7 Best Text Annotation and Labeling Services Providers in 2024
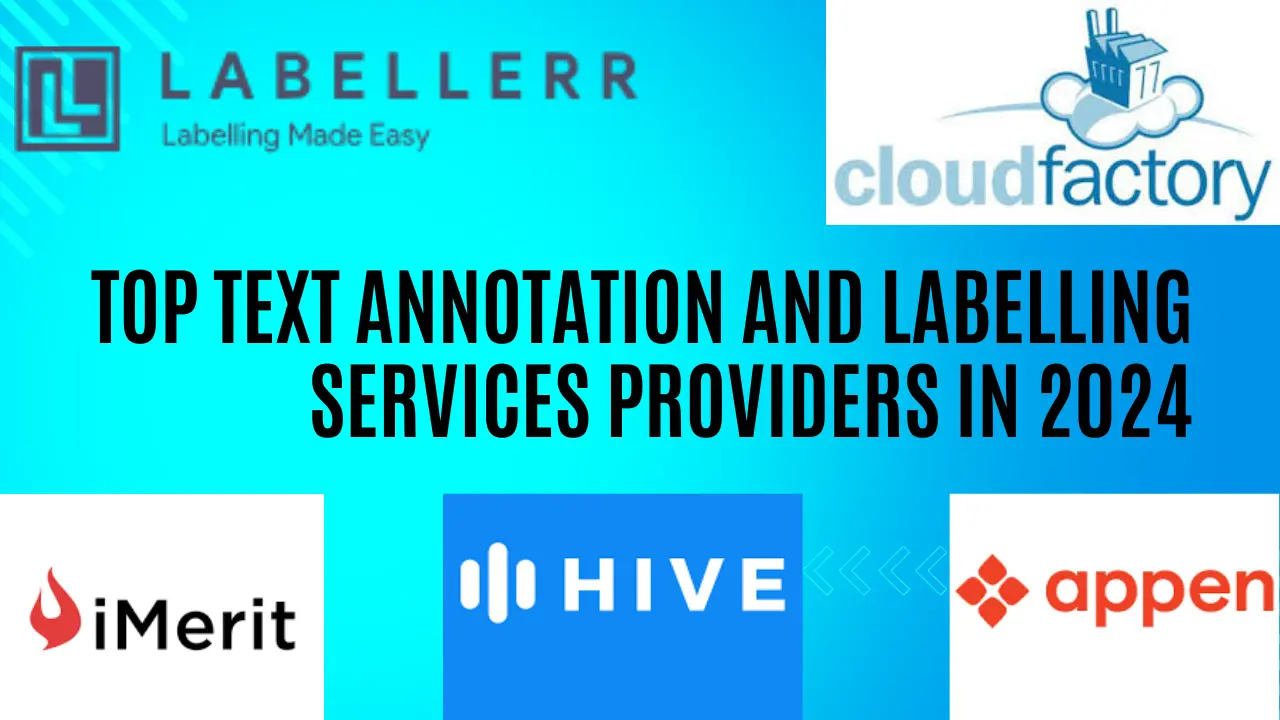
In the realm of machine learning and artificial intelligence, data serves as the fundamental building block for creating intelligent systems.
A critical step in preparing data for training models is annotation and labeling, where specific elements within a dataset are tagged with meaningful information.
Text annotation and labeling services play an essential role in this process, providing the groundwork for applications such as natural language processing (NLP), sentiment analysis, named entity recognition, and more.
These services have gained prominence as the need for accurately annotated data has increased, along with the complexity and scale of AI projects.
By leveraging these services, organizations can significantly accelerate the data preparation phase and ensure high-quality labeled datasets, thereby improving model performance and reliability.
This blog will explore some of the top text annotation and labeling service providers.
Features to Look in Annotation and Labeling Services
When evaluating top text annotation and labeling service providers, several key features are critical to ensuring a smooth, efficient, and accurate labeling process. Here's what to consider when choosing the best platform for your text annotation needs:
1. Flexibility and Customization
Look for a platform that allows customization of annotation workflows and supports a range of text-based tasks.
This flexibility is crucial as it enables users to adapt to different project requirements, whether it's named entity recognition, sentiment analysis, text classification, or any other specific annotation needs.
2. Collaboration and Team Management
A strong collaborative environment is essential, especially for larger projects involving multiple annotators.
The platform should offer tools for task assignment, progress tracking, and quality control. Features like real-time collaboration, review workflows, and consensus mechanisms help ensure consistency and improve overall project management.
3. Quality Control and Assurance
High-quality data is crucial for effective machine learning. The platform should include robust quality control features, such as review processes, inter-annotator agreement checks, and automated error detection.
This ensures that the labeled data is accurate and reliable, reducing the need for rework.
4. Automation and Active Learning
Automation features can significantly speed up the annotation process. Look for platforms that offer active learning, where a model assists annotators by making predictions or suggesting labels.
This can reduce the workload on human annotators while improving efficiency and consistency.
5. Integration and Export Options
The ability to integrate with existing machine learning pipelines is vital. Check whether the platform supports various data export formats and can be easily integrated with other tools or frameworks. This flexibility allows for seamless data flow between different stages of your machine learning process.
6. Security and Data Privacy
Data security is a top concern, especially when working with sensitive information. Ensure the platform has robust security measures, including encryption, secure data storage, and access controls. Compliance with industry standards and regulations (such as GDPR) is also crucial.
7. Usability and User Experience
A user-friendly interface can make a significant difference in productivity. Consider platforms with intuitive interfaces that are easy to navigate and understand. This is especially important when onboarding new annotators or working with diverse teams.
8. Scalability and Performance
Your chosen platform should be able to handle projects of varying sizes, from small-scale tasks to large enterprise projects. Scalability ensures that the platform can grow with your needs without compromising performance or speed.
9. Support and Community
Reliable customer support and an active community can be invaluable. Look for platforms that offer technical support, comprehensive documentation, and user communities. These resources can help troubleshoot issues, share best practices, and provide additional guidance.
By considering these features, you can select a text annotation and labeling service provider that meets your project's requirements and helps you build high-quality datasets for your machine learning and AI applications.
Here is the List
1) Labellerr
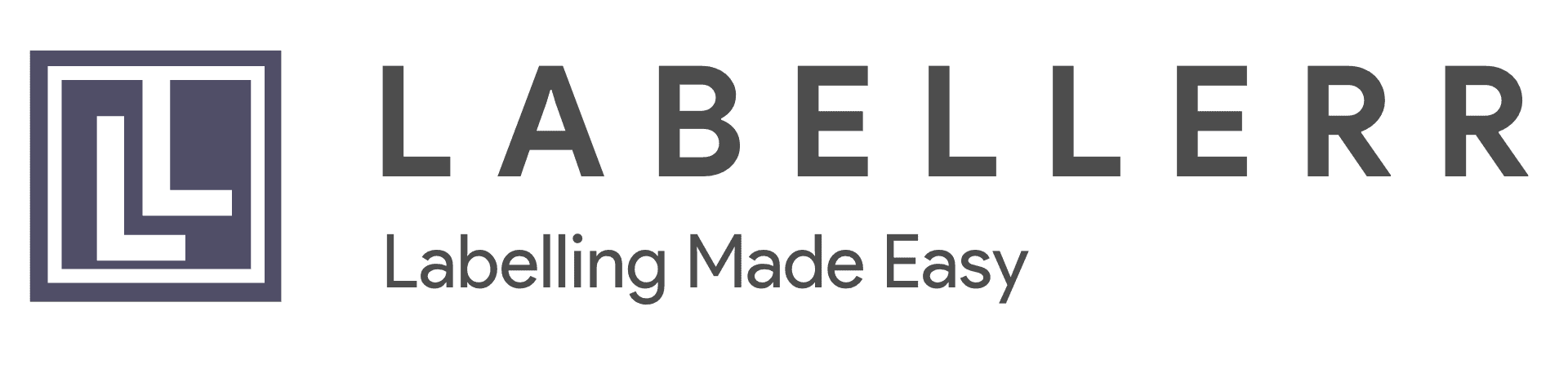
Labellerr is a text annotation and labeling service provider designed to support a range of machine learning and natural language processing (NLP) applications.
It also has its labeling tool with a user-friendly interface, robust feature set, and support for various text annotation tasks, such as text classification, named entity recognition, and sentiment analysis.
Labellerr caters to both individual developers and enterprise teams, offering a scalable solution for text labeling projects of varying complexity.
One of Labellerr's key features is its flexible workflow design, allowing users to create customized annotation processes that fit their specific project needs.
The platform provides a rich set of tools for annotators, including highlighting, tagging, and categorization, with options to review and approve annotations to ensure quality control.
It provide large pool of experts like language experts, medical experts and other industry expert to perform text annotation tasks.
2) iMerit
iMerit stands out as a leading provider of text annotation and labeling services, renowned for its accuracy, versatility, and scalability.
The company specializes in various aspects of text annotation, including entity recognition, sentiment analysis, content categorization, and more.
With its services deployed across a range of industries, from e-commerce and social media to healthcare and finance, iMerit is known for its ability to deliver high-quality annotated data to power sophisticated machine learning models.
The company uses a unique combination of technology and human expertise to ensure accurate text annotation, providing clients with the essential data they need to drive AI and NLP projects.
3) Hive
Hive positions itself as a strong contender in the text annotation and labeling arena, offering a compelling blend of human expertise and technological prowess.
They have a massive pool of over 5 million registered workers, ensuring access to a diverse range of linguistic skills for tackling your project.
This translates to efficient handling of large text datasets while maintaining accuracy. Hive's secret weapon lies in its robust quality control mechanisms.
They employ a multi-annotator approach for verification, followed by meticulous QA procedures.
This ensures the consistency and reliability of the labeled data, ultimately empowering you with high-quality training data to fuel your next-generation language models.
4) Cloudfactory
CloudFactory is recognized as a premier provider of text annotation and labeling services, offering a comprehensive suite of solutions to meet the data needs of AI and machine learning projects.
With a global network of highly trained data analysts, CloudFactory is capable of handling complex annotation tasks with accuracy and efficiency.
The company's approach combines human intelligence with advanced technology, ensuring that the annotated data is not only precise but also contextualized to meet specific client requirements.
Whether it's sentiment analysis, named entity recognition, or text categorization, CloudFactory's flexible and scalable services make it a trusted partner for AI-driven enterprises.
5) Cogito Tech
Cogito is a prominent object detection annotation service provider that has established itself as a leader in delivering high-quality, scalable data annotation solutions.
The company specializes in creating annotated datasets for a variety of computer vision applications, from autonomous vehicles and surveillance systems to medical imaging and retail analytics.
Cogito's object detection annotation services are designed to accurately label objects within images and videos, enabling clients to train robust machine learning models.
The company's blend of advanced technology and experienced annotators ensures that each project meets stringent quality and accuracy standards.
6. Scale AI
Scale AI is a leading data labeling platform specializing in providing high-quality annotations for machine learning projects.
The platform supports various data types, including text, images, and audio. For text annotation, Scale AI offers services such as named entity recognition, sentiment analysis, and transcription.
Scale AI's key selling point is its emphasis on accuracy and consistency, with rigorous quality control measures to ensure high-quality annotations.
The platform employs a combination of human annotators and automated tools to deliver precise results at scale.
Scale AI is known for its flexibility, allowing customers to customize their annotation workflows and define specific quality standards. It also offers detailed analytics and reporting tools to track project progress and identify areas for improvement.
These features make Scale AI an attractive choice for organizations seeking a reliable and scalable text annotation solution.
7) Appen
Appen comes as a leader in the text annotation and labeling services domain. Their extensive experience, spanning over two decades, translates into a deep understanding of the text data.
Appen boasts a global workforce with diverse linguistic and cultural backgrounds. This ensures that your text annotation projects, whether involving sentiment analysis, intent classification, or any other specialized task, are handled by annotators with the requisite knowledge and cultural understanding.
This meticulous approach guarantees high-quality, relevant training data that effectively fuels the development of your cutting-edge NLP applications.
Conclusion
In conclusion, selecting the right text annotation and labeling service provider is a crucial step in building robust and effective machine learning models, particularly in the domain of natural language processing (NLP).
The quality of annotated data can significantly impact model accuracy and performance, making it essential to choose a platform that aligns with your project's unique needs and goals.
As you explore different service providers, consider key features such as flexibility, collaboration tools, quality control, automation, integration capabilities, security, usability, scalability, and customer support.
A comprehensive assessment of these factors will guide you toward a platform that not only meets your immediate requirements but also offers the scalability to grow with your future projects.
Each service provider has its strengths, whether it's an open-source platform that offers customization and adaptability or a more structured solution with built-in quality controls and automation. The best choice depends on your specific context, including the complexity of your projects, team size, and security requirements.
Read our other listicles:
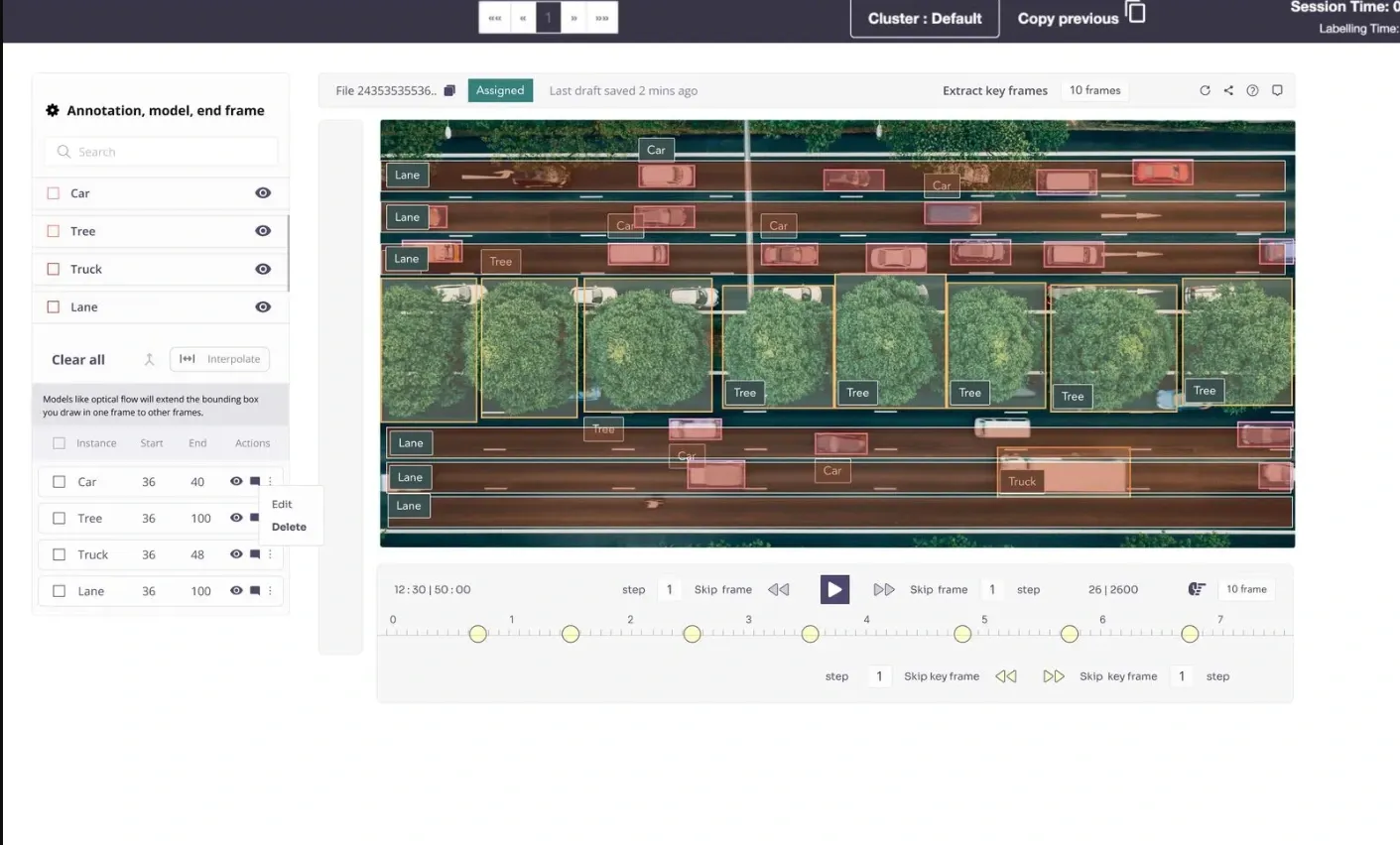
Frequently Asked Questions
1) What is text annotation, and why is it important?
Text annotation is the process of adding labels, tags, or metadata to text data to make it easier for machine learning models to understand and analyze. It is crucial because high-quality annotations are fundamental to training accurate and reliable models, particularly in natural language processing (NLP) applications.
2) What types of tasks can be accomplished with text annotation services?
Text annotation services can be used for a variety of tasks, including text classification, named entity recognition, sentiment analysis, entity linking, dependency parsing, transcription, and more. The specific tasks depend on the goals of your machine learning project.
3) What should I consider when choosing a text annotation and labeling service provider?
Key factors to consider include flexibility and customization, collaboration and team management, quality control, automation features, integration capabilities, security and data privacy, usability, scalability, and customer support. These factors will help you determine which platform best suits your needs.
Book our demo with one of our product specialist
Book a Demo