Accelerate Driver Monitoring Annotation with Labellerr
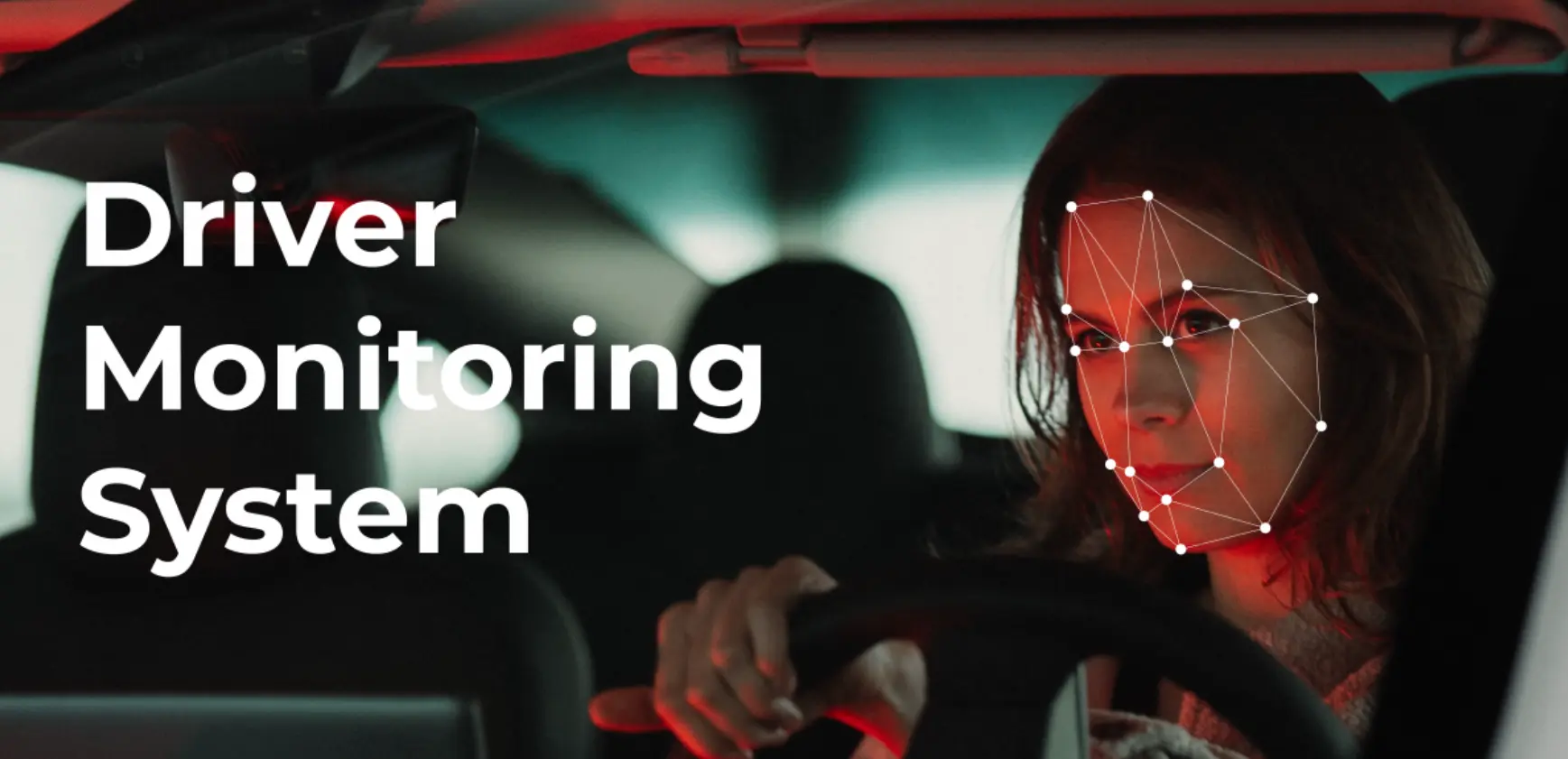
Table of Contents
Introduction
In the world of transportation, ensuring road safety is very important.
Driver monitoring systems play a crucial role in this endeavor by providing insights into driver behavior and attentiveness.
Companies and researchers are leveraging advanced technologies like computer vision and AI to develop innovative solutions for monitoring drivers in real-time.
These technologies enable the detection of potential distractions, fatigue, and other factors that may compromise road safety.
However, to achieve this, accurate labeling of driver behaviors and actions is essential, requiring sophisticated annotation tools like Labellerr.
Challenge
Monitoring drivers effectively poses significant challenges due to the dynamic nature of human behavior and the diverse range of factors that can influence driving performance.
Identifying subtle signs of distraction, fatigue, or impairment with the complexities of real-world driving scenarios can be difficult.
Traditional methods of driver monitoring, such as manual observation or self-reporting, are often unreliable and impractical for real-time applications.
Moreover, the vast volume of data generated by driver monitoring systems can overwhelm manual annotation processes, leading to delays and inaccuracies in data labeling.
Labellerr's Role in Driver Monitoring
To address these challenges, companies and researchers turn to annotation platforms like Labellerr to streamline the labeling process for driver monitoring data.
Labellerr's advanced annotation tools and automation features can accurately annotate driver behaviors and actions in real-time video feeds.
From detecting drowsiness to identifying distractions, Labellerr enables the rapid and precise labeling of critical driver-related events.
Its customizable workflows and scalable infrastructure further enhance efficiency, allowing stakeholders to analyze vast amounts of driver data with ease.
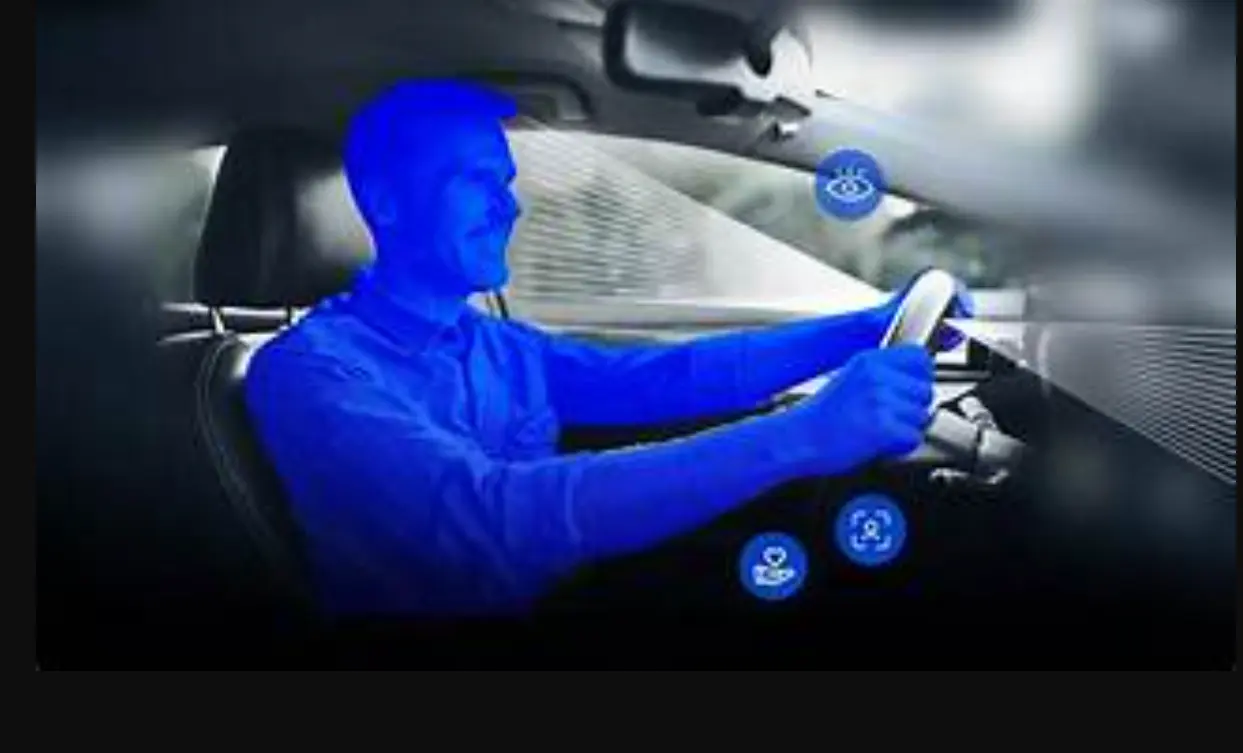
1. Cost Savings
Labellerr offers a cost-effective solution for driver monitoring projects by optimizing the annotation process and maximizing resource efficiency.
By reducing manual efforts and streamlining workflow automation, Labellerr helps companies and researchers achieve significant cost savings without compromising data quality or accuracy.
2. Reduced Turnaround Time
With Labellerr's efficient annotation tools and automated workflows, the turnaround time for labeling driver monitoring data is dramatically reduced.
This accelerated process enables timely analysis of driver behavior, facilitating proactive interventions to prevent accidents and enhance road safety.
3. Quality Control
Labellerr ensures the accuracy and reliability of labeled data in driver monitoring applications through rigorous quality control measures.
By validating annotations against predefined criteria and standards, Labellerr enhances the trustworthiness and effectiveness of driver monitoring systems, thereby improving overall road safety outcomes.
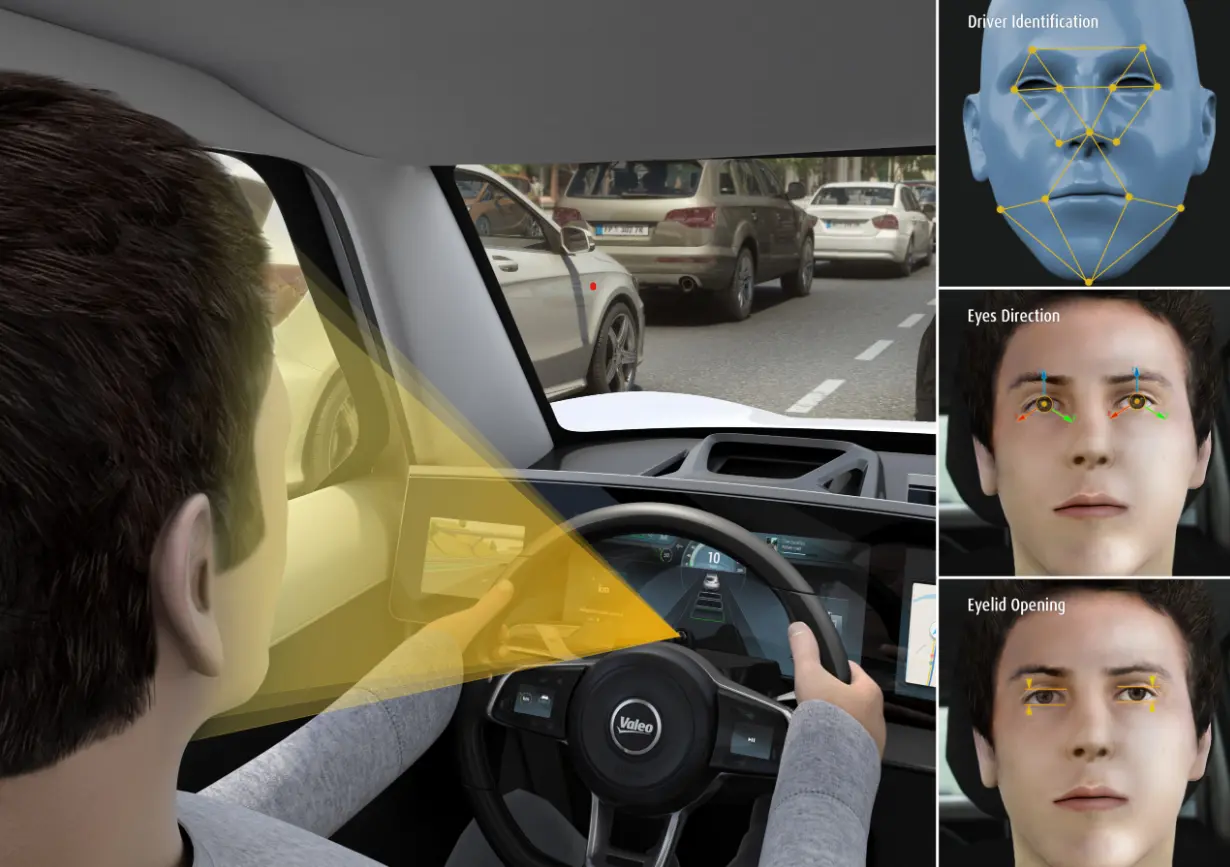
4. Scale Up and Volume
Labellerr's scalability allows for seamless handling of large volumes of driver monitoring data, enabling companies and researchers to scale up their projects as needed.
Whether dealing with extensive datasets or increasing workload demands, Labellerr can efficiently accommodate the scalability requirements of driver monitoring initiatives.
5. Custom Workflows
Labellerr offers customizable workflows tailored to the specific requirements of driver monitoring projects.
This flexibility allows stakeholders to design annotation processes that align precisely with the objectives and parameters of their driver behavior analysis, resulting in more accurate and tailored solutions.
Conclusion
Incorporating Labellerr into driver monitoring systems revolutionizes road safety efforts, providing valuable insights into driver behavior and attentiveness.
By leveraging advanced annotation technologies, companies and researchers empower transportation authorities to proactively address risks and enhance road safety measures.
With Labellerr's support, innovators continue to advance the field of driver monitoring, driving positive change in the transportation industry.
Frequently Asked Questions
1. How can Driver Monitoring improve driving safety?
Driver Monitoring Systems (DMS) utilize machine learning algorithms to analyze various facial and behavioral cues of drivers in real-time, such as eye gaze direction, head position, blink rate, and facial expressions, to assess their cognitive and emotional states.
By continuously monitoring these metrics, DMS can detect driver drowsiness, distraction, or impairment, and issue timely alerts or interventions to mitigate potential risks.
Additionally, DMS can personalize driving assistance features based on individual driver behavior, enhancing overall driving safety by promoting attentiveness and reducing the likelihood of accidents caused by human error.
2. Which technology is best for Driver Monitoring Systems (DMS)?
Computer vision technology is often considered the best fit for Driver Monitoring Systems (DMS).
By leveraging deep learning algorithms trained on large datasets of facial and behavioral data, computer vision systems can accurately detect and analyze various driver cues in real time, such as eye movements, head position, and facial expressions.
This allows for robust detection of driver drowsiness, distraction, or impairment, enabling timely interventions to enhance driving safety.
Additionally, computer vision-based DMS can be seamlessly integrated into existing automotive systems, making it a practical and effective choice for deployment in vehicles.

Simplify Your Data Annotation Workflow With Proven Strategies
.png)
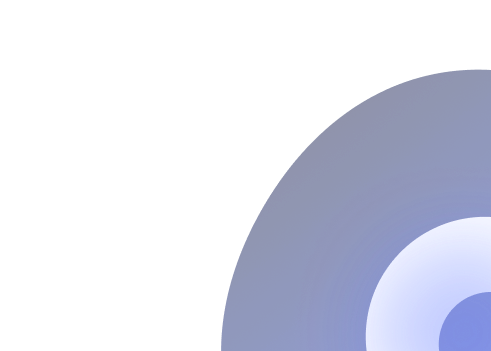