Accelerate Ultrasound Imaging Annotation With Labellerr Using SAM
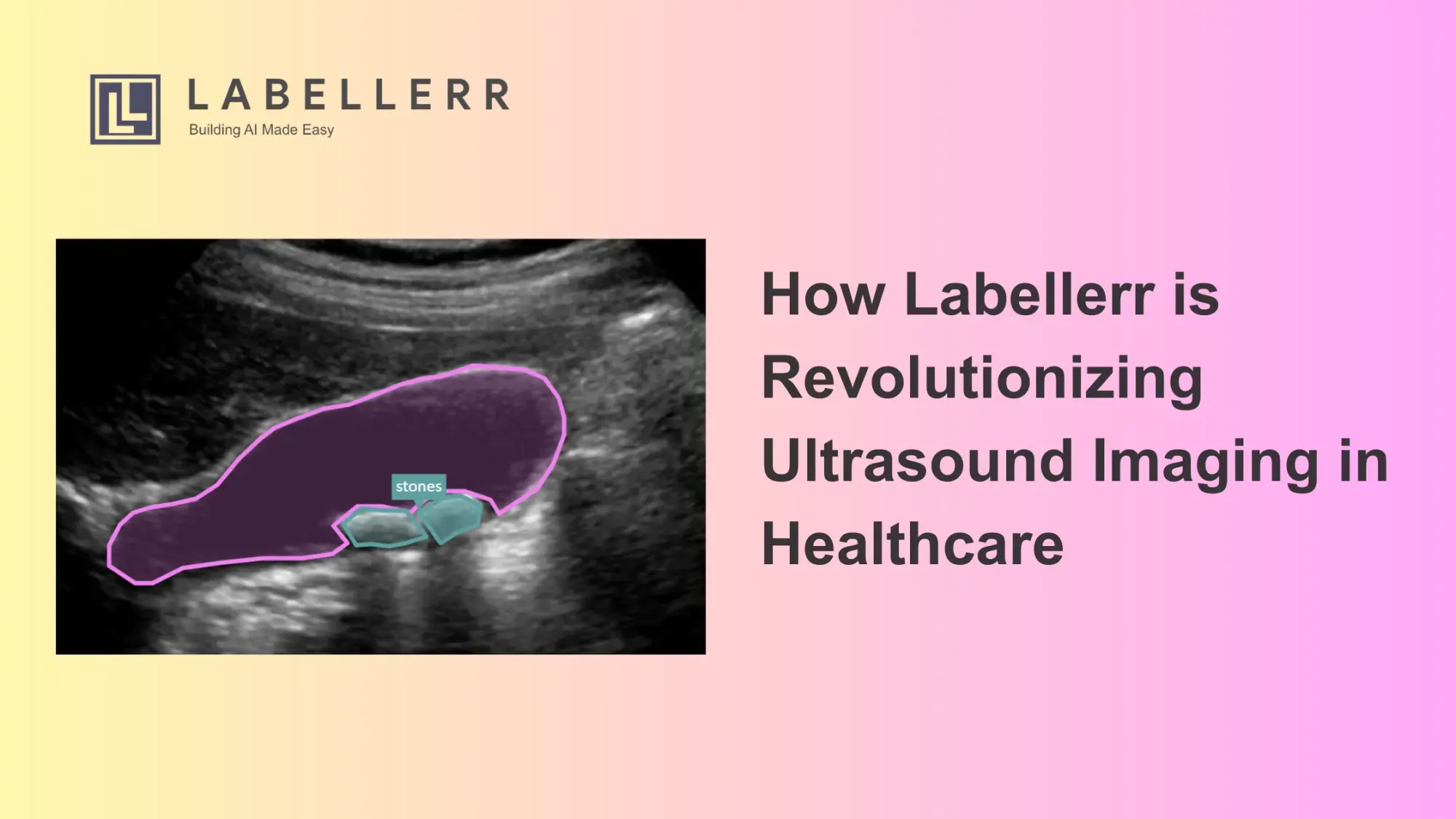
Introduction
In today's world of healthcare innovation, the development of AI-powered image analysis software holds immense promise for revolutionizing medical diagnostics.
Among many imaging modalities, ultrasound stands out for its versatility and non-invasive nature offering valuable insights into a wide range of medical conditions.
However, harnessing the full potential of ultrasound imaging requires overcoming significant challenges in annotating complex ultrasound images for model training.
This sets the stage for exploring how annotation platforms like Labellerr play a crucial role in addressing these challenges, facilitating the development of advanced AI-powered ultrasound technologies that enhance patient care and outcomes.
Challenge
Annotating ultrasound images for model training presents significant challenges due to the complexity of the structures involved.
Unlike other medical imaging modalities, ultrasound images lack clear boundaries, making it difficult for annotators to accurately delineate relevant structures.
This inherent complexity slows down the annotation process and increases the likelihood of errors, hindering the development of AI-powered image analysis software in the healthcare sector.
Solution: Labellerr's Role
To address these challenges, healthcare companies and researchers employ annotation platforms like Labellerr to streamline the annotation process for ultrasound images.
By harnessing Labellerr's capabilities, they can annotate sequential and non-sequential ultrasound data with increased speed and accuracy, thereby doubling their release velocity for developing AI-powered image analysis software.
Read the full documentation for using SAM in an annotation workflow with Labellerr.
This improved efficiency enables medical institutions worldwide to benefit from more advanced and reliable diagnostic tools, ultimately enhancing patient care and outcomes.
1. Cost Savings
Implementing Labellerr for ultrasound annotation results in substantial cost savings for healthcare institutions and researchers.
By optimizing the annotation process, Labellerr ensures efficient resource allocation, minimizing unnecessary expenditures while maximizing the value derived from annotated ultrasound data.
2. Reduced Turnaround Time
Labellerr significantly accelerates the ultrasound annotation process, leading to a notable reduction in turnaround time for training data pipelines.
Its advanced annotation tools and automation features streamline the workflow, allowing healthcare professionals and researchers to analyze ultrasound data more rapidly and efficiently.
3. Quality Control
Ensuring the accuracy and reliability of annotated ultrasound data is paramount for the development of robust AI-powered ultrasound technologies.
Labellerr integrates rigorous quality control mechanisms to validate the precision of annotated images, enhancing the overall trustworthiness and effectiveness of ultrasound analysis software.
4. Scale Up and Volume
Labellerr's scalability enables seamless handling of large volumes of ultrasound data, empowering healthcare institutions and researchers to scale up their ultrasound annotation projects as needed.
Whether dealing with extensive datasets or increasing workload demands, Labellerr can efficiently accommodate the scalability requirements of ultrasound annotation initiatives.
5. Custom Workflows
Labellerr offers customizable workflows tailored to the specific needs of ultrasound annotation projects.
This flexibility allows stakeholders to design annotation processes that align precisely with the objectives and requirements of their ultrasound analysis, resulting in more accurate and tailored solutions.
6. Foundation Model-Based Labeling
Leveraging advanced foundation models, Labellerr accelerates the ultrasound annotation process by enhancing the understanding of ultrasound images.
By leveraging state-of-the-art technology, Labellerr improves the accuracy and efficiency of ultrasound labeling tasks, thereby facilitating the development of more advanced ultrasound analysis software.
7. Active Learning-Based Labeling
Labellerr employs active learning techniques to continuously enhance the accuracy of ultrasound annotations over time.
This dynamic approach ensures that ultrasound analysis models become progressively more adept at identifying and interpreting ultrasound images, leading to more precise diagnostic outcomes and treatment recommendations.
8. Automated QA (Quality Assurance)
Labellerr automates quality assurance processes to minimize errors and inconsistencies in annotated ultrasound data.
By implementing automated QA mechanisms, Labellerr enhances the reliability of ultrasound analysis software, ensuring that healthcare professionals can trust the insights derived from annotated ultrasound images.
9. Automated Import and Export of Data
Streamlining the data management process, Labellerr automates the import and export of ultrasound data, reducing manual efforts and saving time for healthcare professionals and researchers.
This automation enhances the efficiency of data management tasks, enabling stakeholders to focus more on analyzing and interpreting ultrasound data.
10. Collaborative Annotation Pipeline
Labellerr facilitates collaboration among multiple users with different roles in the ultrasound annotation process.
This collaborative approach ensures that diverse perspectives and expertise contribute to the annotation process, leading to more comprehensive and accurate insights into ultrasound images and improving patient care outcomes.
Conclusion
The integration of advanced annotation technologies like Labellerr into the healthcare sector revolutionizes the development of AI-powered image analysis software for ultrasound imaging.
By overcoming the challenges associated with annotating complex ultrasound images, companies and researchers can accelerate innovation in medical diagnostics, ultimately improving patient care and advancing healthcare practices globally.
Frequently Asked Questions
1. How Labellerr help ML teams build computer vision AI 10x faster?
Labellerr expedites the process of building computer vision AI for ML teams by offering a comprehensive annotation platform that accelerates image labeling tasks.
Through its efficient workflows, scalable infrastructure, and advanced foundation models, Labellerr enables teams to annotate images 10 times faster, significantly reducing turnaround time.
Its automated quality assurance, active learning capabilities, and collaborative annotation pipeline further streamline the development process, ensuring rapid and accurate model training for computer vision applications.
2. How computer-aided diagnosis can improve the accuracy of ultrasound diagnosis?
Computer-aided diagnosis (CAD) enhances the accuracy of ultrasound diagnosis by providing additional support to healthcare professionals in interpreting ultrasound images.
CAD systems utilize advanced image processing algorithms and machine learning techniques to analyze ultrasound images, helping to identify subtle abnormalities or patterns that may be missed by the human eye alone.
By integrating CAD into the diagnostic workflow, healthcare providers can benefit from improved accuracy, faster diagnosis, and potentially earlier detection of diseases or conditions, leading to more effective patient management and treatment strategies.
Book our demo with one of our product specialist
Book a Demo