Synthetic data vs real data: which is a better option?
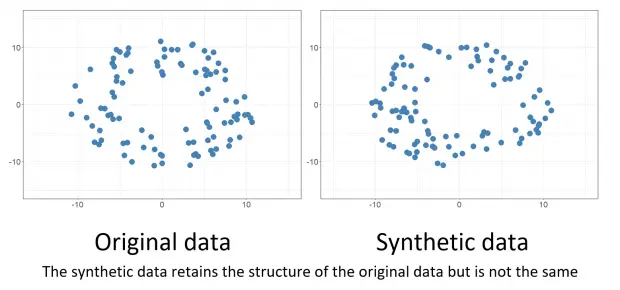
The development of driver-less vehicles is advancing. Modern AI is being used by medical institutions to enhance patient outcomes. Additionally, financial organizations are changing how they manage risk. Data continues to influence every element of human life, from the way we live to the way we work. And this is only the start.
The growth of data-driven AI is not, however, without significant difficulties. It is a difficult and frequently imprecise effort to locate, validate, and occasionally generate data for machine learning. How can data scientists obtain the data they require, at the size they require, without sacrificing accuracy, balance, or quality? Do they utilize real-world data or synthetic data?
Here we have presented in detail, which is ideal for your project. Let’s first differentiate between real-world data and synthetic data.
Real-world data
Image Source-IQVIA
A collection of real-world data recordings that describe a felt event is known as a real-world dataset. It may fall into one of two categories: small real-world datasets or large real-world datasets. You can obtain real-world data from Google Dataset Search, Kaggle and various government websites Real data might include, for instance, data that is a ten-year-old backup of an active system that contains information on actual people, matters, or instances.
Synthetic data
Image Source-Researchgate
Synthetic data is information that is produced artificially rather than by genuine methods. It is frequently developed with the use of algorithms and is applied to a variety of tasks, such as testing data for new tools and products, model validation, and training AI models. One sort of data augmentation is synthetic data.
Variation in synthetic data
In order to conceal sensitive personal information and preserve statistical details of features in the original data, synthetic data is generated at random. Three categories can be used to broadly classify synthetic data:
There are various types of Synthetic data ranging from Fully synthetic data, Partially synthetic data and Hybrid Synthetic.
- Fully synthetic data: This data is entirely generated; no authentic data are there. As a result, it is nearly impossible to re-identify any particular unit, yet all parameters are still completely available.
- Partially synthetic data: Only sensitive data is substituted with synthetic datasets; the rest is partially synthetic. The imputation model must be heavily relied upon for this. Due to the real values that are still there in the dataset, this results in a reduction in model dependence, but it also means that some exposure is feasible.
- Hybrid Synthetic: Data that is both real and synthetic are combined to create hybrid synthetic data. The fundamental distribution of the original data is examined, and the nearest neighbor of every data point is created while ensuring the relationship and consistency between several variables in the dataset. To create hybrid data, a near-record from the synthetic data is selected for each record from the real data.
Real data vs synthetic data
Image source- CIO Insight
Why real data is not preferred by users?
More than advantages, real data has more disadvantages and you might not prefer it over Synthetic data.
Real world data is difficult to collect
It can be hard to gather real data. AI for driver-less vehicles, for instance, cannot solely rely on real-world data. Companies developing this technology, like Waymo from Alphabet, must run simulations.
Consider this: you require training data on collisions in order to teach an AI to avoid an automobile accident. But collecting massive datasets of actual auto accidents is simply too risky and costly, so you simulate collisions instead.
Real data is rare
Data that can only be collected very seldom can likewise be subject to the principle of risky collection. While, Synthetic data can produce unusual events in enough quantity to correctly train an AI model, for instance, if your AI system is searching for a "needle in a haystack."
Take into account that some of the advantageous applications of AI are centred on "unusual" events. Rare events are difficult to record because of the complexity of these issues. Returning to the automobile example, you hardly ever have the opportunity to get this data because car wrecks don't happen very frequently. You can decide how many failures you wish to mimic using synthetic data.
When working with real-world data, data engineers have to:
- Ensure discretion and privacy.
- Label data consistently.
- Remove duplicate records and incorrect records from the data.
- assemble information from several sources, frequently in different formats
How does synthetic data win over real data?
Many companies are opting for synthetic data over real data due to the fact that it comes with various benefits. Here are some benefits of synthetic data.
Synthetic data provides full user control
In a simulation using synthetic data, everything is controllable. It has both blessings and drawbacks.
Because there are instances where edge circumstances that can be recorded in real datasets are missed by synthetic data, it can be a curse. You might wish to use transfer learning for these applications to mix some actual data with the synthetic datasets.
However, this is also a benefit because you may choose the event frequency, item distribution, and much more.
Annotation with synthetic data is intact
The flawless annotation offered by synthetic data is another benefit. Never again will manual data collection be necessary.
Each object in a scene can automatically create a variety of annotations. This might not seem like a significant concern, but it's a major factor in the low cost of synthetic data in comparison to real-world data. The labelling of data is free. Instead, the initial expenditure in creating the simulation is the primary cost of using synthetic data. The cost-effectiveness of creating data over genuine data increases rapidly after that.
Synthetic data is generally multispectral
Companies that manufacture autonomous vehicles have learned how difficult it is to annotate non-visible data. They have therefore been among the strongest supporters of synthetic data.
Many businesses produce fictitious LiDAR data using simulations. Because it is synthetic, the data is already categorized and the ground truth is known. For computer vision applications using infrared or radar imaging, when humans can't completely interpret the imagery, synthetic data works well.
How organizations are utilizing synthetic data?
Synthetic testing data is more flexible, scalable, and realistic than regulation test data and is simpler to create for testing. The development of software and data-driven testing both depend on this data.
Training AI/ML models — The training of AI models increasingly uses artificial data. Real data can be supplemented using data synthesis, which can upsample uncommon events or patterns and improve algorithm training. In general, synthetic training data outperforms real-world data and is essential for creating superior AI models.
Synthetic data for governance helps eliminate biases in real-world data. In order to stress test an AI model with data sets that hardly appear in the real world, the dataset is also helpful. Artificial data is necessary for AI that can be explained and offers an understanding of model behavior.
With Labellerr’s SaaS data annotation platform, you can effortlessly annotate, manage, and generate high-quality datasets tailored to your needs. Sign up for a free Researcher Plan and see how Labellerr can accelerate your machine learning workflow!
Conclusion
Synthetic data production is quicker, more adaptable, and more scalable when compared to real-world data. It can also serve as a useful tool for modelling and creating data that doesn't actually exist in the world by changing the parameters.
Data scientists may provide machine learning algorithms with data to reflect any condition using synthetic data. Synthetic test data is a great technique to test a theory or predict numerous outcomes since it can reflect "what if" possibilities.
If you find this blog interesting, then stay in touch with us for more information!

Simplify Your Data Annotation Workflow With Proven Strategies
.png)
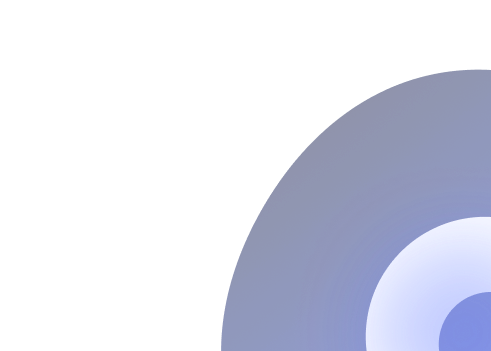