Accelerate Retail Product Recognition Annotations with Labellerr Using SAM
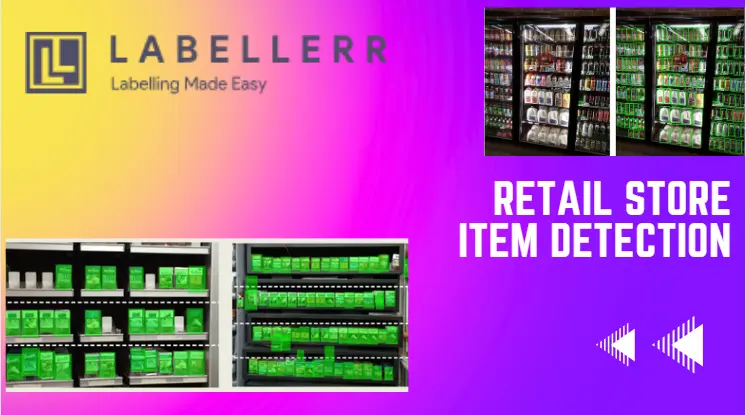
Table of Contents
- Introduction
- Challenge
- Solution: Labellerr's Role in Product Recognition
- Conclusion
- Frequently Asked Questions
Introduction
In the retail industry, the ability to accurately identify and recognize products on store shelves is important for inventory management, shelf monitoring, and improving the overall shopping experience.
According to an IHL survey, over 30% of consumers find empty shelves while shopping. This has an influence on both consumer satisfaction and the retailer's profitability owing to missed sales. Retailers who adopt product detection systems can ensure that shelves are refilled quickly, improving the shopping experience and potentially increasing sales.
Traditional methods of manually checking shelves for stock levels and product placement are time-consuming and prone to errors.
However, advancements in computer vision technology have made the way for automated solutions that can analyze images captured by in-store cameras and accurately identify products on shelves.
Annotating large volumes of shelf images with precise product labels is essential for training machine learning models to perform this task effectively.
Labellerr annotation platform plays an important role in annotating shelf images to create labeled datasets for training accurate product recognition models.
Challenge
Identifying and recognizing products on store shelves using computer vision presents a challenge in retail shops.
High Volume and Variety: The vast array of products stocked on shelves, each characterized by distinct shapes, sizes, colors, and packaging designs, complicates the task of accurate recognition, particularly when dealing with similar-looking items or products with ambiguous labeling.
Annotators may utilize hierarchical classification to train models with a hierarchical order. First, classify things into broad categories (e.g., beverages, electronics) and then subcategorize them into specific types (e.g., soda, smartphone) within those groups. This reduces the total number of unique products that the model must recognize at once.
Lighting Variations: Lighting conditions within retail spaces vary widely, ranging from bright overhead lights to dimly lit areas, introducing further complexity by altering the appearance of products and creating shadows or reflections.
Additionally, variations in scale across shelf images captured by in-store cameras require precise annotation to account for these differences, ensuring that the trained models can effectively generalize to diverse viewing angles.
Here, we will be dealing with complex shelf images crammed with various objects. The task involves meticulously outlining each individual product on the shelf, separating it from its neighbors and capturing its entirety.
This could include anything from toothpaste tubes to cereal boxes and clothing items. While this seems straightforward, the sheer number of objects and their close proximity present significant challenges for manual annotation.
Occlusions and Partial Views: Pinpoint accuracy with every click can be difficult, especially for products touching or partially overlapping. Inaccurate annotations can negatively impact product recognition models.
To deal with partial occulusions annotators may use either of the techniques:
Part-based detection: Train models to identify each element of a product (for example, a bottle cap or a brand) even when the complete object is not visible. This enables identification even with partial occlusions.
Contextual reasoning: Train models to use contextual information such as shelf layout, product proximity, and category information to generate accurate predictions about occluded products.
Overcoming these challenges demands advanced annotation methodologies and tools to streamline the process, ensure accuracy and consistency, and generate high-quality labeled datasets essential for training robust product recognition models.
Solution: Labellerr's Role in Product Product Recognition
To address these challenges, companies and researchers employ annotation platforms like Labellerr to streamline the annotation process.
By harnessing Labellerr's capabilities, they can annotate data with increased speed and accuracy, thereby doubling their release velocity for developing AI-powered image analysis software.
Read the full documentation for using SAM in an annotation workflow with Labellerr.
Below we discuss multiple factors by which Labellerr helps teams to overcome challenges.
Cost Savings
Labellerr offers significant cost savings for product recognition tasks on store shelves by optimizing the annotation process and reducing manual labor costs.
Its advanced automation features streamline the annotation workflow, minimizing the need for extensive human intervention.
By automating repetitive tasks and leveraging efficient annotation algorithms, Labellerr enables organizations to annotate large volumes of shelf images efficiently and cost-effectively, ultimately saving time and resources.
Intuitive Interface
Labellerr provides an intuitive user interface designed to simplify the annotation process for annotators working on product recognition tasks.
Its user-friendly design and navigation tools empower annotators to label products on store shelves with ease, reducing the learning curve and enhancing productivity.
With Labellerr's intuitive interface, annotators can focus on annotation tasks without being hindered by the complexity of the retail environment.
Robust Segmentation Features
Labellerr incorporates robust segmentation features, including the Segment Anything Model (SAM), which enables precise and accurate delineation of products within shelf images.
SAM's advanced algorithms can effectively segment diverse products, regardless of their shapes, sizes, colors, or packaging designs.
By leveraging SAM's segmentation capabilities, Labellerr ensures the accuracy and reliability of labeled datasets, enhancing the performance of product recognition models.
Custom Workflows
Labellerr supports customizable workflows tailored to the specific requirements of product recognition tasks on store shelves.
Organizations can define custom annotation protocols, designate product categories, and customize labeling criteria to suit their unique needs.
This flexibility ensures that annotations are consistent, standardized, and aligned with the objectives of the project, ultimately leading to more reliable and accurate labeled datasets for training product recognition models.
Active Learning Based Labeling
Labellerr employs active learning techniques to optimize the product recognition process on store shelves.
By intelligently selecting the most informative samples for annotation, Labellerr maximizes the efficiency of data labeling, reducing manual effort while improving the performance of trained models.
This active learning-based approach enables organizations to prioritize labeling efforts on data points that are most beneficial for model training, ultimately leading to more accurate and effective product recognition models.
Automated Import and Export of Data
Labellerr streamlines the process of importing and exporting data for product recognition tasks on store shelves with its automated functionalities.
Using various YOLO (You Only Look Once) models for object detection, organizations can seamlessly upload large volumes of shelf images onto the platform.
This integration with YOLO models enables efficient object detection and annotation, ensuring accurate labeling of products within shelf images.
Collaborative Annotation Pipeline
Labellerr facilitates collaboration among annotators with its collaborative annotation pipeline.
Multiple users can work simultaneously on annotating shelf images for product recognition, allowing for distributed workflows and real-time collaboration.
This collaborative approach enhances productivity and ensures consistency and accuracy in the labeled dataset, ultimately improving the performance of product recognition models.
Automated QA (Quality Assurance):
Labellerr integrates automated quality assurance mechanisms to ensure the accuracy and reliability of annotated product recognition data.
Advanced algorithms analyze annotations in real-time, flagging inconsistencies or errors for review by annotators.
This automated QA process maintains the quality of the labeled dataset, reducing the risk of errors and ensuring the high performance of trained models in product recognition tasks on store shelves.
Conclusion
In conclusion, Labellerr serves a tool for enhancing product recognition on store shelves through precise and efficient data annotation.
By addressing the challenges associated with annotating shelf images for product recognition tasks, Labellerr empowers organizations to create accurate and reliable datasets essential for training robust recognition models.
With its advanced features such as active learning-based labeling, integration with YOLO models for object detection, collaborative annotation pipeline, and automated quality assurance, Labellerr streamlines the annotation process, fosters collaboration among annotators, and ensures the quality and consistency of labeled data.
Labellers allow the development of more effective inventory management systems, shelf monitoring solutions, and customer shopping experiences.
Frequently Asked Questions
Q1) What is product recognition on store shelves, and why is it important?
Product recognition on store shelves involves using computer vision technology to identify and recognize products displayed on retail shelves.
It is essential for tasks such as inventory management, shelf monitoring, and enhancing the overall shopping experience for customers.
Q2) How does Labellerr assist in annotating shelf images for product recognition?
Labellerr provides advanced annotation tools and features specifically designed for annotating shelf images for product recognition tasks.
Its intuitive interface and active learning-based labeling streamline the annotation process, enabling annotators to label products accurately and efficiently.
References

Simplify Your Data Annotation Workflow With Proven Strategies
.png)
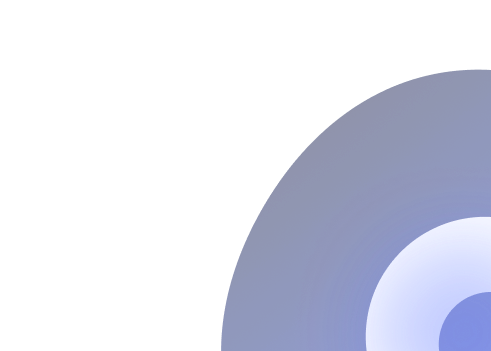