Enhancing Wound Image Segmentation with Labellerr
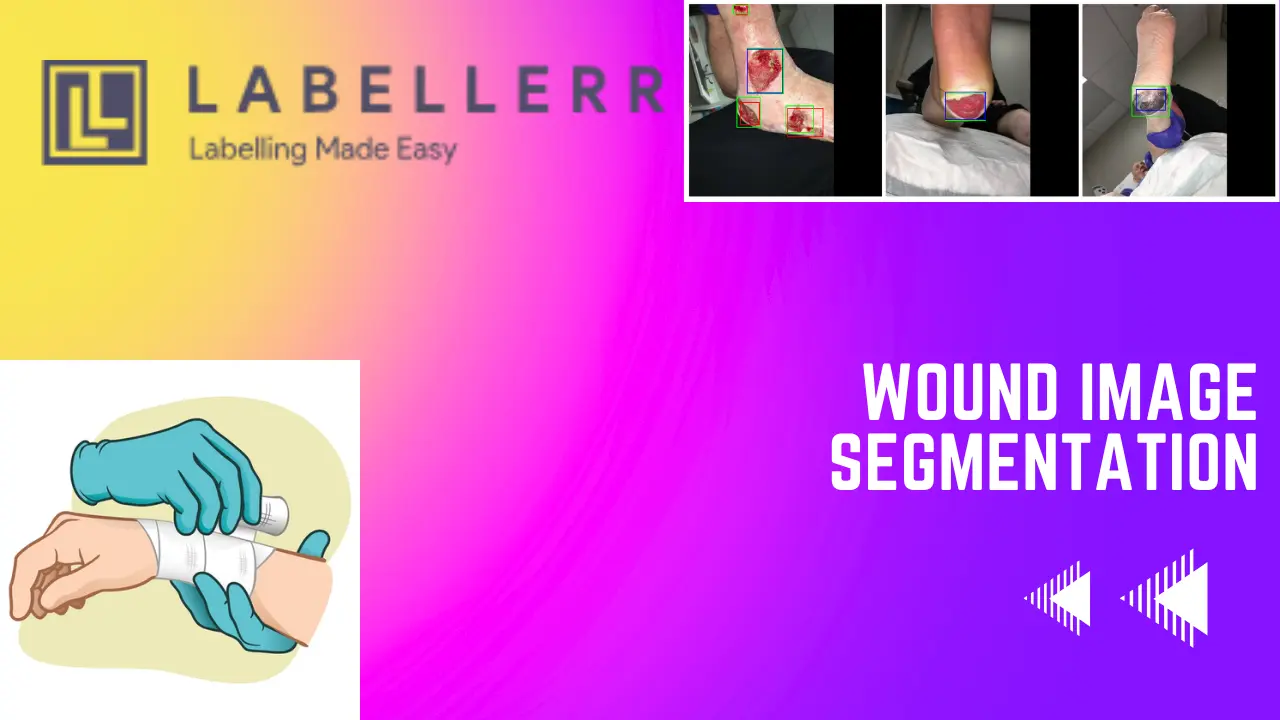
Wound segmentation using computer vision technology has emerged as a valuable tool in medical imaging for precise diagnosis and treatment planning.
Accurate segmentation of wounds from medical images, such as photographs or scans, enables healthcare professionals to assess the size, shape, and severity of wounds with greater precision.
This information plays a crucial role in determining appropriate treatment strategies and monitoring wound healing progress over time.
However, manual segmentation of wounds from medical images is a labor-intensive and time-consuming task, prone to subjectivity and variability among annotators.
To address these challenges, the integration of Labellerr, a leading data annotation platform, offers a solution for automating and standardizing the annotation process.
By leveraging Labellerr's advanced annotation tools and robust segmentation features, healthcare professionals can streamline the annotation of wound images, enhancing efficiency and accuracy in wound segmentation tasks.
Challenge
Segmenting wounds from medical images poses several challenges that can hinder the accuracy and efficiency of the annotation process.
Firstly, wounds come in various shapes, sizes, and textures, making it difficult to develop a one-size-fits-all segmentation approach.
In wound segmentation using computer vision, annotated classes include Background, Limb, Wound bed, Granulation, Slough, and Eschar.
Background represents non-limb or non-wound areas, while limb denotes human limbs for reference.
The wound bed signifies the general wound area, granulation indicates healing tissue in shades of red, slough represents yellow necrotic tissue, and eschar denotes black/brown necrotic tissue.
These annotations enable accurate segmentation, which is crucial for precise wound analysis in clinical contexts.
The complexity of wound morphology, including irregular edges, overlapping tissues, and varying degrees of tissue damage, further complicates the segmentation task.
Additionally, manual segmentation by healthcare professionals is time-consuming and subjective, leading to inconsistencies and inter-annotator variability.
These challenges present the need for advanced segmentation algorithms and automated annotation tools, such as those provided by Labellerr, to improve the efficiency and accuracy of wound segmentation in medical imaging.
Solution Using Labellerr for Wound Image Segmentation
Intuitive Interface
Labellerr offers an intuitive interface designed to streamline the annotation process for wound segmentation.
With user-friendly tools and clear labeling options, annotators can easily identify and delineate wound regions in medical images.
The intuitive interface minimizes the learning curve, enabling annotators to efficiently annotate wound areas without being hindered by complex annotation tools.
This user-friendly interface enhances productivity and ensures consistency in the segmentation process.
Cost Savings
Labellerr provides significant cost savings for healthcare facilities by optimizing the annotation process for wound segmentation.
By automating repetitive tasks and streamlining workflow management, Labellerr reduces the time and resources required for manual annotation.
This results in cost savings associated with labor expenses and improves the overall efficiency of the wound segmentation process.
Additionally, Labellerr's pay-per-task pricing model ensures cost-effectiveness by allowing healthcare facilities to pay only for the annotations they need.
Robust Segmentation Features
Labellerr incorporates robust segmentation features, including the advanced Segment Anything Model, specifically tailored for wound segmentation tasks.
This model excels at accurately delineating wound boundaries, even in cases of irregular shapes, overlapping tissues, and varying textures.
By leveraging state-of-the-art segmentation algorithms, Labellerr ensures precise and consistent segmentation results, essential for accurate wound assessment and treatment planning.
The robust segmentation capabilities of Labellerr enhance the quality and reliability of annotated data, ultimately improving the performance of wound segmentation models in medical imaging applications.
Active Learning Based Labeling
Labellerr implements active learning techniques to optimize the annotation process for wound segmentation.
By intelligently selecting the most informative image regions for annotation, Labellerr maximizes the efficiency of data labeling, reducing manual effort while improving the performance of trained models.
This active learning-based approach enables annotators to prioritize labeling efforts on areas that are most beneficial for model training, ultimately leading to more accurate and effective wound segmentation models.
Automated Import and Export of Data
Labellerr streamlines the process of importing and exporting data for wound segmentation tasks with its automated functionalities.
Whether integrating data from medical imaging devices, electronic health records, or other sources, Labellerr simplifies the data management process, enabling seamless integration of images into the annotation platform.
Similarly, Labellerr facilitates the export of annotated data, ensuring compatibility with various medical imaging systems and analysis tools for further processing.
Collaborative Annotation Pipeline
Labellerr fosters collaboration among healthcare professionals and annotators with its collaborative annotation pipeline.
Multiple users can work simultaneously on annotating wound images, enabling distributed workflows and real-time collaboration.
This collaborative approach enhances productivity and ensures consistency and accuracy in the labeled dataset, ultimately improving the performance of wound segmentation models.
Additionally, Labellerr's collaborative annotation pipeline facilitates knowledge sharing and expertise exchange among team members, further enhancing the quality and reliability of the annotated data.
Frequently Asked Questions
Q1) What is wound segmentation, and why is it important in medical imaging?
Wound segmentation is the process of identifying and outline the boundaries of wounds in medical images, such as photographs or scans. It is essential in medical imaging for precise diagnosis, treatment planning, and monitoring of wound healing progress.
Q2) How does Labellerr assist in wound segmentation tasks?
Labellerr provides advanced tools and features specifically designed for wound segmentation, including an intuitive interface, robust segmentation algorithms, and active learning-based labeling techniques.
These functionalities streamline the annotation process and improve the efficiency and accuracy of wound segmentation tasks.
Book our demo with one of our product specialist
Book a Demo