19 Smart Automobile Use Cases Using Vision AI
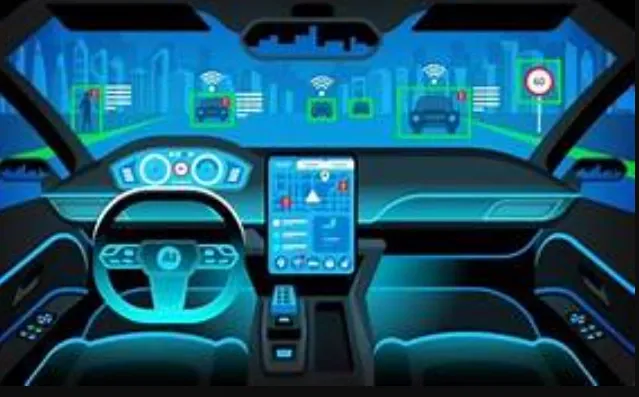
Artificial intelligence and data annotation are driving transformative innovations in the automobile industry. Their integration has the potential to revolutionize vehicle design, manufacturing, on-road safety, and autonomous driving. This blog explores the vital roles of AI and data annotation in the automobile sector, showcasing diverse use cases and annotation methods shaping the future of smart automobiles.
Crucial Roles of AI and Data Annotation in Robotics
- Traffic Detection and Traffic Signs
- AI Application: AI-powered computer vision systems are used to detect and recognize traffic signs and signals.
- Data Annotation: Data annotation is essential to train AI models to recognize and interpret traffic signs accurately. This includes annotating images or video frames with labels indicating the presence and type of traffic signs.
- Benefits: Improved road safety, reduced human error, and faster response to accidents through AI-enabled traffic detection and sign recognition.
2. Lane & Road Marking
- AI Application: AI-based computer vision is employed to detect and interpret lane markings, road edges, and other road markings.
- Data Annotation: Data annotation involves marking lanes, arrows, stop signs, and other relevant road features in images or video frames.
- Benefits: Enhanced driving assistance systems that help human drivers stay in their lanes, recognize road markings, and drive safely.
3. Traffic Flow Analysis
- AI Application: AI is used for real-time traffic flow analysis, which includes pathway analysis, people counting, and dwell time analysis.
- Data Annotation: Annotated data is essential to train AI systems to accurately count and analyze vehicle, bus, and train movements in traffic.
- Benefits: Efficient traffic management, improved road safety, and better urban planning through AI-driven traffic flow analysis.
4. AI-Powered Parking Management
- AI Application: AI and computer vision are integrated into smart parking management systems to count parked vehicles and monitor parking space availability.
- Data Annotation: Annotated data is used to train AI systems to recognize and track parked vehicles.
- Benefits: Improved parking space utilization, reduced congestion, and enhanced user experience in parking lots.
5. Road Condition Monitoring (RCM)
- AI Application: AI-based in-vehicle optical road monitoring systems are used to monitor and classify road conditions in real time.
- Data Annotation: Data annotation involves labeling images or video frames with information about road conditions, such as potholes, debris, or snow.
- Benefits: Enhanced road maintenance, improved road safety, and reduced vehicle wear and tear through AI-powered road condition monitoring.
6. Automatic Traffic Incident Detection
- AI Application: AI, coupled with video surveillance, is used to monitor road networks and detect traffic incidents automatically.
- Data Annotation: Annotated and labeled data is crucial to train AI systems to recognize and respond to various traffic incidents.
- Benefits: Faster and more accurate detection of accidents and incidents, leading to improved road safety.
7. Automated License Plate Recognition (ANPR)
- AI Application: ANPR systems automatically recognize and read license plate numbers for various applications.
- Data Annotation: Data annotation is essential for training AI models to identify and interpret license plate characters accurately.
- Benefits: Enhanced security and convenience, as ANPR is used for tasks like parking management and law enforcement.
8. In-Cabin/Driver or Occupant Monitoring
- AI Application: AI is used for monitoring drivers and occupants in vehicles, ensuring safety and attention.
- Data Annotation: Data annotation is crucial for training AI systems to recognize and interpret driver and occupant behavior, such as drowsiness or distractions.
- Benefits: Enhanced safety and assistance for drivers and passengers in autonomous and non-autonomous vehicles.
9. Driver Assistance
- AI Application: AI-based driver assistance systems use sensors and cameras to assist drivers in real-time by monitoring blind spots, pedestrian detection, and providing warnings or intervention when necessary.
- Data Annotation: Data annotation is crucial for training AI models to recognize and respond to various objects and situations on the road, such as pedestrians, other vehicles, and obstacles.
- Benefits: Enhanced safety, reduced accidents, and improved driver awareness through AI-powered driver assistance systems.
10. Autonomous Vehicles
- AI Application: AI, including machine learning, deep learning, and sensor fusion, is the foundation for autonomous vehicles, allowing them to navigate, detect obstacles, and make driving decisions without human intervention.
- Data Annotation: Annotated data from various sensors (lidar, radar, cameras) is used to train AI algorithms to perceive and understand the surrounding environment.
- Benefits: Potential for safer and more efficient self-driving cars, leading to reduced accidents and enhanced mobility.
11. Driver Monitoring
- AI Application: AI algorithms monitor driver behavior, including detecting drowsiness and ensuring user recognition. This technology can adjust settings like mirrors and seat positions.
- Data Annotation: Annotated data of driver behavior and facial features is used to train AI systems for monitoring and recognition.
- Benefits: Improved safety, reduced accidents due to driver fatigue, and enhanced driver comfort.
12. AI in Manufacturing
- AI Application: AI technologies, including robotics and machine learning, are employed in manufacturing to optimize production processes, improve quality control, and enhance efficiency.
- Data Annotation: Annotated data can be used to train robots and AI systems to handle tasks such as parts assembly and quality control.
- Benefits: Faster and more efficient manufacturing processes, improved quality, and reduced errors.
13. Personal Assistant
- AI Application: AI-enabled personal assistants integrated into vehicles can understand and respond to voice commands, offering features such as making calls, adjusting settings, and providing information.
- Data Annotation: Annotated data helps train the voice recognition and natural language processing (NLP) systems used in personal assistants.
- Benefits: Enhanced in-car user experience, hands-free operation, and improved convenience.
14. Passenger Experience
- AI Application: AI technologies, including IoT, image data analysis, and object recognition, are used to enhance passenger experiences in vehicles.
- Data Annotation: Annotated data can help train AI systems to recognize objects, provide entertainment options, and offer personalized services to passengers.
- Benefits: Improved passenger comfort, engagement, and entertainment during travel.
15. Supply Chain Management
- AI Application: AI is utilized to optimize supply chain management, ensuring efficient tracking and logistics of automotive components.
- Data Annotation: Annotated data may be used to monitor the transportation journey of vehicle parts, track arrivals, and predict demand spikes.
- Benefits: Improved supply chain efficiency, reduced delays, and enhanced production planning.
16. Automotive Insurance
- AI Application: AI is used in the insurance sector to expedite the claims process, detect incidents, and assess vehicle damage.
- Data Annotation: Annotated data assists in image analysis and damage assessment, facilitating faster and more accurate claims processing.
- Benefits: Speedier claims processing, reduced fraud, and improved customer service in the insurance industry.
17. Quality Control
- AI Application: AI-based quality control systems are used to inspect vehicles for defects and identify issues during manufacturing.
- Data Annotation: Annotated data is essential to train AI systems to recognize and categorize defects and quality issues.
- Benefits: Improved vehicle quality, reduced defects, and faster issue resolution.
18. Connected Cars
- AI Application: IoT and AI technologies enable vehicles to communicate with each other and infrastructure, enhancing safety and traffic management.
- Data Annotation: Annotated data may involve mapping roads, monitoring traffic, and facilitating vehicle-to-vehicle communication.
- Benefits: Safer and more efficient traffic flow, reduced accidents, and better road management.
19. AI in Designing
- AI Application: AI streamlines the vehicle design process by assisting in tasks such as real-time tracking, programmable shading, and generating design ideas.
- Data Annotation: Annotated data can be used to train AI systems for design and engineering tasks.
- Benefits: Faster and more efficient design processes, better design workflows, and product innovation.
These use cases illustrate the wide-ranging applications of AI, computer vision, and data annotation in the automotive industry, contributing to safety, efficiency, and innovation across various aspects of vehicle design, manufacturing, operation, and management.
Data Annotation Types Used for Smart Automobiles
- Bounding Boxes
- Description: Bounding boxes are rectangular or square shapes drawn around objects in an image or frame of a video. They indicate the location and size of the object of interest.
- Use Cases: Bounding boxes are commonly used for object detection and localization. They are especially useful when the shape of objects is relatively simple and can be approximated by a rectangle.
- Examples: Identifying and localizing cars, pedestrians, traffic signs, and other objects on the road.
2. 3D Cuboids
- Description: 3D cuboids are similar to bounding boxes but provide three-dimensional information. They are used to annotate objects in terms of depth, width, and length, covering the X, Y, and Z axes.
- Use Cases: 3D cuboids are essential for understanding the 3D position and volume of objects in space. This is critical for depth perception and collision avoidance in autonomous vehicles.
- Examples: Annotating the 3D position and size of vehicles, pedestrians, and obstacles.
3. Polygons
- Description: Polygons are irregular shapes drawn around objects in images or videos. They provide more accurate object detection and location for objects with complex or irregular shapes.
- Use Cases: Polygons are used when objects such as humans, animals, or bikes cannot be effectively enclosed by a 2D or 3D bounding box. They offer a more precise representation of object boundaries.
- Examples: Outlining and accurately locating pedestrians, animals, or objects with non-rectangular shapes.
4. Semantic Segmentation
- Description: Semantic segmentation involves associating every pixel in an image with a class label. It divides an image into segments or regions, each corresponding to a specific object or class.
- Use Cases: Semantic segmentation is crucial for understanding the environment in fine detail. It allows a self-driving car to differentiate between various objects and their surroundings.
- Examples: Identifying and segmenting individual objects like cars, pedestrians, traffic lights, and road surfaces within an image.
5. Lines and Splines
- Description: Annotators draw lines or splines in images to indicate boundaries, lanes, and edges. These lines help train models to recognize and navigate road lanes.
- Use Cases: Lines and splines are important for autonomous vehicles to follow lane discipline, navigate safely in traffic, and avoid collisions.
- Examples: Annotating lane markings, road edges, and other critical lines for autonomous driving.
These annotation methods are chosen based on the specific requirements of the project and the complexity of the objects and information that need to be annotated. Each method plays a critical role in training machine learning models for autonomous and semi-autonomous vehicles, enabling them to perceive and navigate the real world accurately and safely.
Benefits of AI in the Automobile Industry
AI has brought about numerous advantages in the automotive sector, revolutionizing how vehicles are conceived, crafted, and operated. The following are key benefits of AI in the automotive industry:
Enhanced Safety: AI has introduced advanced safety systems, such as lane departure warning (LDW), autonomous emergency braking (AEB), and adaptive cruise control (ACC). These systems can detect potential hazards, alert drivers, take preventive actions, and mitigate the risk of accidents.
Predictive Maintenance: AI, in conjunction with IoT, offers predictive maintenance capabilities. IoT systems monitor real-time vehicle conditions by analyzing extensive data, enabling proactive maintenance to address issues before they become critical. This helps reduce downtime and improves vehicle performance.
Improved Driver Experience: Smart vehicles equipped with AI-driven infotainment systems provide personalized experiences to passengers and drivers. Voice assistants understand regional languages and perform tasks like music playback, navigation, and climate control, enhancing the overall driving experience.
Autonomous Driving: AI technology has enabled the development of self-driving cars, offering increased safety, mobility, and traffic flow optimization. This innovation has particularly benefited individuals with mobility challenges, allowing them to access autonomous transportation.
Cost Savings: AI implementation in the automotive industry optimizes various operations, ranging from design to manufacturing. It enhances manufacturing processes, streamlines supply chains, and identifies potential vehicle issues early on, resulting in significant cost reductions.
AI has made substantial contributions to the automotive industry by transforming safety, maintenance, driver experience, autonomy, and operational cost efficiency. These advancements are reshaping how vehicles are designed, manufactured, and utilized.
LabelGPT for Faster Data Annotation in the Automobile Industry
In the ever-evolving landscape of AI applications in the automobile industry, we are excited to introduce LabelGPT, a pioneering prompt-based labeling tool that has the potential to revolutionize data annotation for automotive-related use cases.
While LabelGPT was originally designed for broader applications, its capabilities can be harnessed effectively in the realm of automotive data annotation. Let's explore how LabelGPT can be utilized in this context:
Import Automotive Data: Seamlessly connect your automotive-related images and videos from diverse sources, including vehicle camera feeds, road surveillance footage, and sensor data, to the LabelGPT platform.
Specify Labeling Requirements: Provide text prompts that precisely define the objects and elements within the automotive data that require annotation. You can select from various labeling types, such as vehicle detection and tracking, lane marking recognition, or road sign classification.
Effortless Review: Within a short span of time, LabelGPT will generate annotated automotive content and furnish confidence scores. These scores serve to validate the accuracy and reliability of the annotations. Reviewing and validating the labels is made simple.
Benefits of LabelGPT in the Automobile Industry
Eliminate Manual Labeling: LabelGPT's labeling engine, driven by advanced AI models, can automatically detect and label automotive-related objects and events based on straightforward prompts. This eliminates the need for labor-intensive manual annotation, which is especially time-consuming in the automotive domain.
Rapid Labeling: Bid farewell to the arduous task of labeling automotive data frame by frame. LabelGPT can annotate multiple elements within automotive footage with a single prompt, significantly reducing the time and effort required for data annotation.
Enhanced Accuracy: LabelGPT provides confidence scores, guaranteeing that the annotations adhere to rigorous quality standards. This precision is paramount for the success of machine learning models in the automotive industry, where safety and reliability are of utmost importance.
In the automotive industry, LabelGPT stands as a transformative tool that streamlines data annotation, accelerates the development of autonomous driving algorithms, and ensures the highest levels of accuracy and safety in AI-driven automotive applications.
Conclusion
As the automotive industry accelerates towards a future defined by autonomous driving, connectivity, and enhanced safety, AI and data annotation stand as indispensable pillars of progress.
From improving road safety through traffic sign recognition to streamlining manufacturing processes and advancing autonomous vehicles, the applications are both diverse and promising.
The precise annotation methods, including bounding boxes, polygons, semantic segmentation, and more, enable machines to perceive and navigate the world with ever-increasing accuracy.
In this evolving automotive landscape, the role of AI and data annotation cannot be overstated. They are the driving forces behind safer roads, more efficient transportation, and vehicles that are not merely smart, but intelligent.
The collaboration of these technologies continues to reshape the automotive industry, ushering in an era where the roads are safer, the driving experience is enhanced, and the future is undeniably smarter.
Frequently Asked Questions
1. What are the most common AI use cases in the automotive industry?
Driver assistance systems represent one of the most remarkable and impactful applications of artificial intelligence in the automotive industry. These systems leverage AI's capabilities, including computer vision and sensor fusion, to enhance road safety and improve the driving experience.
By continuously monitoring the vehicle's surroundings, AI-driven sensors and cameras can detect and respond to potential hazards in real-time. Whether it's identifying pedestrians, other vehicles, or obstacles, these systems provide crucial warnings or even intervene by autonomously applying brakes or steering to prevent accidents.
Additionally, AI's role in driver assistance extends to monitoring driver behavior, detecting signs of fatigue or distraction, and ensuring driver attentiveness through timely alerts. The result is a substantial reduction in accidents caused by human errors, making roads safer and driving more secure and convenient.
2. How artificial intelligence is transforming the automobile industry?
Artificial Intelligence (AI) is driving a swift and profound transformation in the automobile industry, establishing itself as a vital element of autonomous driving technology.
The pivotal catalyst behind the triumph of AI applications across industries, including automotive, is the vast reservoir of data amassed through interconnected devices and services.
3. Will AI reshape the automotive industry by 2025?
According to a Deloitte report, the automotive industry is poised to reach a staggering total worth of USD 27 billion by the year 2025. This report underscores the significant potential of artificial intelligence (AI) and machine learning (ML) in revolutionizing the automotive landscape and our perception of cars.
4. What are the advantages of AI in the automotive industry?
AI's integration into the automotive industry has brought about a profound transformation, notably in enhancing road safety. This improvement encompasses several key aspects:
- Advanced Driver Assistance Systems (ADAS): AI powers ADAS, which uses sensors and cameras to monitor the vehicle's surroundings in real time. It can detect potential hazards, such as pedestrians, vehicles, and obstacles, and take preventive actions, like issuing warnings or applying brakes to avert collisions.
- Driver Behavior Monitoring: AI algorithms analyze driver behavior for signs of fatigue or distraction and issue alerts to ensure driver attentiveness, reducing accidents caused by human errors.
- Autonomous Vehicles: AI is fundamental to self-driving cars, which can navigate and make driving decisions autonomously. By eliminating human errors, autonomous vehicles have the potential to significantly enhance road safety.
- Traffic Management: AI-driven traffic flow analysis optimizes traffic signals, reduces congestion, and aids in urban planning, resulting in smoother traffic and fewer accidents due to gridlocks and chaotic road conditions.
Book our demo with one of our product specialist
Book a Demo