6 Best V7 Alternatives in 2025
In 2025, V7 alternatives like Labellerr, CVAT, iMerit, Encord, Labelbox, and Dataloop offer various features for data labeling. Labellerr stands out with auto-annotation and fast model training.
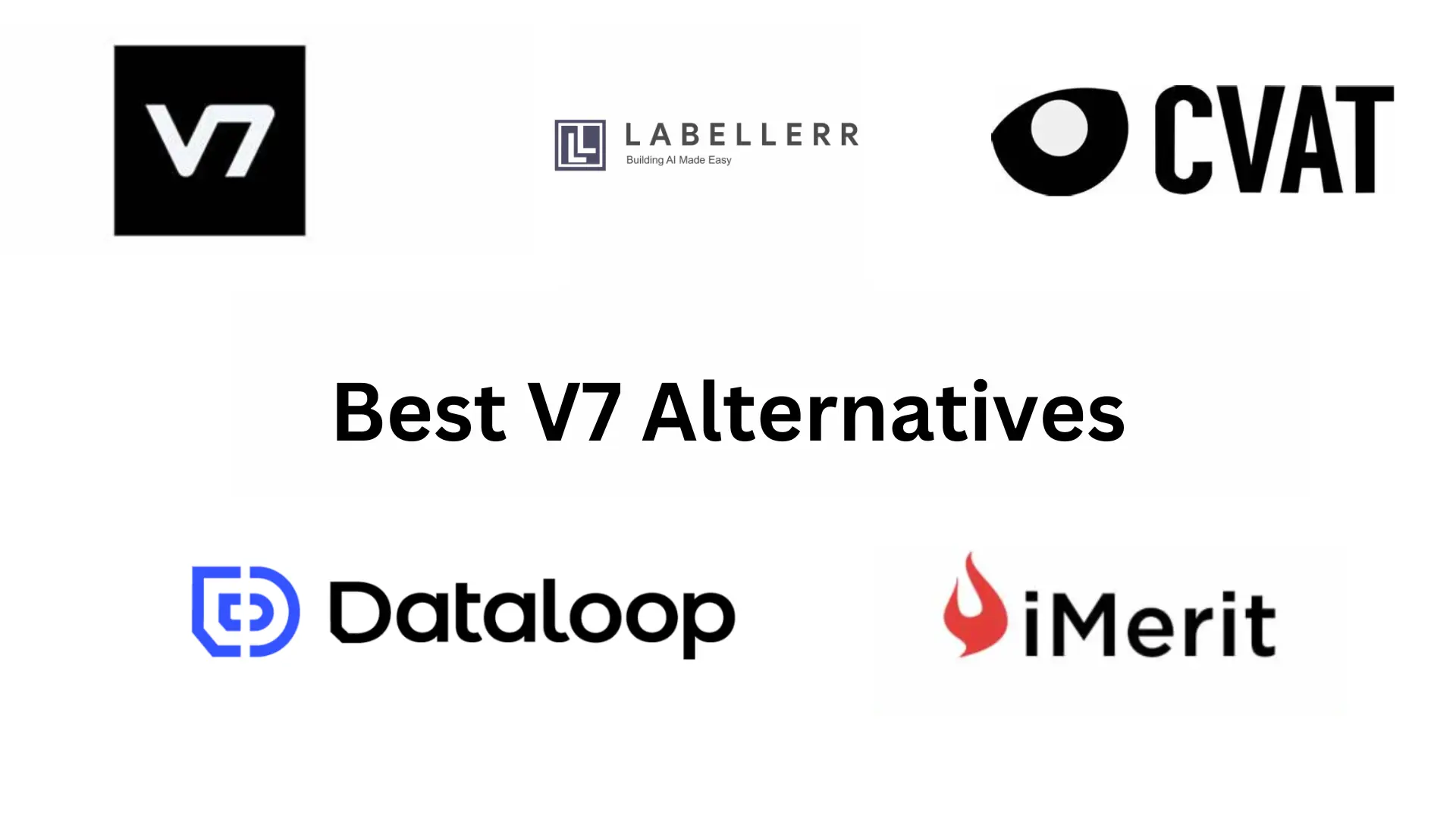
Artificial intelligence is changing quickly, and labeling data is super important for teaching computers and making ML models.
V7 is a recognized data labeling platform, providing essential functionality with an attractive user interface, making it suitable for straightforward annotation tasks.
However, for big business teams, there are some issues like sorting data and showing videos. Also, it might not work well with certain standards.
Advanced commercial teams may encounter several limitations, including:
-> Challenges with data classification
-> Problems with native video rendering
-> Limited compatibility with DICOM standards
-> Absence of organizational groups and project management features
-> Lack of data curation or model evaluation capabilities
-> Pricing structure that may not efficiently accommodate scalability
Due to these considerations, we will investigate alternative options to V7 labs.
We will be looking at other options to find the best fit.
Let's check out Labellerr, CVAT, iMerit, Encord, Labelbox, and Dataloop to see what they offer and which one is good for different needs.
Table Of Content
Here is the list:
1. Labellerr
Labellerr is a robust and feature-rich alternative to V7, designed to streamline and enhance the efficiency of AI teams in the critical tasks of data labeling and model training.
Labellerr provides an auto-annotate feature, that promises a remarkable 99x increase in the speed of generating high-quality labels.
This accelerated annotation process is crucial for significantly reducing the time required for data preparation in various AI applications, including Vision, Natural Language Processing (NLP), and Large Language Models (LLM).
It claims a 10x speed boost in getting models ready for AI deployment, making it an ideal solution for teams seeking rapid iterations in the machine learning workflow.
The platform's automated labeling engine showcases advanced capabilities, incorporating automated annotation, sophisticated analytics, and intelligent quality assurance mechanisms.
This engine efficiently processes vast amounts of data, including millions of images and hours of videos, in just a few weeks.
This scalability and speed make Labellerr a standout choice for projects demanding large-scale data annotation and quick turnaround times.
Labellerr offers a user-friendly experience with a simple UI, making it accessible to both beginners and experienced users.
The platform supports multiple data types, allowing users to seamlessly connect images, videos, PDFs, text, or audio, eliminating the need for multiple tools for different project requirements.
The Smart QA feature, powered by pre-trained models and ground truth-based quality assurance, ensures the accuracy and reliability of labeled data, reducing the time spent on manual quality checks.
In addition to its powerful labeling capabilities, Labellerr integrates seamlessly into the MLOps (Machine Learning Operations) workflow.
It supports easy export in various formats, such as CSV, JSON, COCO, Pascal VOC, and custom formats, facilitating one-click push to popular ML training engines like GCP Vertex AI, AWS SageMaker, or custom environments.
The platform's advanced project management module, coupled with human-in-the-loop automation, contributes to significant time and cost savings—claimed to be at least 10x.
Labellerr's commitment to user support is evident through its 24/7 tech support, ensuring users receive timely assistance on crucial projects.
The platform's comprehensive suite of features, including automated labeling, multiple data type support, smart QA, MLOps integration, and advanced project management, positions it as a compelling alternative to V7, addressing the challenges faced by advanced commercial teams.
With an emphasis on accuracy, reduced time to data preparation, and overall cost-effectiveness, Labellerr stands out as a valuable tool in the AI and machine learning landscape.
Here are some key things to know about Labellerr:
(i) Auto-Annotate Acceleration:
-> Achieves a remarkable 99x increase in labeling speed.
-> Significantly reduces the time required for data preparation.
(ii) Model Training Efficiency
-> Accelerates Vision, NLP, and LLM model preparation for AI deployment by 10x.
-> Ideal for fast-tracking the machine learning workflow.
Automated Labeling Engine
-> Processes millions of images and hours of videos within a few weeks.
-> Demonstrates scalability and speed in large-scale data annotation.
User-Friendly Interface
-> Intuitive UI designed for both beginners and experienced users.
-> Simplifies the data labeling and model training process.
Multiple Data Type Support
-> Connects images, videos, PDFs, text, or audio seamlessly.
-> Eliminates the need for multiple tools for different project requirements.
Smart QA (Quality Assurance)
-> Incorporates pre-trained models and ground truth-based QA.
-> Ensures accuracy and reliability of labeled data, reducing manual checks.
MLOps Integration
-> Streamlines integration into the MLOps workflow.
-> Supports easy export in various formats, enabling one-click push to popular ML training engines.
Advanced Project Management
-> Human-in-the-loop automation for efficient project management.
-> Contributes to significant time and cost savings, claimed to be at least 10x.
2. CVAT
CVAT, which stands for Computer Vision Annotation Tool, is a platform designed for annotating data, especially in the field of computer vision.
What makes it unique is that it relies on the power of a community, making it a solution driven by collaboration for labeling data.
CVAT helps people mark and describe things in pictures, especially when computers are learning to understand those images.
The special thing about CVAT is that it's like a team effort - people from different places work together to make it better at labeling and understanding pictures.
Here are some key things to know about CVAT:
(i) Open Source and Free
CVAT is like a free and open book. Anyone can use it without paying money. This makes it great for small businesses, student projects, and researchers who don't want to spend a lot of money.
(ii) Community Power
Lots of people from around the world help make CVAT better. It's like a team effort where everyone can pitch in and make it even more useful.
(iii) Labeling Engine
The core of AI focused on data involves managing datasets through curation, annotation, training, and continuous improvement.
CVAT excels in this by offering features for labeling with bounding boxes, polygons, and key points.
(iv) Customizable
People can customize CVAT according to their requirements by adding special plugins, creating personalized workflows, or incorporating support for new types of data.
(v) Simple to Use
Whether you're just starting or you're already a pro, CVAT is made for everyone.
It's designed to be easy for beginners and still useful for experts.
(vi) Not Perfect for Everything
Although CVAT provides various annotation tools, it may lack certain advanced features that some users might need for their particular annotation tasks.
3. iMerit
iMerit is recognized as a service provider specializing in data labeling, offering solutions for annotations and data management.
iMerit distinguishes itself by taking a service-oriented approach to data annotation when compared to traditional labeling platforms.
Key Features and Benefits of iMerit:
(i) Tailored Solutions
iMerit stands out by offering customizable solutions to meet specific needs, whether it's annotating, analyzing, categorizing, or segmenting data.
(ii) Insightful Metrics
Users can gain valuable insights through metrics provided by iMerit. These metrics include details like the annotator's working hours, the number of objects annotated per hour, and more, helping in understanding and optimizing the annotation process.
(iii) Free Trial
iMerit allows users to explore its services with a free trial. This allows users to experience the platform before making any commitments.
(iv) Pricing Transparency
While iMerit provides a free trial, its pricing plan is not openly disclosed on its website. Users might need to inquire directly to get details about the cost of their services.
(v) User Interface Consideration
It's worth noting that iMerit's user interface might be less intuitive and user-friendly, especially for beginners.
This suggests that there might be a learning curve for those new to the platform.
Therefore, iMerit offers a service-focused approach with customizable solutions and insightful metrics.
However, potential users may need to directly inquire about pricing, and the platform may have a learning curve for those new to data annotation.
4. Encord
Encord stands out as a leading platform offering an alternative for constructing annotation workflows, managing visual data, addressing data errors, and monitoring model performance.
Key Features:
(i) AI-assisted labeling and Workflow Tooling
Encord is a cutting-edge platform that leverages micro-models, providing assistance in AI-assisted labeling and workflow creation.
It is well-suited for various annotation and labeling tasks, QA workflows, and the training of computer vision models.
(ii) Tailored for Computer Vision
Specifically designed for computer vision applications, Encord natively supports a diverse range of annotation types, including bounding box, polygon, polyline, instance segmentation, key points, classification, and more.
(iii) Use-Case-Specific Annotations
Encord caters to specific needs, offering annotations like native DICOM and NIfTI for medical imaging and features designed for Synthetic Aperture Radar (SAR) data in geospatial applications.
(iv) MLOps Workflows
Integrated workflows for MLOps (Machine Learning Operations) empower computer vision and machine learning teams.
This helps in identifying edge cases and gaps in training data, enabling the generation of augmented data to enhance label quality.
(v) Collaboration and Quality Assurance
Encord streamlines collaboration, annotator management, and quality assurance workflows.
This ensures precise tracking of annotator performance and enhances label quality.
(vi) Robust Security
The platform prioritizes robust security features, including label audit trails, encryption, and compliance with FDA, CE, and HIPAA standards.
(vii) Advanced SDK and API Access
Encord offers an advanced Python SDK and API access, along with easy export capabilities in JSON and COCO formats.
This enhances flexibility and integration with external systems.
(viii) Data Quality Enhancement
Encord automates the identification and correction of dataset biases and errors, such as outliers, duplication, and labeling mistakes.
(ix) Integrated Tagging
The platform supports integrated tagging for both data and labels, including tagging for outliers.
(x) Quality Metrics
Encord employs quality metrics (data, label, and model) to assess and improve performance across the entire ML pipeline, covering data curation, data labeling, and model training.
5. Labelbox
Labelbox specializes in providing data labeling services tailored for large-scale computer vision and NLP projects within enterprises.
The platform stands out for its user-friendly and modular setup, allowing easy customization to align with existing workflows.
Users can employ GraphQL and Python APIs to seamlessly integrate data into Labelbox, facilitating automated data ingestion into their training dataset environment.
Here are some key things to know about Labelbox:
(i) Supports Various Labeling Scenarios
Labelbox supports various data labeling scenarios, including simultaneous labeling by internal and external teams, labeling by an integrated managed data labeling workforce, and model-assisted labeling using the Labelbox Prediction API.
(ii) API-Driven Labeling Queue Prioritization
It offers a useful tool for auto-prioritizing the most critical data for labeling through API-driven labeling queue prioritization.
(iii) Enhanced Speed and Accuracy
Enterprises can significantly enhance the speed and accuracy of data annotation by leveraging the mentioned features. Integrated analytics and histograms allow for performance comparison among different annotators.
(iv) Dataset Management
While Labelbox excels in data labeling capabilities, it falls somewhat short in dataset management features. Basic storage and organization features are available for labeling datasets, but advanced features like version control or comprehensive search functionalities are lacking.
(v) Free Plan and Pricing
Labelbox provides a free plan for test-driving the platform, limited to image and video data labeling. Pro and Enterprise users can opt for on-demand pricing, and managed data labeling services start at $6 per labeling hour.
Hence, Labelbox offers robust data labeling capabilities, but users may find limitations in dataset management features. The platform's pricing plans cater to different user needs, with a free plan for exploration and various options for more extensive usage.
6. Dataloop
Dataloop not only offers data labeling services but also provides a comprehensive set of tools to manage data workflows and establish (semi-) automated deployment pipelines for new models.
This enables ML teams to iterate on models more swiftly, minimizing deployment risks.
Here are some key things to know about Dataloop:
(i) Data Labeling Support
Dataloop supports annotations for both images and videos.
The platform includes automation annotation tools such as an AI assistant that automatically converts four single points into multi-vertice polygons, model-assisted labeling capabilities similar to Labelbox, and smart object tracking for auto-duplicating annotations between video frames and sequenced images.
(ii) Accelerated Data Labeling
To expedite the data labeling process, Dataloop allows users to augment their workforce by hiring additional individuals from Dataloop’s fully managed workforce network.
(iii) Robust Data Labeling Toolkit
Dataloop's toolkit for data labeling is robust, providing a variety of features.
However, it's worth noting that interpolation outside of bounding boxes for videos is not offered.
(iv) Pricing Model
Dataloop operates on an on-demand pricing model, offering flexibility for users based on their specific needs.
Hence, Dataloop offers a strong suite of tools not just for data labeling but also for managing data workflows and automating model deployment.
While their data labeling toolkit is comprehensive, users should be aware of the absence of interpolation outside of bounding boxes for videos.
The platform's on-demand pricing model adds flexibility for users in terms of cost management.
Conclusion
In the fast-evolving world of artificial intelligence, data labeling plays a crucial role in training machine learning models.
Among the myriad platforms available, V7 Labs has been a recognized player, offering essential functionality with an appealing user interface.
However, for advanced commercial teams, encountering limitations in areas like data classification, video rendering, and compatibility with standards like DICOM has prompted a search for alternatives.
We explored various alternative data labeling platforms like Labellerr, CVAT, iMerit, Encord, Labelbox, and Dataloop to understand their unique features and suitability for diverse needs.
Frequently Asked Questions
1. What are the best V7 alternatives?
The best alternatives to V7, considering factors like data classification, video rendering, compatibility with standards, and overall features, include Labellerr, CVAT, iMerit, Encord, Labelbox, and Dataloop.
Each of these platforms offers unique features and strengths, making them suitable alternatives for advanced commercial teams seeking robust data labeling solutions.
Additionally, the alternatives mentioned by reviewers and users in the content align with the considerations for finding suitable replacements for V7.
2. Why do we use V7?
We use V7 because it empowers our machine learning teams by providing a seamless and efficient platform for annotating data, training models, and establishing ground truth.
V7 significantly accelerates our labeling process, boasting a remarkable 9–10 times speed improvement compared to VGG.
The appeal lies in its commercial off-the-shelf nature, making it readily available, and its user-friendly interface, which is intuitive and easily navigable even for non-technical individuals.
The simplicity and effectiveness of V7 contribute to streamlining our annotation and model training workflows, ultimately enhancing overall efficiency in our machine learning endeavors.
3. Is V7 a data training platform?
V7 stands out as a data training platform prioritizing automation and user-friendly functionality.
With its emphasis on advanced machine learning capabilities, a standout feature is Auto-Annotate, which harnesses the power of AI to swiftly segment objects in images.
This innovative feature results in a substantial reduction in annotation time, potentially achieving up to a 10x increase in efficiency.

Simplify Your Data Annotation Workflow With Proven Strategies
.png)
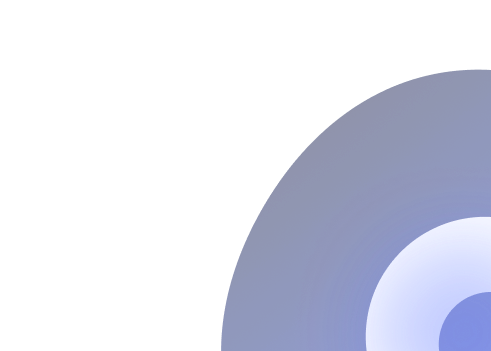