Oil Rig Safety Analysis Using Computer Vision
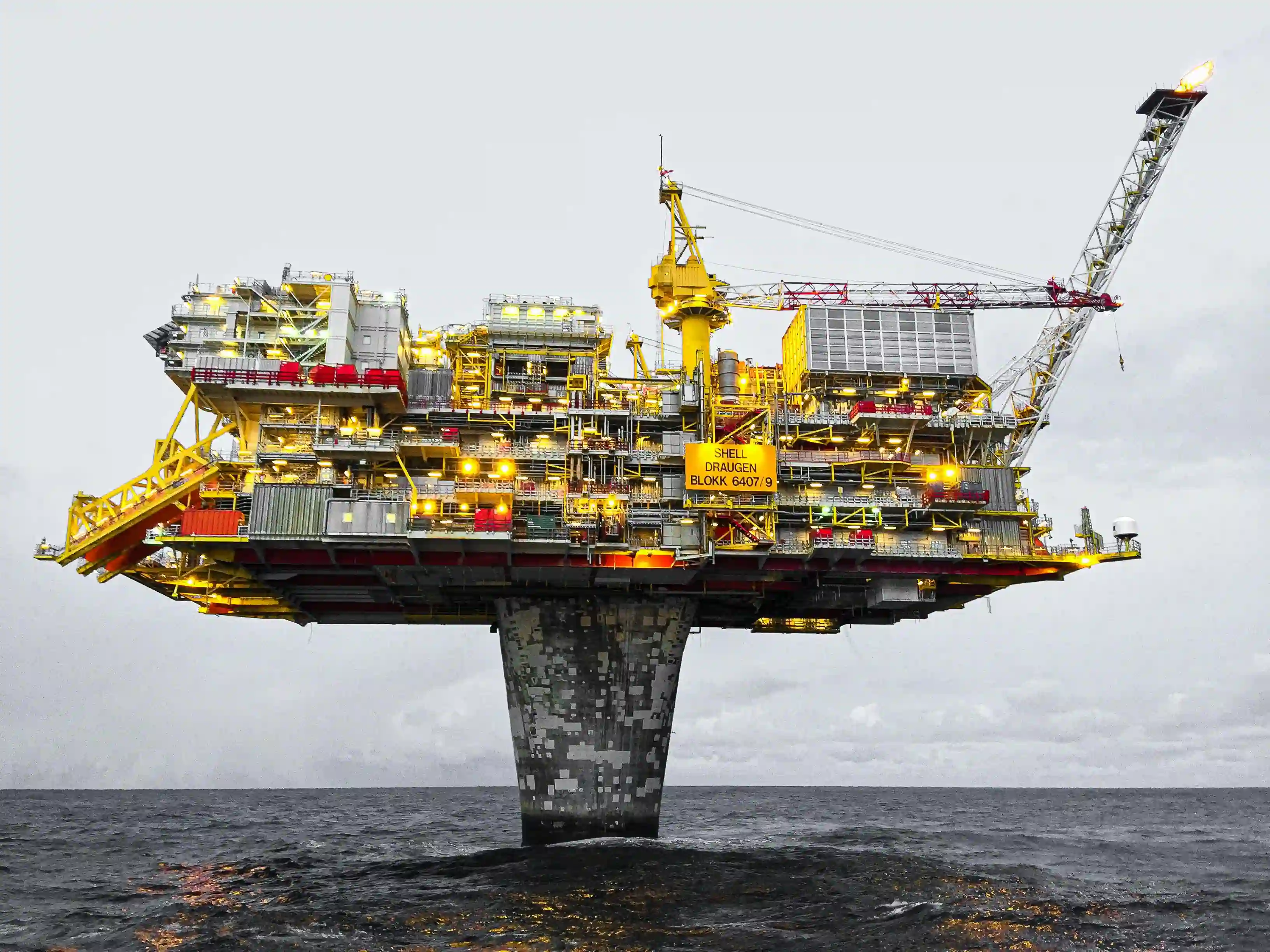
Introduction:
Offshore operations pose inherent risks due to harsh environmental conditions, volatile seas, and complex machinery. Workers face equipment malfunctions, hazardous material exposure, and potential accidents.
Coordination among personnel, constant monitoring of equipment, and adherence to safety protocols are vital.
According to the US Bureau of Labor Statistics, the fatality rate for oil and gas extraction workers is nearly eight times higher than the average for all industries. In addition to saving lives, safety analysis also minimizes operational disruptions, prevents environmental damage, and safeguards company reputation.
Table of Contents
- Predictive Maintenance
- Integration of IoT Devices
- Safety Monitoring
- Emergency Response Planning
- Drilling Optimization
- Environmental Monitoring
- Supply Chain Optimization
- Remote Operations
- Conclusion
- FAQ
Predictive Maintenance
Predictive Maintenance refers to the use of AI and analytics to predict equipment failures and thus ensuring prompt and timely maintenance. Machine learning algorithms analyze vast streams of sensor data, including temperature, pressure, and vibration readings, to detect subtle patterns indicative of potential tear and breakdown of machinery.
Advanced AI techniques like supervised learning, recurrent neural networks, and anomaly detection algorithms are used. This approach ensures the smooth operation of oil rigs while maximizing asset lifespan.
Integration with IoT Devices
By integrating IoT devices with AI, oil rig operations transform into a cohesive ecosystem. This enhances operational efficiency and empowers informed decision-making. This also enables remote monitoring and control of critical devices.
IoT connectivity facilitates condition-based maintenance, allowing for targeted interventions and resource optimization. Ultimately, this revolutionizes management in challenging offshore environments.
Strengthening Worker Safety
Utilizing AI-driven solutions, organizations can implement personalized safety protocols tailored to individual workers' needs and working conditions, fostering a safer and more supportive environment.
These solutions can include wearable devices to detect signs of fatigue, stress, or other health-related issues. Moreover, AI algorithms can optimize work schedules and task assignments to minimize physical strain and fatigue, ultimately enhancing worker well-being and productivity.
By prioritizing employees' holistic health and safety, AI-driven solutions cultivate a culture of care and support within the workplace. This proactive approach not only minimizes the risk of accidents but also fosters a collaborative environment where workers feel valued and protected.
Emergency Response Planning
Emergency response planning involves preparing for and mitigating the impact of unforeseen events or crises on oil rigs. AI enhances this by analyzing vast amounts of data to simulate various emergency scenarios and develop optimized response plans.
AI algorithms such as genetic algorithms, Monte Carlo simulations, and reinforcement learning are employed to model different emergency scenarios and predict potential outcomes. Tools like simulation software and decision support systems leverage these algorithms to generate realistic simulations and recommend effective response strategies.
Drilling Optimization
Drilling optimization involves maximizing the efficiency and productivity of drilling operations on oil rigs. AI plays a pivotal role in enhancing drilling optimization by analyzing geological data and optimizing drilling parameters. Support vector machines, artificial neural networks, and decision trees, are deployed to predict optimal drilling locations and trajectories.
Drilling simulators enable engineers to simulate various scenarios and identify the most efficient drilling strategies. Additionally, advanced data analytics techniques, like data fusion, are utilized to continuously monitor drilling performance and identify areas for improvement. With this oil rig operators can achieve higher drilling rates and maximize resource extraction while minimizing environmental impact.
Environmental Monitoring
Environmental monitoring involves collecting and analyzing data on atmospheric conditions. AI technologies provide accurate predictions, early warnings, and insights into weather patterns, aiding in decision-making and risk management on oil rigs.
AI-driven weather forecasting models leverage recurrent neural networks (RNNs) and convolutional neural networks (CNNs), to analyze vast amounts of historical weather data and current atmospheric conditions. These models enable oil rig operators to anticipate adverse weather events, such as storms or hurricanes, well in advance.
By integrating AI into environmental monitoring practices, oil rig operators can and enhance overall safety and efficiency in offshore environments.
Supply Chain Optimization
Supply chain optimization entails the strategic management of the flow of goods, services, and information from suppliers to customers to maximize efficiency and minimize costs.
Demand forecasting models, inventory optimization algorithms, and route optimization algorithms, are employed to predict demand, optimize inventory levels, and streamline transportation routes. These algorithms analyze historical sales data, market trends, and other relevant factors to accurately forecast demand and ensure the availability of products at the right place and time.
This proactive approach enables organizations to respond effectively to changing market dynamics and ensure the seamless flow of materials and resources to support offshore operations.
Remote Operations
Remote operations involve the management and control of oil rig activities from a centralized location, often using advanced technologies such as AI, robotics, and telecommunications. This approach enables operators to monitor and manage rig operations remotely, reducing the need for on-site personnel and enhancing operational efficiency and safety.
Robotics and AI automation technologies are used to perform tasks traditionally carried out by human workers, such as inspection, maintenance, and repairs. Autonomous vehicles, and remotely operated robots navigate the rig environment, perform inspections, and execute maintenance tasks under the supervision of remote operators.
Conclusion
In conclusion, the integration of AI technologies in the oiling industry has ushered in a new era of efficiency, safety, and productivity.
Frequently Asked Questions
How is Artificial Intelligence used in the oil industry
Artificial Intelligence (AI) in the oil industry optimizes drilling processes, predicts equipment failures, and analyzes seismic data for exploration. Machine learning algorithms enhance reservoir modeling, improving extraction efficiency and reducing costs.
How is AI used in safety management
AI enhances safety management by analyzing data from sensors and cameras to detect hazards in real-time. It predicts potential safety incidents, allowing proactive measures to prevent accidents. Machine learning algorithms analyze historical safety data to identify patterns and improve safety protocols.
How can AI transform industrial safety
AI transforms industrial safety by integrating data from various sources to provide a holistic view of safety risks. Predictive analytics identify potential hazards and recommend preventive measures. AI-powered safety monitoring systems continuously assess worker behavior and environmental conditions, enabling real-time interventions to mitigate risks.
How is Artificial Intelligence used in petroleum engineering.
In petroleum engineering, AI optimizes reservoir management through predictive modeling and simulation. Machine learning algorithms analyze geological data to identify optimal drilling locations and extraction techniques. AI-driven systems enhance well performance monitoring and production optimization. Deep learning techniques improve seismic interpretation accuracy, aiding in reservoir characterization and enhanced oil recovery strategies.

Simplify Your Data Annotation Workflow With Proven Strategies
.png)
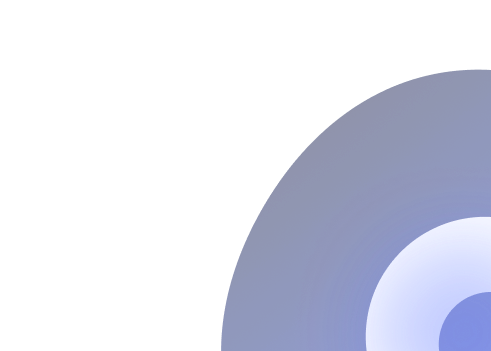