These 10 datasets you won't find on Kaggle-Part 1
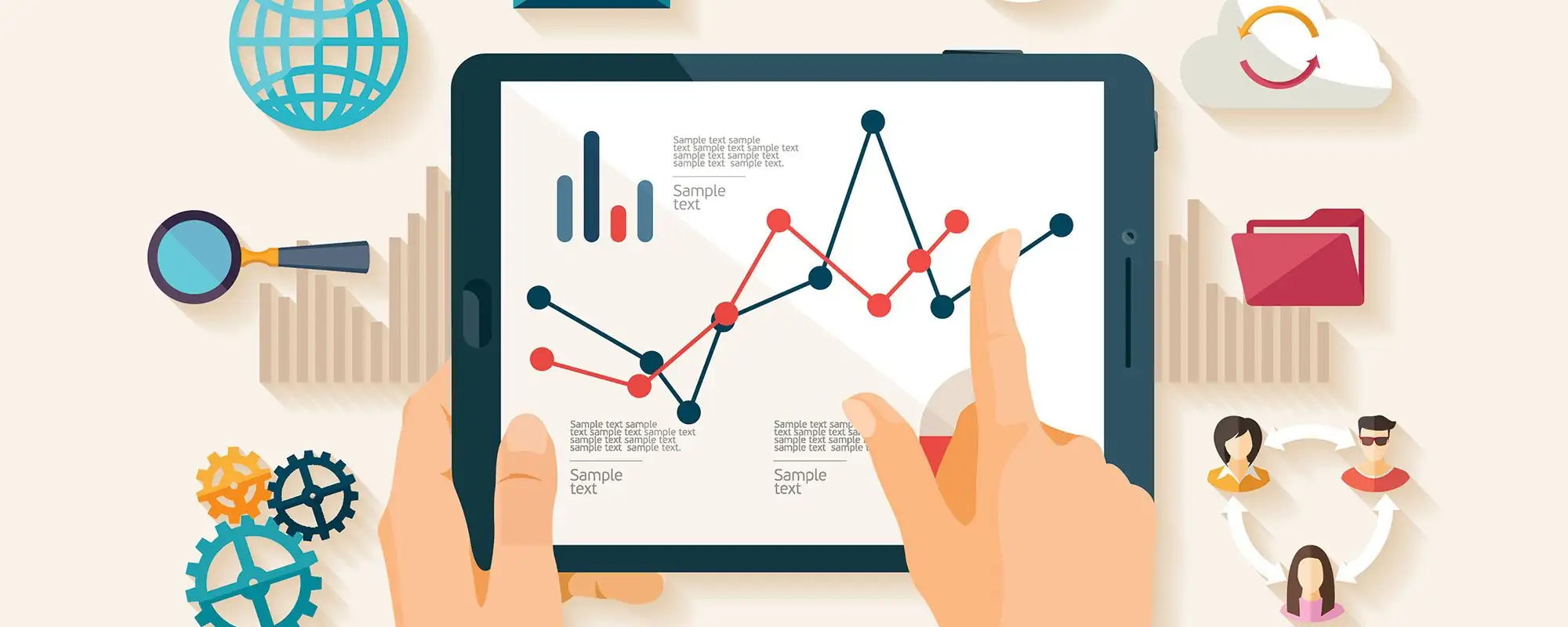
Are you someone who deals with datasets every new day and are always looking for a new variety of datasets? If you are a data enthusiast, then CVPR (IEEE/CVF Computer Vision and Pattern Recognition Conference (CVPR)) is something that is extremely important for you. Recently, they have published a list of 10 Datasets you might not find on Kaggle that might be of your interest if you are related to the Computer Vision industry.
Kaggle is for those interested in machine learning and data science. Users of Kaggle can work together, access and share datasets, use notebooks with GPU integration, and compete with several other data professionals to tackle data science problems.
Here we have listed 10 Datasets you might not find on Kaggle that might be of use to you. But first, let’s understand CVPR in detail.
1. FS6D: Few-Shot 6D Pose Estimation of Novel Objects
Author: Yisheng He, Yao Wang, Haoqiang Fan, Jian Sun, Qifeng Chen
The close-set assumption and 6D object pose estimation networks' reliance on high-fidelity object CAD models prevent them from scaling to huge numbers of object instances. In this work, we investigate the few-shot 6D object pose estimation, an open set problem that involves predicting the 6D posture of an unknown object from a small number of support views without additional training. To solve the issue, they have emphasized the significance of thoroughly examining the look and geometry relationship between the provided supported views and query scene patches, and they have suggested a framework for matching dense RGBD prototypes by extracting and transforming dense RGBD prototypes.
They have also demonstrated how important priors from various looks and shapes are to generalization under the issue set, and we suggest ShapeNet6D, a sizable RGBD photorealistic dataset, for network pre-training. The domain gap from the synthesis dataset is also removed using a quick and efficient online texture blending method, which improves appearance diversity at a minimal cost. In order to aid future research, we build benchmarks using well-known datasets and propose potential solutions to this issue.
The dataset:
1. The ImageNet dataset contains 14,197,122 annotated images according to the WordNet hierarchy. Since 2010 the dataset has been used in the ImageNet Large Scale Visual Recognition Challenge (ILSVRC), a benchmark in image classification and object detection. The publicly released dataset contains a set of manually annotated training images. A set of test images is also released, with the manual annotations withheld. ILSVRC annotations fall into one of two categories: (1) image-level annotation of a binary label for the presence or absence of an object class in the image, e.g., “there are cars in this image” but “there are no tigers,” and (2) object-level annotation of a tight bounding box and class label around an object instance in the image, e.g., “there is a screwdriver centered at position (20,25) with a width of 50 pixels and height of 30 pixels”. The ImageNet project does not own the copyright of the images, therefore only thumbnails and URLs of images are provided.
- A Total number of non-empty WordNet synsets: 21841
- Total number of images: 14197122
- Number of images with bounding box annotations: 1,034,908
- Number of synsets with SIFT features: 1000
- Number of images with SIFT features: 1.2 million
2. The MS COCO (Microsoft Common Objects in Context) dataset is a large-scale object detection, segmentation, key-point detection, and captioning dataset. The dataset consists of 328K images.
Splits: The first version of the MS COCO dataset was released in 2014. It contains 164K images split into training (83K), validation (41K) and test (41K) sets. In 2015 an additional test set of 81K images was released, including all the previous test images and 40K new images.
Based on community feedback, in 2017 the training/validation split was changed from 83K/41K to 118K/5K. The new split uses the same images and annotations. The 2017 test set is a subset of 41K images of the 2015 test set. Additionally, the 2017 release contains a new unannotated dataset of 123K images.
3. ShapeNet is a large-scale repository for 3D CAD models developed by researchers from Stanford University, Princeton University and the Toyota Technological Institute in Chicago, USA. The repository contains over 300M models with 220,000 classified into 3,135 classes arranged using WordNet hypernym-hyponym relationships. The ShapeNet Parts subset contains 31,693 meshes categorized into 16 common object classes (i.e. table, chair, plane etc.). Each shape ground truth contains 2-5 parts (with a total of 50 part classes).
4. The YCB-Video dataset is a large-scale video dataset for 6D object pose estimation. provides accurate 6D poses of 21 objects from the YCB dataset observed in 92 videos with 133,827 frames.
You can download the data from here
Related Research Paper for your reference
DenseFusion: 6D Object Pose Estimation by Iterative Dense Fusion published on CVPR 2019 by Chen Wang, Danfei Xu, Yuke Zhu, Roberto Martín-Martín, Cewu Lu, Li Fei-Fei, Silvio Savarese
The surprising impact of mask-head architecture on novel class segmentation published on ICCV 2021 by Vighnesh Birodkar, Zhichao Lu, Siyang Li, Vivek Rathod, Jonathan Huang ·
Learning ABCs: Approximate Bijective Correspondence for isolating factors of variation with weak supervision published on CVPR 2022 by Kieran A. Murphy, Varun Jampani, Srikumar Ramalingam, Ameesh Makadia
Leveraging redundancy in attention with Reuse Transformers published by Srinath Bhojanapalli, Ayan Chakrabarti, Andreas Veit, Michal Lukasik, Himanshu Jain, Frederick Liu, Yin-Wen Chang, Sanjiv Kumar
2. MobRecon: Mobile-Friendly Hand Mesh Reconstruction From Monocular Image
Author: Xingyu Chen, Yufeng Liu, Yajiao Dong, Xiong Zhang, Chongyang Ma, Yanmin Xiong, Yuan Zhang, Xiaoyan Guo
In this paper, they have suggested a paradigm for single-view hand mesh rebuilding that combines high reconstruction accuracy with quick inference speed and temporal coherence. They have specifically suggested simple yet powerful stacking structures for 2D encoding. They offer a useful graph operator for 3D decoding called depth-separable spiral convolution. For bridging the distinction between 2D and 3D representations, we also introduce a unique feature lifting module. This module starts with a map-based position regression (MapReg) block to merge the benefits of both the position regression and heatmap encoding paradigms for better temporal coherence and 2D accuracy.
Pose pooling and pose-to-vertex lifting techniques, which convert 2D pose encodings to semantic properties of 3D vertices, come after MapReg. Overall, the MobRecon hand reconstruction framework has low computational costs, small model sizes, and a fast inference speed of 83FPS on an Apple A14 CPU. Numerous tests on well-known datasets including FreiHAND, RHD, and HO3Dv2 show that our MobRecon performs better in terms of reconstruction accuracy and temporal coherence.
The datasets:
1. The ImageNet dataset contains 14,197,122 annotated images according to the WordNet hierarchy. Since 2010 the dataset has been used in the ImageNet Large Scale Visual Recognition Challenge (ILSVRC), a benchmark in image classification and object detection. The publicly released dataset contains a set of manually annotated training images. A set of test images is also released, with the manual annotations withheld. ILSVRC annotations fall into one of two categories: (1) image-level annotation of a binary label for the presence or absence of an object class in the image, e.g., “there are cars in this image” but “there are no tigers,” and (2) object-level annotation of a tight bounding box and class label around an object instance in the image, e.g., “there is a screwdriver centered at position (20,25) with a width of 50 pixels and height of 30 pixels”. The ImageNet project does not own the copyright of the images, therefore only thumbnails and URLs of images are provided.
- Total number of non-empty WordNet synsets: 21841
- Total number of images: 14197122
- Number of images with bounding box annotations: 1,034,908
- Number of synsets with SIFT features: 1000
- Number of images with SIFT features: 1.2 million
2. FreiHAND is a 3D hand pose dataset which records different hand actions performed by 32 people. For each hand image, MANO-based 3D hand pose annotations are provided. It currently contains 32,560 unique training samples and 3960 unique samples for evaluation. The training samples are recorded with a green screen background allowing for background removal. In addition, it applies three different post-processing strategies to training samples for data augmentation. However, these post-processing strategies are not applied to evaluation samples.
3. Rendered Hand Pose (RHD) is a dataset for hand pose estimation. It provides segmentation maps with 33 classes: three for each finger, palm, person, and background. The 3D kinematic model of the hand provides 21 key points per hand: 4 key points per finger and one key point close to the wrist.
You can download the data from here
Related Research Paper for your reference
Exploring the Limits of Language Modeling published by Rafal Jozefowicz, Oriol Vinyals, Mike Schuster, Noam Shazeer, Yonghui Wu
FrankMocap: A Monocular 3D Whole-Body Pose Estimation System via Regression and Integration published by Yu Rong, Takaaki Shiratori, Hanbyul Joo
Camera-Space Hand Mesh Recovery via Semantic Aggregation and Adaptive 2D-1D Registration published on CVPR 2021 by Xingyu Chen, Yufeng Liu, Chongyang Ma, Jianlong Chang, Huayan Wang, Tian Chen, Xiaoyan Guo, Pengfei Wan, Wen Zheng
Learning to Estimate 3D Hand Pose from Single RGB Images published on ICCV 2017 by Christian Zimmermann, Thomas Brox ·
3. Keypoint Transformer: Solving Joint Identification in Challenging Hands and Object Interactions for Accurate 3D Pose Estimation
Author: Shreyas Hampali, Sayan Deb Sarkar, Mahdi Rad, Vincent Lepetit
They have suggested a reliable and precise technique for determining the 3D postures of two hands interacting closely from a single color photograph. This is a particularly difficult condition because there could be significant occlusions and numerous confusions between the joints. Modern techniques address this issue by concurrently addressing the issues of localizing the joints and identifying them by regressing a heatmap for each joint. In this work, we suggest separating these tasks by using a CNN to first locate joints as 2D key points and then self-attention between CNN features at these key points to associate them with the associated hand joint.
The resulting architecture, which they have referred to as "Keypoint Transformer," is very effective because it delivers cutting-edge performance on the InterHand2.6M dataset using just about half the model parameters. They have also demonstrated how it can be quickly expanded to accurately predict the 3D position of an object being held in one or two hands. In addition, they have developed a brand-new dataset with over 75,000 photos of two hands moving an object that is completely annotated in 3D and will be released to the public.
The dataset:
1. A hand-object interaction dataset with 3D pose annotations of hand and object. The dataset contains 66,034 training images and 11,524 test images from a total of 68 sequences. The sequences are captured in multi-camera and single-camera setups and contain 10 different subjects manipulating 10 different objects from the YCB dataset. The annotations are automatically obtained using an optimization algorithm. The hand pose annotations for the test set are withheld and the accuracy of the algorithms on the test set can be evaluated with standard metrics using the CodaLab challenge submission(see project page). The object pose annotations for the test and train set are provided along with the dataset.
2. The InterHand2.6M dataset is a large-scale real-captured dataset with accurate GT 3D interacting hand poses, used for 3D hand pose estimation The dataset contains 2.6M labeled single and interacting hand frames.
You can download the data from here
Related Research Paper for your reference
FrankMocap: A Monocular 3D Whole-Body Pose Estimation System via Regression and Integration published by Yu Rong, Takaaki Shiratori, Hanbyul Joo
ContactPose: A Dataset of Grasps with Object Contact and Hand Pose published on ECCV 2020 by Samarth Brahmbhatt, Chengcheng Tang, Christopher D. Twigg, Charles C. Kemp, James Hays
InterHand2.6M: A Dataset and Baseline for 3D Interacting Hand Pose Estimation from a Single RGB Image published on ECCV 2020 by Gyeongsik Moon, Shoou-I Yu, He Wen, Takaaki Shiratori, Kyoung Mu Lee
Interacting Attention Graph for Single Image Two-Hand Reconstruction published on CVPR 2022 by Mengcheng Li, Liang An, Hongwen Zhang, Lianpeng Wu, Feng Chen, Tao Yu, Yebin Liu
4. Focal Click: Towards Practical Interactive Image Segmentation
Author: Xi Chen, Zhiyan Zhao, Yilei Zhang, Manni Duan, Donglian Qi, Hengshuang Zhao
Users can derive target masks using interactive segmentation by clicking in a positive or negative way. The gap between intellectual approaches and industrial needs has been extensively studied, but it persists for two reasons: first, current models are inefficient enough to operate on low-power devices, and second, they perform poorly when used to improve existing masks because they could not avoid destroying the correct part. By anticipating and adjusting the mask in certain locations, FocalClick addresses both problems at once. A coarse segmentation on the Target Crop and a local improvement on the Focus Crop is used to break down the slow prediction made on the entire image into two quick inferences.
They have defined a subtask called Interactive Mask Correction and suggest Progressive Merge as the way to make the model function with preexisting masks. Users of Progressive Merge can efficiently modify any preexisting mask since it uses morphological information to determine where to update and where to preserve. With much fewer FLOPs than SOTA approaches, FocalClick outperforms them. Additionally, it demonstrates a clear advantage when modifying existing masks.
The dataset:
1. DAVIS-585-A dataset for interactive segmentation with simulated initial masks.
2. The MS COCO (Microsoft Common Objects in Context) dataset is a large-scale object detection, segmentation, key-point detection, and captioning dataset. The dataset consists of 328K images.
Splits: The first version of the MS COCO dataset was released in 2014. It contains 164K images split into training (83K), validation (41K) and test (41K) sets. In 2015 an additional test set of 81K images was released, including all the previous test images and 40K new images.
Based on community feedback, in 2017 the training/validation split was changed from 83K/41K to 118K/5K. The new split uses the same images and annotations. The 2017 test set is a subset of 41K images of the 2015 test set. Additionally, the 2017 release contains a new unannotated dataset of 123K images.
3. The Densely Annotation Video Segmentation dataset (DAVIS) is a high-quality and high-resolution densely annotated video segmentation dataset under two resolutions, 480p and 1080p. There are 50 video sequences with 3455 densely annotated frames at the pixel level. 30 videos with 2079 frames are for training and 20 videos with 1376 frames are for validation.
4. LVIS is a dataset for long-tail instance segmentation. It has annotations for over 1000 object categories in 164k images.
You can download the data from here
Related Research Paper for your reference
Naive-Student: Leveraging Semi-Supervised Learning in Video Sequences for Urban Scene Segmentation published on ECCV 2020 by Liang-Chieh Chen, Raphael Gontijo Lopes, Bowen Cheng, Maxwell D. Collins, Ekin D. Cubuk, Barret Zoph, Hartwig Adam, Jonathon Shlens ·
EdgeFlow: Achieving Practical Interactive Segmentation with Edge-Guided Flow
Published by Yuying Hao, Yi Liu, Zewu Wu, Lin Han, Yizhou Chen, Guowei Chen, Lutao Chu, Shiyu Tang, Zhiliang Yu, Zeyu Chen, Baohua Lai ·
Open-vocabulary Object Detection via Vision and Language Knowledge Distillation
Published on ICLR 2022 by Xiuye Gu, Tsung-Yi Lin, Weicheng Kuo, Yin Cui ·
Exploring Plain Vision Transformer Backbones for Object Detection published by Yanghao Li, Hanzi Mao, Ross Girshick, Kaiming He
5. JRDB-Act: A Large-Scale Dataset for Spatio-Temporal Action, Social Group and Activity Detection
Author: Mahsa Ehsanpour, Fatemeh Saleh, Silvio Savarese, Ian Reid, Hamid Rezatofighi
Advancements in the interpretation of human-centered visual situations have been made possible by the availability of large-scale video action understanding datasets. However, learning to recognise human actions and their social interactions in an unrestricted real-world environment made up of many people, with potentially highly unbalanced and long-tailed distributed action labels from a stream of sensory data captured from a mobile robot platform, remains a difficult task, in part because there isn't a comprehensive, accurate large-scale dataset. We offer JRDB-Act in this study as an expansion of the current JRDB, which is collected by a social mobile manipulator and reflects a true distribution of human daily-life actions in an academic campus context.
One pose-based action label and several additional (optional) interaction-based action labels are attached to each human bounding box. Additionally, JRDB-Act offers social group annotation, making it easier to group people based on how they interact in a scene and deduce their social activities (common activities in each social group). The level of the annotators' confidence is noted next to each annotated label in JRDB-Act, which aids in the creation of trustworthy evaluation methods. We create an end-to-end trainable pipeline to learn and infer these tasks, namely individual action and social group recognition, in order to show how one can successfully use such annotations.
The dataset:
1. JRDB-Act is an extension of the JRDB dataset to create a large-scale multi-modal dataset for Spatio-temporal action, social group and activity detection.
JRDB-Act has been densely annotated with atomic actions, and comprises over 2.8M action labels, constituting a large-scale Spatio-temporal action detection dataset. Each human bounding box is labeled with one pose-based action label and multiple (optional) interaction-based action labels. Moreover, JRDB-Act comes with social group identification annotations conducive to the task of grouping individuals based on their interactions in the scene to infer their social activities (common activities in each social group).
2. The Kinetics dataset is a large-scale, high-quality dataset for human action recognition in videos. The dataset consists of around 500,000 video clips covering 600 human action classes with at least 600 video clips for each action class. Each video clip lasts around 10 seconds and is labeled with a single action class. The videos are collected from YouTube.
3. Volleyball is a video action recognition dataset. It has 4830 annotated frames that were handpicked from 55 videos with 9 player action labels and 8 team activity labels. It contains group activity annotations as well as individual activity annotations.
You can download the data from here
Related Research Paper for your reference
JRDB-Act: A Large-scale Dataset for Spatio-temporal Action, Social Group and Activity Detection published on CVPR 2022 by Mahsa Ehsanpour, Fatemeh Saleh, Silvio Savarese, Ian Reid, Hamid Rezatofighi
JRDB-Pose: A Large-scale Dataset for Multi-Person Pose Estimation and Tracking
Published by Edward Vendrow, Duy Tho Le, Hamid Rezatofighi
MoViNets: Mobile Video Networks for Efficient Video Recognition published on CVPR 2021 by Dan Kondratyuk, Liangzhe Yuan, Yandong Li, Li Zhang, Mingxing Tan, Matthew Brown, Boqing Gong
Spatio-Temporal Dynamic Inference Network for Group Activity Recognition published on ICCV 2021 by Hangjie Yuan, Dong Ni, Mang Wang
Want to know more about the datasets that you might not find on Kaggle? Check out Part 2 https://www.labellerr.com/blog/these-10-datasets-you-wont-find-on-kaggle-part2/

Simplify Your Data Annotation Workflow With Proven Strategies
.png)
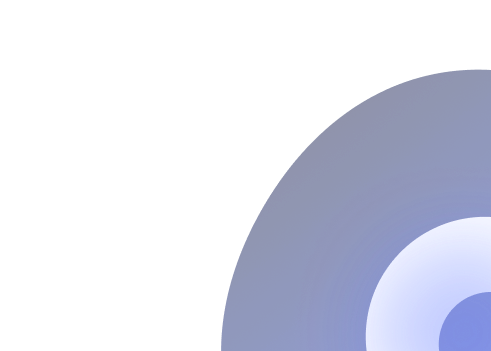