Undoubtedly one of the most well-liked AI/ML models is the deep neural network. This deep learning model structure was influenced by the neural network of the human brain. This AI model combines many inputs to produce a single output value using layers of artificial neurons. The most advanced artificial intelligence model is this one. It excels at resolving intricate issues with massive data sets.
The development of mobile apps frequently makes use of deep neural networks to offer audio, image, and image recognition services, as well as to process natural language.
2. Linear regression
The linear regression model, which is centered on supervised learning, is widely used in statistics. Finding correlations between the input and result variables is the main goal of this model.
A linear regression model can forecast the value of a dependent variable depending on the value of an independent variable. In the fields of healthcare, insurance, commerce, and finance, these models are employed in linear discriminant analysis.
3. Logistic regression
Another well-liked AI model that is closely similar to the linear regression model is this one. It is a statistical method that incorporates the set of available independent factors to forecast the dependent variable's class.
However, because it can only be used to address classification-based issues, the logistic regression differentiates from a linear regression model. If your aim is handling binary classification issues, then logistic regression is the best option.
4. Decision trees
The decision Tree model is simple and incredibly effective. The decision tree decides by using information from previous decisions that are currently available. These trees frequently use an if/then structure.
Both classification and regression issues can be resolved using decision trees. Additionally, the earliest types of predictive analytics were powered by simple decision trees.
5. Naive Bayes
This AI Model is a straightforward yet powerful AI model that can be used to address a variety of challenging issues. It is particularly used for test classification and is dependent on the Bayes Theorem.
The model operates under the presumption that the existence of one feature does not entail the existence of any additional feature. The model is referred to as "naive" because this presumption is virtually never accurate.
Both binary, as well as multiple-class classifications, can be performed with it. Spam filtering and the classification of medical data are some of its uses.
6. Random forest
How powerful would an entire forest be if a sole decision tree is a potent AI model? A random forest is made up of many different decision trees. Each decision tree delivers its outcome, which is combined with those of all the other trees in the forest. Finally, the combined findings produce a more precise conclusion, forecast, or choice.
When you have a big amount of data, then the random forest is an excellent AI model. Both regression, as well as classification issues, are addressed by this model. Random forest models play a significant role in the capacity of contemporary predictive analytics.
7. Support vector machines
Support Vector Machine, or SVM, is a rapid and effective model that performs exceptionally well when processing small amounts of data. It works for issues involving binary classification. SVM is quicker and more effective with a dataset of small samples than more recent technologies like artificial neural networks, such as those used to solve text categorization problems.
This supervised machine learning approach applies to classification, feature extraction, and regression issues.
8. K-Nearest neighbors
The k-nearest neighbor's algorithm, also referred to as KNN or k-NN, is a supervised learning classifier that employs proximity to producing classifications or forecasts about the gathering of a single data point. Although it can be applied to classification or regression issues, this method operates under the presumption that related things (data) are located close to one another.
Supervised Learning Models
Supervised learning models are trained using labeled datasets, which means that each data point has characteristics and a corresponding label. Finding a mapping function that converts the input variable into the output variable is the goal of supervised learning. The supervisor that teaches the machine to accurately forecast the output is the training data that is given to the machine learning model. Numerous applications, including risk assessment, image classification, fraud detection, and spam filtering, can benefit from supervised learning.
Unsupervised Learning Models
In Unsupervised learning models, models are not supervised by training data. Instead, models analyze the supplied data to uncover any hidden patterns and insights. Clustering, association, and dimensionality reduction are the three basic tasks that unsupervised learning models are used for. In comparison to supervised learning, unsupervised learning algorithms enable users to carry out more complicated processing tasks.
Compared to other natural learning processes, unsupervised learning, however, might be more unpredictable. Algorithms for unsupervised learning include neural networks, clustering, and anomaly detection. The most popular unsupervised learning techniques include dimensionality reduction, association rule learning, and clustering.
Reinforcement Learning Models
The reinforcement Learning model enables an agent to learn in an interactive environment through trial and error while using feedback from its own actions and experiences. In RL, an agent's behavior should select activities that have a tendency to raise the long-term sum of values of the reinforcement signal. An agent is connected to its environment through perception and action.
Model-free reinforcement learning has two major methods for representing agents: Q-learning and Policy optimization. A Markov decision process (MDP), which is a collection of environment and agent states, actions, and probabilities of transition from state to state under the action, is how reinforcement learning methods are modeled.
Choosing the Right AI Model for Your Needs
Given the variety of models available, each with unique strengths and drawbacks, choosing the best AI model for your purposes can be difficult.
Here are some tips to assist you in selecting the ideal AI model for your business issue:
- Describe your Issue: You must identify your issue and comprehend your goals before selecting an AI model. This will enable you to focus your search and select the model that best suits your requirements.
- Think about Your Data: Your choice of AI model will also be influenced by the kind and quantity of data you have. There are models that work better with structured data and others that work better with unstructured data.
- Analyze the Performance: Prior to selecting a particular AI model, it is crucial to assess the performance of each one. This may be accomplished by comparing the models' accuracy, precision, recall, and other parameters after testing them on your data.
- Understand the Model's Limitations: Before selecting an AI model, it's critical to be aware of any restrictions it may have. For instance, certain models might not be appropriate for real-time applications, while others might struggle with huge amounts of data.
- Consult a Professional: It's a good idea to get professional help if you're unclear about which AI model to select. This might be from a data scientist, an AI service provider, or an AI consultant.
Overall, your business case and requirements will determine which AI model to choose. The quantity, variety, and pace of your data, the kind of issue you're attempting to resolve, as well as the capabilities and restrictions of various AI models, are some important aspects that you should consider.
Conclusion
In the end, it can be concluded that if you want to get the best results from data and make effective decisions, then it is important to know about AI models and implement them in your usage.
AI models are very effective for problem-solving and based on the requirement in the use cases, you can get better results. These models will undoubtedly be used in a much wider range of industries soon, including computer vision, medicine, commerce, finance, banking, etc.
Our platform automates labeling for images, text, and more, saving you time and money. Try our free auto-labeling tool now and start building better AI!
If you are looking for an option to implement an AI model into your field and are stuck training your data, then don’t worry! Labellerr is here to help you. We are highly skilled at providing training data for a variety of use cases with various domain authorities.
To know more about such related information, stay tuned!
FAQs
1.What are the different types of AI models?
Classification models, regression models, clustering models, reinforcement learning models, and deep learning models are among the several categories of AI models. Each sort of model has unique traits and is appropriate for various activities and applications.
2. How are AI models used in real-world applications?
In several real-world applications across numerous sectors, AI models are widely employed. They are used in a variety of industries, including healthcare for the purpose of illness diagnosis and treatment planning, finance for the purpose of fraud detection and risk assessment, autonomous cars for the purpose of object identification and navigation, customer service for chatbots, and many more. Automation, optimization, and intelligent decision-making are made possible by AI models, which enhance efficiency and results.
3. What are some examples of unsupervised learning models?
Algorithms for grouping data, such as K-means, hierarchical clustering, and DBSCAN, are examples of unsupervised learning models. These models are intended to find structures and patterns in datasets that don't have clear labels or established groups. They are helpful for activities like data exploration, anomaly detection, and consumer segmentation.
4. What industries benefit the most from AI models?
AI models are utilized in various industries. Healthcare is one of the most notable instances, where AI models help with medication discovery and medical image interpretation. For individualized suggestions and demand predictions, e-commerce uses AI algorithms. AI models are used in manufacturing for quality assurance and proactive maintenance. Other areas that gain a lot from AI models include agriculture, banking, transportation, cybersecurity, marketing, and others.
5. Are there any limitations or challenges associated with AI models?
AI models have amazing potential, but they also have constraints and difficulties. One difficulty is the requirement for substantial volumes of high-quality data for accurate model training. Significant problems also include ethical issues and biases in data and model results. Furthermore, it continues to be difficult to analyze and explain AI models, particularly in sophisticated deep-learning structures. Additional restrictions that must be addressed include malicious assaults and cybersecurity risks. It's critical to strike the correct balance between innovation and ethical usage of AI models.

Simplify Your Data Annotation Workflow With Proven Strategies
.png)
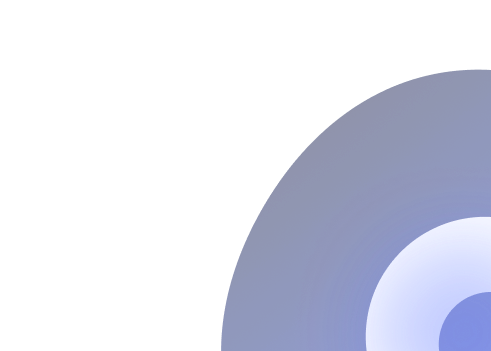