5 Best Tools for LLM Fine-Tuning in 2025
In 2025, top tools for fine-tuning Large Language Models (LLMs) include Labellerr, Kili, Label Studio, Labelbox, and Databricks Lakehouse. These platforms offer customizable workflows, high-quality data labeling, collaboration, and integration.
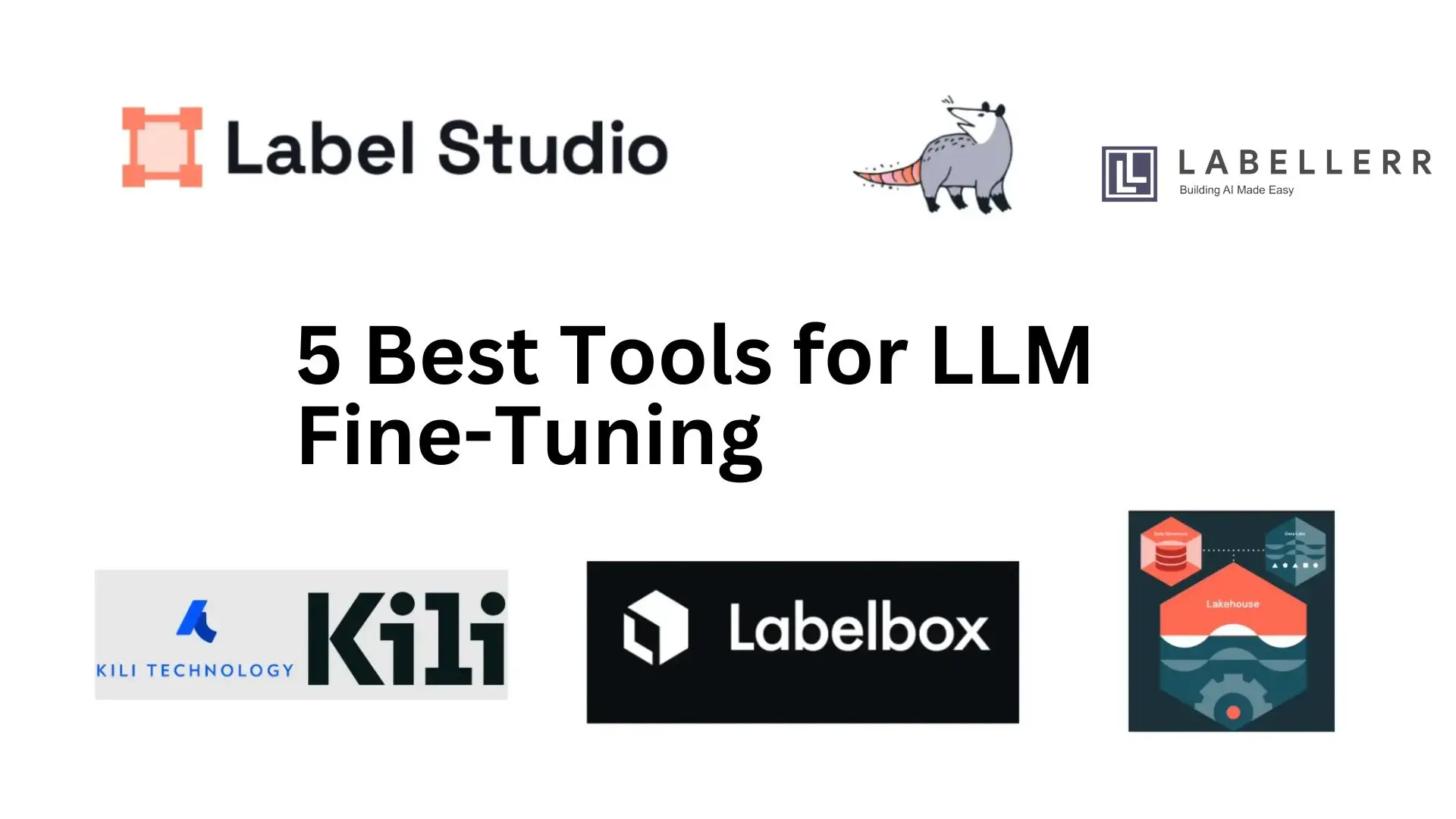
Finding the right tools to fine-tune Large Language Models (LLMs) can be a daunting task, especially with the growing number of options in 2025.
If you’ve tried customizing an LLM for your specific needs, you already know the struggle: compatibility issues, high costs, and complex setups can turn a straightforward goal into a time-consuming challenge.
The good news?
The right tools can make all the difference.
Fine-tuning not only enhances an LLM’s accuracy for specialized tasks but can also increase your model’s efficiency and reduce operational costs—two reasons why most AI-driven businesses now prioritize fine-tuning as part of their strategy.
In this post, we’ll explore the top five fine-tuning tools for LLMs in 2025. Each tool comes with its own strengths, suited to various levels of expertise, budgets, and use cases.
By the end, you’ll know exactly which option aligns best with your goals and how to start using it for optimal results.
Table of Contents
- Labellerr
- Killi
- Label Studio
- Labelbox
- Databricks Lakehouse
- Amazon SageMaker Ground Truth
- Dataloop
- Conclusion
- FAQ
1. Labellerr
Labellerr's LLM Fine-Tuning Tool emerges as an advanced and efficient platform, specifically designed to assist machine learning teams in setting up the Large Language Model (LLM) fine-tuning process seamlessly.
With Labellerr's versatile and customizable workflow, ML teams can expedite the preparation of high-quality training data for LLM fine-tuning in a matter of hours.
The tool offers a range of features to optimize the fine-tuning process, making it a smart, simple, and fast solution.
Key Features
- Custom Workflow Setup
Tailored Annotation Tasks: Labellerr enables the creation of custom annotation tasks, ensuring the labeling process aligns with the specific requirements of LLM fine-tuning, such as text classification, named entity recognition, and semantic text similarity.
- Multi-Format Support
Versatility Across Data Types: Labellerr supports various data types, including text, images, audio, and video.
This adaptability is particularly valuable for LLMs engaged in multi-modal tasks involving different data types.
- Collaborative Annotation for Efficiency
Team Collaboration: Labellerr's collaborative annotation capabilities, especially in the Enterprise version, facilitate multiple annotators working on the same dataset.
This collaborative feature streamlines the annotation process, distributes the workload, and ensures annotation consistency.
- Quality Control Tools
Ensuring Annotation Precision: Labellerr provides features for quality control, including annotation history and disagreement analysis.
These tools contribute to maintaining the accuracy and quality of annotations, crucial for the success of the LLM fine-tuning process.
- Integration with Machine Learning Models
Active Learning Workflows: Labellerr can be seamlessly integrated with machine learning models, allowing for active learning workflows.
This means leveraging LLMs for pre-annotation, followed by human correction, enhancing the efficiency of the annotation process, and improving fine-tuning results.
- Pricing:
Pro Plan: Starts at $499 per month for 10-user access with 50,000 data credits included. Additional data credits can be purchased at $0.01 USD per data credit, and extra users can be subscribed to at $29 USD per user.
Enterprise Plan: Offers professional services, including tool customization and ML consultancy.
- G2 Review
2. Kili
Kili's LLM Fine-Tuning Tool is a comprehensive and user-friendly solution for individuals and enterprises seeking to enhance the performance of their Language Model Models (LLMs).
The platform offers a one-stop-shop experience, addressing five crucial elements for successful fine-tuning: clear evaluation, high-quality data labeling, feedback conversion, seamless LLM integration, and expert annotator access.
Key Features
- Clear Evaluation for Effective Fine-Tuning
Custom Evaluation Criteria: Users can establish criteria such as following instructions, creativity, reasoning, and factuality.
Automated LLM Assessments: Kili combines automated assessments with human reviews for both scalability and precision.
- High-Quality Data Labeling
Diverse Task Handling: Kili's platform covers various tasks, including classification, ranking, transcription, and dialogue utterances.
Advanced QA Workflows: Users can set up advanced QA workflows, implement QA scripts, and detect errors in machine learning datasets.
- Feedback Conversion for Actionable Insights
Advanced Filtering System: Through an advanced filtering system, Kili overcomes noise and information scarcity in user feedback.
Efficient Targeting: Users can swiftly identify significant conversations, converting user insights into actionable training data.
- Seamless Integration with Leading LLMs
Native Copilot LLM-Powered System: Users can natively use a Copilot LLM-powered system for annotation.
Plug-and-Play Integrations: Kili offers plug-and-play integrations with market-leading LLMs like GPT, eliminating unnecessary 'glue' code.
- Expert Annotator Access for Industry-Relevant Excellence
Qualified Data Labelers: Kili provides qualified annotators with industry-specific expertise.
Handpicked Labelers: Annotators are handpicked to ensure high-quality standards, delivering labeled datasets swiftly, often within days.
- Pricing
- Free Plan: $0/month for individual contributors with up to 100 annotations.
- Grow Plan: Custom pricing for companies, includes features like zero-shot labeling co-pilot, instant quality review scores, and more.
- Enterprise Plan: Custom pricing for large companies, includes feature like SSO, on-premise hosting, custom contracts, and dedicated customer success support.
- Positive User Testimonials
User-Friendly Interface: Testimonials highlight Kili's user-friendly platform and easy navigation.
Efficient Tools: Users praise the efficiency of Kili's tools for data labeling and LLM fine-tuning.
3. Label Studio
Label Studio's LLM Fine-Tuning Tool is a versatile and powerful data annotation platform specifically designed to enhance the process of fine-tuning Large Language Models (LLMs).
This tool plays a crucial role in preparing the data essential for refining LLMs by offering a range of features tailored to the intricacies of language model optimization.
Key Features
- Custom Data Annotation Tasks:
Tailored Annotation: Label Studio allows the creation of custom annotation tasks specific to fine-tuning LLMs, accommodating needs such as text classification, named entity recognition, or semantic text similarity.
- Versatile Multi-Format Support:
Adaptability Across Data Types: Label Studio supports diverse data types, including text, images, audio, and video, catering to the multi-modal nature of LLM tasks involving different data formats.
- Collaborative Annotation Capabilities:
Enhanced Teamwork: Label Studio Enterprise facilitates collaborative annotation, streamlining the efforts of multiple annotators on the same dataset.
This collaborative feature aids in workload distribution and ensures consistency in annotations, crucial for preparing large datasets for LLM fine-tuning.
- Quality Control Features:
Ensuring Annotation Precision: Label Studio Enterprise provides tools for quality control, including annotation history and disagreement analysis.
These features contribute to maintaining the accuracy and quality of annotations, essential for the success of the fine-tuning process.
- Seamless Integration with ML Models:
Active Learning Workflows: Label Studio seamlessly integrates with machine learning models, enabling active learning workflows.
Leveraging LLMs to pre-annotate data, followed by human correction, enhances the efficiency of the annotation process and contributes to improved fine-tuning results.
Label Studio emerges as a powerful tool for optimizing the fine-tuning process of Large Language Models.
Through tailored annotation tasks, versatile support for multiple data formats, collaborative annotation features, robust quality control, and integration with machine learning models, Label Studio empowers users to efficiently prepare high-quality datasets, unlocking the full potential of LLMs.
- Pricing
- Community Edition: Free, open-source, self-hosted.
- Starter Cloud: Starts at $99/month with a free trial includes 1000 annotation credits with fully managed cloud service. Additional users cost $50/month.
- Enterprise: Custom pricing, includes advanced features like single sign-on, enhanced quality workflows, and dedicated support.
- Capterra Review:
4. Labelbox
Labelbox's LLM Fine-Tuning Tool is a comprehensive solution designed to facilitate the fine-tuning process of Large Language Models (LLMs).
LLMs leverage deep learning techniques for tasks such as text recognition, classification, analysis, generation, and prediction, making them pivotal in natural language processing (NLP) applications like AI voice assistants, chatbots, translation, and sentiment analysis.
Labelbox's tool specifically targets the enhancement of LLMs by providing a structured framework for fine-tuning.
Key Features
- Customizable Ontology Setup
Define your relevant classification ontology that is aligned with your specific LLM use case, ensuring the model is tuned to understand and generate content specific to your domain.
- Project Creation and Annotation in Labelbox Annotate
Create a project in Labelbox, that matches the defined ontology for the data you want to classify with the LLM.
Utilize Labelbox Annotate to generate labeled training data, allowing for efficient and accurate annotation.
- Iterative Model Runs
Leverage iterative model runs to rapidly fine-tune OpenAI's GPT-3 model. Labelbox supports the diagnosis of performance, identification of high-impact data, labeling of data, and creation of subsequent model runs for the next iteration of fine-tuning.
- Google Colab Notebook Integration
The integration with Google Colab Notebook streamlines the process, allowing for the importing of necessary packages, including Open AI and Labelbox, directly within the notebook.
API keys connect to instances seamlessly.
- Adaptive Training Data Generation
The tool guides users in generating training data based on the defined ontology.
This step is crucial for adapting GPT-3 to the specific use case, ensuring that the model captures nuances relevant to the targeted domain.
- Cloud-Agnostic Platform
Labelbox's cloud-agnostic platform ensures compatibility with various model training environments and cloud service providers (CSPs).
The platform seamlessly connects to the model training environment, enhancing flexibility and accessibility.
- Pricing
- Free Plan: Free for individuals or small teams, includes essential labeling platform and data curation with natural language search.
- Starter Plan: Starts at $0.10 per Labelbox Unit (LBU), includes unlimited users, projects, and ontologies, custom labeling workflows, and project performance dashboard.
- Enterprise Plan: Custom pricing, includes advanced features like enterprise dashboard, advanced labeling analytics, SSO, and dedicated technical support.
- G2 Review
5. Databricks Lakehouse
Databricks Lakehouse serves as a comprehensive platform for fine-tuning LLMs, with a focus on distributed training and real-time serving endpoints.
The Lakehouse platform combines data processing and machine learning tools, making it easier to train and deploy LLMs efficiently. It works well with tools like Ray AI Runtime (AIR) and Hugging Face, simplifying the fine-tuning process.
Key Features
1. Ray AIR Integration
Utilizes Ray AIR Runtime for distributed fine-tuning of LLMs, enabling efficient scaling across multiple nodes.
Offers integration with Spark data frames and leverages Hugging Face for data loading.
2. Model Tuning with RayTune
Allows for model hyperparameter tuning using RayTune, an integrated hyperparameter tuning tool within the Ray framework.
Distributed fine-tuning ensures optimal model performance for specific use cases.
3. MLFlow Integration for Model Tracking
It integrates with MLFlow for tracking and logging model versions.
MLFlow's Transformer Flavor is utilized, providing a standardized format for storing models and their checkpoints.
4. Real-Time Model Endpoints
Enables the deployment of LLMs with real-time serving endpoints on Databricks.
Supports both CPU and GPU serving options, with upcoming features for optimized serving of large LLMs.
5. Efficient Batch Scoring with Ray
Demonstrates efficient batch scoring using Ray BatchPredictor, suitable for distributing scoring across instances with GPUs.
6. Low-Latency Model Serving Endpoints:
Databricks Model Serving Endpoints are introduced, offering low-latency and managed services for ML deployments.
GPU serving options are available, with upcoming features like optimized serving for large LLMs.
- Pricing
- Pay-as-you-go: No upfront costs, pay for what you use at per second granularity.
- Committed Use Contracts: Access discounts and benefits by committing to certain usage levels.
- Pricing Tiers: Different tiers like Data Engineering Light, Standard, Premium, Enterprise, and Data Analytics, starting at $0.07 per DBU.
- Azure Databricks: Pricing varies based on compute resources, with options for SQL Compute Clusters and Standard tier features.
Conclusion
Fine-tuning Large Language Models (LLMs) can be a complex and demanding process, but the right tools can significantly simplify and enhance your efforts.
The tools we've explored—Labellerr, Kili, Label Studio, Labelbox, and Databricks Lakehouse—each offer unique features and strengths that cater to different needs in the fine-tuning journey.
Take the time to go through each tool and consider what aligns best with your specific requirements.
Whether you need customizable workflows, high-quality data labeling, or seamless integration with existing systems, there’s a solution that fits your needs.
By choosing the right tool, you can unlock the full potential of your LLMs and drive better results in your natural language processing projects.
Read our other listicles:
Frequently Asked Questions
1. What is LLM fine-tuning?
LLM fine-tuning is a process that improves the performance of a pre-trained language model (LLM) for specific tasks or domains. It involves retraining the model on a new dataset to update its parameters and make it more effective.
2. How to fine-tune large language models (LLMs)?
Large Language Model (LLM) fine-tuning involves adapting the pre-trained model to specific tasks. This process takes place by updating parameters on a new dataset. Specifically, the LLM is partially retrained using <input, output> pairs of representative examples of the desired behavior.
3. Do you need memory for LLM fine-tuning?
Yes, memory is an important consideration when fine-tuning Large Language Models (LLMs).

Simplify Your Data Annotation Workflow With Proven Strategies
.png)
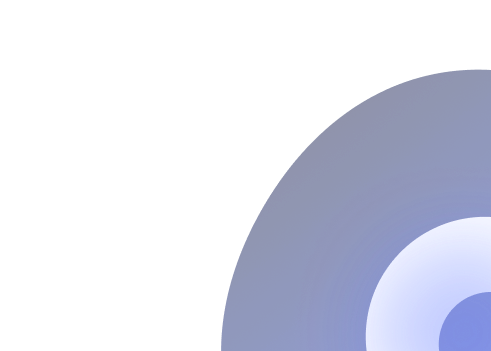