6 Best Scale AI Alternatives in 2025
Explore six top alternatives to Scale AI for data labeling in 2025. From AI-driven platforms like Labellerr and V7 to open-source options like CVAT, discover the best tools tailored for various industries, offering flexibility, cost-efficiency, and scalability.
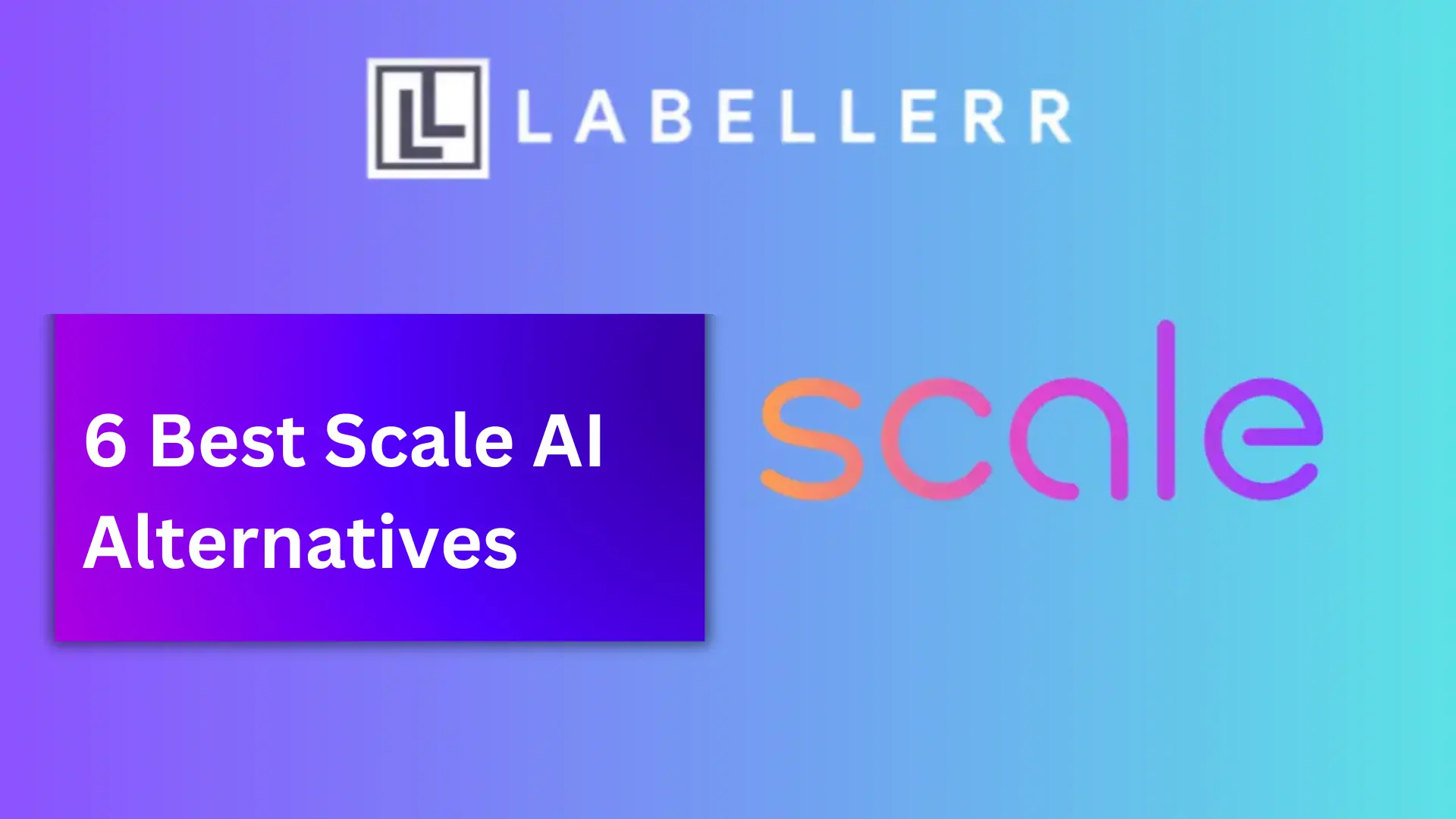
As artificial intelligence advances, it's crucial to focus on data labeling to train machine learning models effectively and improve their performance.
Launched in 2016, Scale AI is one of the most sought-after tools that offers high-quality training data for AI applications such as AR/VR, robotics, mapping, self-driving cars, and more.
Despite its variety of cutting-edge features, Scale AI may not be an ideal fit for every enterprise’s labeled data needs.
Businesses have diverse needs—some prioritize cost-efficiency, others seek customized data solutions, while some prefer platforms that offer greater flexibility or a more hands-on approach.
Fortunately, there are plenty of other better data labeling and annotation tools available on the market.
You can compare and choose one that best fits your specific needs, budget, and goals.
Based on reviews, ratings from various websites, and our team’s personal experience with the software, our data labeling experts have curated a list of the best Scale AI alternatives.
We’ll delve into their unique features, pros, cons, and provide G2 user reviews to help you choose the best for your requirements.
Table of Contents
- What is Scale AI?
- Why consider Scale AI alternatives?
- Top 6 Scale AI Alternatives in 2025 and Beyond
- Labellerr
- V7
- SuperAnnotate
- Dataloop
- Labelbox
- CVAT
- Conclusion
- Frequently Asked Questions
What is Scale AI?
Scale AI is a leading platform that provides high-quality data and AI solutions to power advanced machine learning models.
It helps enterprises, government agencies, and generative AI companies by integrating and managing data efficiently, using AI-driven data labeling and fine-tuning technologies.
Scale AI supports building, optimizing, and deploying AI models tailored to specific business needs.
Why consider Scale AI alternatives?
Although Scale AI is among the first on-demand platforms to deliver data augmentation service and has been one of the most popular choices for a long time, considering scale AI competitors can be a smart decision for a few reasons.
- More budget-friendly options
- New players that provide fresh perspectives
- Integration capabilities
- Customer support
- Customized options
- User experience
Besides, based on reviews on different websites, Scale AI has some downsides, too, that may prompt you to consider its competitors.
- Cost Efficiency: Scale AI's solutions can be expensive, with costs starting at $5,000 for basic models and reaching over $500,000 for advanced applications.
Many alternatives offer similar services at a more budget-friendly rate. - Fresh Perspectives and Features: Newer players in the market often provide innovative solutions, improved integration capabilities, and a better user experience that might suit your specific needs more closely than Scale AI.
- Customization and Flexibility: Alternatives may offer more customized options and better customer support tailored to specific industries, like medical imaging or NLP, where Scale AI's capabilities might be limited.
- Dependency and Control: Relying on an external service like Scale AI creates dependency, which can lead to issues with project timelines if there are service changes or downtimes.
- Privacy and Security: Sharing sensitive data with third-party services can raise privacy and security concerns, especially if your business deals with regulated or confidential data.
Ultimately, exploring the best Scale AI alternatives keeps you informed and ensures you’re maximizing your resources.
We recommend testing out different data labeling software to find the one that best suits your needs.
Top 6 Scale AI Alternatives in 2025 and Beyond
1. Labellerr
Labellerr is a modern data labeling platform that leverages AI-driven automation to improve the accuracy and speed of data annotation.
Its machine learning-assisted labeling, pre-annotation, and quality control features make it a reliable solution for industries such as healthcare, retail, automotive, and more.
Top Features
• AI-driven automation tools for faster labeling
• Pre-labeling using machine learning models
• Supports video, image, and text annotation
• Detailed quality control and reporting
• Seamless integration with ML pipelines
Pros
• Offers fast and efficient workflows
• Easy to use with high-quality output
• Supports a wide variety of data types
• Highly scalable for enterprise needs
Cons
• Limited public recognition compared to some competitors
• Relatively new in the market
Best For: Enterprises looking for a scalable and AI-enhanced data annotation platform.
Pricing: Custom pricing based on enterprise needs.
G2 Review: You can check the customer reviews here.
2. V7
V7 offers an advanced AI-powered data annotation platform designed for handling complex image and video datasets.
It stands out for its ability to automate repetitive tasks through machine learning models, which increases the speed and accuracy of annotations.
V7 is widely used in healthcare, automotive, and retail industries for projects requiring high precision.
It is particularly praised for its user-friendly interface, which allows users to train AI models within the platform and manage annotations with ease.
Top Features:
• AI-assisted auto-labeling for images and videos
• Real-time collaboration tools for teams
• Supports 2D and 3D data annotations
• Model training and iteration within the platform
• Automated quality control tools
Pros:
• AI-powered automation significantly reduces manual work
• User-friendly interface with real-time collaboration features
• Supports a variety of data types, including 3D
• Built-in model training enhances annotation accuracy
Cons:
• Pricing can be prohibitive for smaller projects or teams
• May have a learning curve for complex tools and features
Best For: Teams and enterprises dealing with complex datasets that require automated labeling and integrated AI model training.
Pricing: V7's pricing starts at $150 per month for an unlimited number of users.
G2 Review: You can check the customer reviews here.
3. SuperAnnotate
SuperAnnotate is a powerful annotation tool that combines AI-assisted labeling with manual annotation. It is particularly suited for image, video, and text annotations, offering both 2D and 3D support.
SuperAnnotate’s collaboration features make it a great choice for teams needing to manage large-scale projects efficiently.
Top Features:
• AI-powered auto-annotation for 2D and 3D data
• Collaboration tools for teams
• Built-in quality control workflows
• Supports a wide range of data types
• Customizable workflows for different industries
Pros:
• High accuracy with AI and manual annotation
• Strong team collaboration features
• Easy to use with real-time feedback
Cons:
• Can be expensive for smaller teams
• Some advanced features have a steep learning curve
Best For: Teams needing precise annotation for complex visual data with a blend of AI and human input.
Pricing: In terms of pricing, SuperAnnotate is affordable, starting at $62 per month per user.
They also provide a free 14-day trial for users to explore the platform.
G2 Review: You can read the customer reviews here.
4. Dataloop
Dataloop provides a fully integrated data management and annotation platform with AI-assisted labeling tools.
The platform is designed for handling large-scale, complex datasets and includes collaboration features to streamline project workflows.
Top Features:
• AI-driven auto-annotation
• Workflow automation and collaboration tools
• Supports image, video, and text data
• End-to-end data management solutions
• Human-in-the-loop quality control
Pros:
• Ideal for complex, large-scale projects
• Strong data management and collaboration features
• AI-powered labeling increases efficiency
Cons:
• Can be overwhelming for smaller teams
• Higher learning curve for new users
Best For: Enterprises needing an end-to-end solution for data annotation and management with AI integration.
Pricing: Custom pricing based on usage and project size.
G2 Review: You can check the customer reviews here.
5. Labelbox
Labelbox is a platform for labeling data, with a focus on collaboration and automation.
Labelbox is a data labeling platform built for enterprise AI projects. It provides an intuitive interface and workflow management tools for creating and managing labeled datasets.
With AI-assisted labeling and customizable templates, Labelbox helps teams reduce manual labor while ensuring high-quality annotations.
Top Features:
• AI-powered labeling tools
• Customizable templates for data annotation
• Collaboration and workflow management
• Integration with major ML pipelines
• Quality assurance with human-in-the-loop processes
Pros:
• Easy-to-use interface with customizable workflows
• Strong integration with ML frameworks
• Scalable for large datasets
Cons:
• Pricing may be high for smaller teams
• Limited support for 3D annotation
Best For: Large AI teams requiring scalable annotation solutions with customizable workflows and collaboration tools.
Pricing: Tiered pricing based on project needs.
G2 Review: You can check the reviews here.
6. CVAT
If you're watching your budget, the Computer Vision Annotation Tool (CVAT) might be the way to go.
CVAT is an open-source tool designed for annotating images and videos. It is especially popular for computer vision tasks such as object detection, image classification, and segmentation.
CVAT supports various data formats and provides an efficient annotation interface that allows users to create, edit, and manage projects.
Top Features:
• Open-source and customizable
• Supports image and video annotations
• Pre-built tools for object detection and segmentation
• Multi-user collaboration
• Integration with machine learning pipelines
Pros:
• Free and open-source
• Highly customizable for advanced users
• Supports multiple annotation types
Cons:
• Steep learning curve for non-technical users
• Limited customer support for troubleshooting
Best For: Technical teams and researchers needing a cost-effective, customizable solution for image and video annotation.
Pricing: Free and open-source.
G2 Review: You can check the reviews here.
Conclusion
As artificial intelligence continues to advance, the importance of accurate and relevant labeled data becomes crucial for training machine learning models effectively.
Scale AI stands out for its data labeling services, diverse annotation types, scalability, and quality control measures.
However, considering factors like cost, dependency on external services, and privacy concerns, exploring alternatives like Labellerr, V7, SuperAnnotate, Dataloop, Labelbox, and CVAT becomes essential.
Remember to consider factors like budget, innovation, integration capabilities, and user experience when making your decision.
By investing time in exploring these alternatives, you can find the perfect fit to to prepare training data from unstructured data without being constrained to conventional restrictions.
Frequently Asked Questions
1. What is scale AI?
Scale AI, headquartered in San Francisco, is an artificial intelligence company that assists businesses in training and labeling their AI software.
In August, OpenAI collaborated with Scale AI to refine turbo versions of its GPT large language models.
Scale AI has also established partnerships with various companies, including Meta, Microsoft, Toyota, and General Motors.
2. What are the alternatives to scale AI?
Several alternatives to Scale AI exist in the AI data labeling and training domain.
Companies like Labelbox, Supervisely, and Alegion offer similar services, providing platforms for data annotation, model training, and deployment.
Additionally, Amazon SageMaker Ground Truth, Google Cloud AutoML, and Microsoft Azure Custom Vision are cloud-based solutions that include data labeling capabilities, catering to businesses seeking comprehensive AI development tools.
The choice among these alternatives often depends on specific project requirements, budget constraints, and integration preferences.
3. What is data labeling?
Data labeling is the process of annotating or tagging data to make it understandable for machine learning algorithms.
It involves assigning specific labels or tags to different elements within a dataset, such as images, text, or audio, to provide the algorithm with labeled examples for learning patterns and making predictions.
This annotated data is crucial for training machine learning models, as it enables the algorithms to generalize and make accurate predictions when faced with new, unseen data.
Data labeling is a fundamental step in the supervised learning pipeline, where the algorithm learns from labeled examples to make predictions on new, unlabeled data.

Simplify Your Data Annotation Workflow With Proven Strategies
.png)
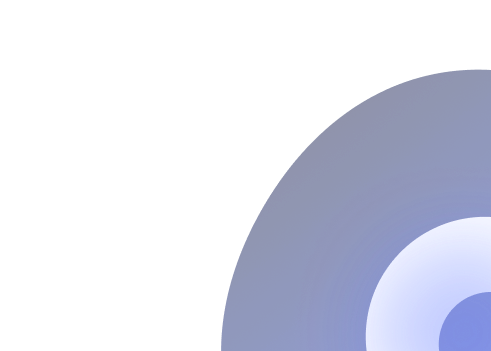