Generate Faster Annotations For Sports Analysis With Labellerr Using SAM
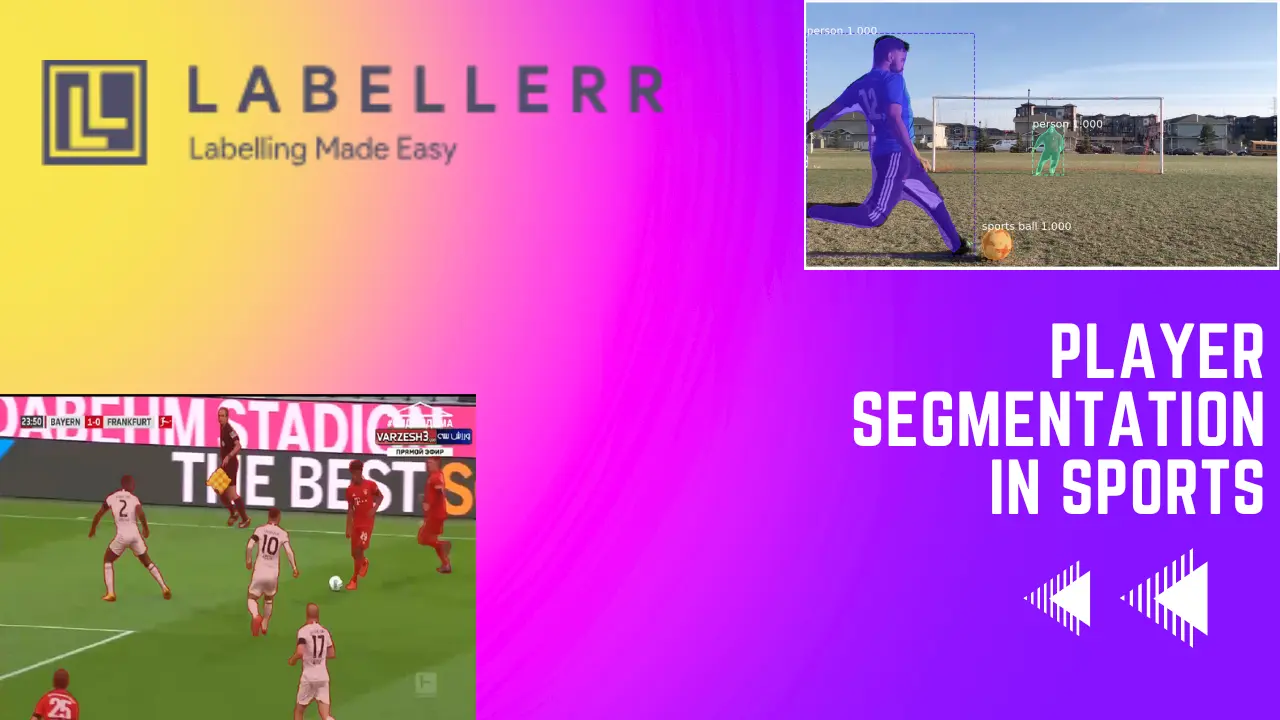
Table of Contents
- Introduction
- Challenge
- Solution Using Labellerr for Player Segmentation in the Sports Industry
- Conclusion
- Frequently Asked Questions
Introduction
In sports industry, the ability to analyze player movements and interactions plays a crucial role in understanding game strategies, enhancing player performance, and providing valuable insights for coaches and analysts.
With computer vision technology, particularly in sports like football, the possibilities for in-depth player segmentation have increased exponentially.
By using advanced algorithms and image processing techniques, computer vision systems can accurately identify and track individual players on the field, allowing for detailed analysis of their actions, positions, and interactions during gameplay.
However, to achieve accurate player segmentation, the availability of annotated datasets is important. You can also explore other uses of AI in sports in this blog.
Labellerr, a data annotation platform, offers solutions for annotating images and videos in the sports industry, enabling the creation of high-quality labeled datasets essential for training precise player segmentation models.
Challenge
The task of player segmentation in the sports industry, particularly in dynamic sports like football, presents lots of challenges.
Difficulties in Player Movement:
Frequent Changes: Players frequently shift positions, making real-time tracking and segmentation challenging.
Sudden Movements: Quick activities, such as jumps and tackles, might blur images, reducing segmentation accuracy.
Players can frequently obstruct each other's vision, necessitating complex occlusion handling solutions.
Annotators may use motion prediction models that train on past data to forecast player movement and potential occlusions.
Or annotators may use object tracking Techniques to track players between frames, even when they make abrupt movements.
Variations in image data:
Lighting Conditions: Insufficient lighting can cast shadows and highlights, distorting player segmentation.
Camera Angles: Varying camera angles affect player proportions, needing viewpoint-invariant techniques.
Image Quality: Grainy or low-resolution images produce unclear details, which reduces segmentation precision.
Annotators can use image normalization to adjust for variation in lighting and camera angles, resulting in consistent image data for segmentation.
Data Volume and Processing Constraints:
Large Datasets: Football matches produce massive volumes of video data, necessitating effective annotation systems.
Real-Time Analysis: For rapid insights, segmentation must be fast enough for near-real-time processing.
Scalability: Techniques must be scalable to accommodate enormous datasets and future demands.
Annotators can implement active learning by having the model identify uncertain player areas in frames and prioritize them for annotation. This directs human effort toward the most critical data points.
Manual Annotation Challenges:
Time-consuming: Manually segmenting every player in every frame is extremely labor-intensive.
Accuracy Limitations: Achieving perfect precision with every click can be challenging and subjective.
Increased Difficulty with numerous Players: Having numerous players significantly increases annotating time.
Annotators can use distributed processing frameworks: Use distributed processing frameworks to parallelize the annotation process across numerous machines, allowing you to efficiently handle large datasets.
Achieving accurate player segmentation requires advanced annotation methodologies and tools capable of addressing these challenges while maintaining the precision and reliability of the annotated datasets.
Solution Using Labellerr for Player Segmentation in the Sports Industry
To address these challenges, companies and researchers employ annotation platforms like Labellerr to streamline the annotation process.
By harnessing Labellerr's capabilities, they can annotate data with increased speed and accuracy, thereby doubling their release velocity for developing AI-powered image analysis software.
To see Labellerr in action for player segmentation in sports, check out this video
Read the full documentation for using SAM in an annotation workflow with Labellerr.
Below we discuss multiple factors by which Labellerr helps teams to overcome challenges.
Intuitive Interface
Labellerr offers an intuitive interface designed to streamline the annotation process for player segmentation in the sports industry.
With user-friendly navigation tools and clear labeling options, annotators can easily identify and annotate individual players within images and videos of football matches.
The intuitive interface minimizes the learning curve, allowing annotators to focus on the task at hand without being hindered by complex annotation tools.
Cost Savings
Labellerr facilitates significant cost savings for sports organizations and analysts by optimizing the annotation process and reducing manual labor costs.
Its advanced automation features automate repetitive tasks, such as image and video uploading and annotation management, minimizing the need for extensive human intervention.
By streamlining the workflow, Labellerr enables efficient annotation of large datasets, ultimately saving time and resources for sports organizations.
Robust Segmentation Features
Labellerr incorporates robust segmentation features, including the Segment Anything Model (SAM), specifically tailored for player segmentation tasks in the sports industry.
SAM's advanced algorithms excel at accurately delineating individual players within images and videos, regardless of variations in player positions, movements, or occlusions.
By leveraging SAM's segmentation capabilities, Labellerr ensures the precision and reliability of annotated datasets, essential for training accurate player segmentation models.
Custom Workflows
Labellerr supports customizable workflows tailored to the unique requirements of player segmentation projects in the sports industry.
Users can define custom annotation protocols, designate player categories, and customize labeling criteria to align with specific project objectives.
This flexibility enables annotators to adapt the annotation process to suit different sports and game scenarios, ultimately enhancing the accuracy and effectiveness of player segmentation models.
Active Learning Based Labeling
Labellerr integrates active learning techniques to optimize the annotation process for player segmentation in the sports industry.
By intelligently selecting the most informative samples for annotation, Labellerr maximizes the efficiency of data labeling, reducing manual effort while improving the performance of trained models.
This active learning-based approach enables sports analysts to prioritize labeling efforts on data points that are most beneficial for model training, ultimately leading to more accurate and effective player segmentation models.
Automated Import and Export of Data
Labellerr streamlines the process of importing and exporting data for player segmentation tasks with its automated functionalities.
Whether integrating data from live broadcasts, recorded matches, or other sources, Labellerr simplifies the data management process, enabling seamless integration of datasets into the annotation platform.
Similarly, Labellerr facilitates the export of annotated data, ensuring compatibility with various machine learning frameworks for model training and deployment.
Collaborative Annotation Pipeline
Labellerr allows collaboration among sports analysts and enthusiasts with its collaborative annotation pipeline.
Multiple users can work simultaneously on annotating images and videos of football matches, enabling distributed workflows and real-time collaboration.
This collaborative approach enhances productivity and ensures consistency and accuracy in the labeled dataset, ultimately improving the performance of player segmentation models.
Automated QA (Quality Assurance)
Labellerr integrates automated quality assurance mechanisms to maintain the accuracy and reliability of annotated data for player segmentation tasks in the sports industry.
Advanced algorithms analyze annotations in real-time, flagging inconsistencies or errors for review by sports analysts.
This automated QA process ensures the quality of the labeled dataset, reducing the risk of errors and enhancing the performance of trained models in player segmentation tasks.
Conclusion
In conclusion, Labellerr stands as a transformative tool in advancing player segmentation in the sports industry, particularly in dynamic sports like football.
By addressing the complex challenges associated with annotating images and videos of football matches, Labellerr empowers sports analysts and enthusiasts to create accurate and reliable datasets essential for training cutting-edge player segmentation models.
With features such as active learning-based labeling, automated import and export of data, collaborative annotation pipelines, and automated quality assurance, Labellerr streamlines the annotation process, enhances productivity, and ensures the consistency and accuracy of labeled datasets.
As a result, Labellerr allows the development of precise and effective player segmentation models, ultimately contributing to improved sports analytics, player performance evaluation, and strategic insights for teams and coaches.
Frequently Asked Questions
Q1) What is player segmentation in sports, and why is it important?
Player segmentation in sports involves using computer vision technology to identify and track individual players within images or videos of sports events, such as football matches.
It is essential for analyzing player movements, interactions, and performance during gameplay, providing valuable insights for teams, coaches, and analysts.
Q2)How does Labellerr assist in annotating images and videos for player segmentation tasks?
Labellerr provides advanced annotation tools and features specifically designed for player segmentation in sports.
Its intuitive interface, robust segmentation capabilities, and collaborative annotation pipeline streamline the annotation process, enabling efficient and accurate labeling of player images and videos.

Simplify Your Data Annotation Workflow With Proven Strategies
.png)
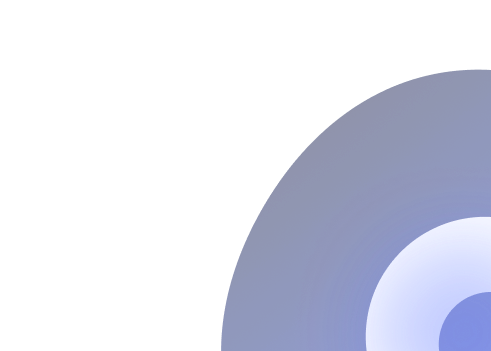