10 Best Video Annotation & Labeling Service Providers In 2024
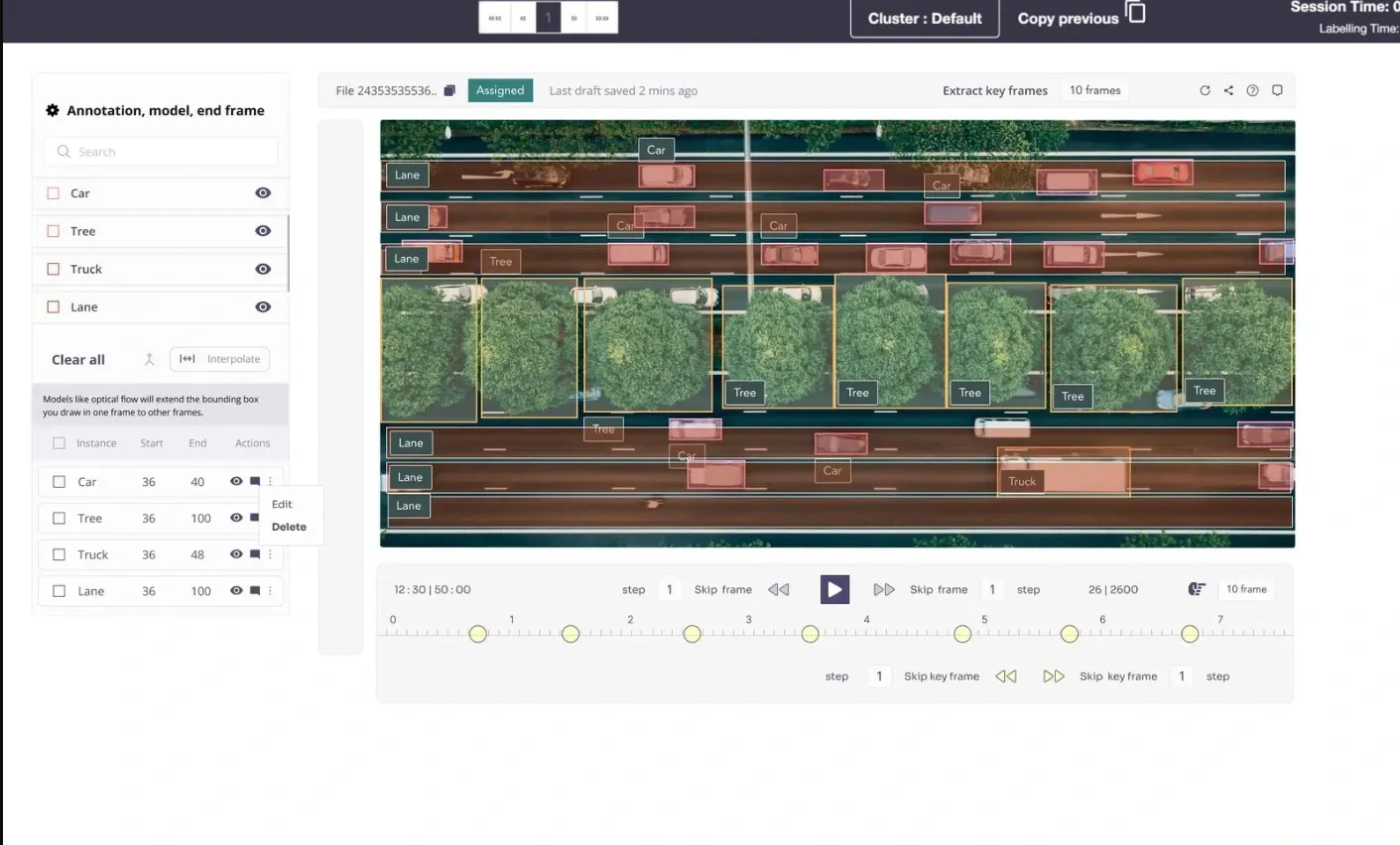
Video Annotation has become an essential technique in computer vision and machine learning fields.
It is essential to improving accuracy since it gives algorithms contextual data and helps identify certain items in video frames.
A number of platforms with distinct features and capabilities have arisen in response to the growing demand for efficient video annotation solutions.
We'll examine the best 10 Video Annotation Services in this blog, emphasising their unique features, usability, and inventiveness.
Table of Contents
- Labellerr
- Labelbox
- Supervisely
- VGG Image Annotator (VIA)
- Scale AI
- Amazon SageMaker Ground Truth
- Hive
- Dataturks
- iMerit
- Appen
- Conclusion
- Frequently Asked Questions
Here's the List:
1. Labellerr
Labellerr stands out as a cloud-based video annotation service designed to simplify the annotation process while supporting various video formats. The platform offers an intuitive interface that facilitates ease of use, making it accessible for users with varying levels of expertise. What sets Labellerr apart is its integration of automated data labeling features, a functionality that significantly expedites the model training process. This automation capability allows users to streamline image annotation projects with just a few clicks, leading to time savings and cost reduction.
The platform provides a range of annotation tools, including bounding boxes, polygons, key points, semantic segmentation, and more. This versatility empowers users to annotate videos with high accuracy and speed. Labellerr's support for real-time collaboration further enhances its usability, enabling team members to work together seamlessly on annotation projects.
Labellerr caters to the growing demand for advanced video annotation capabilities, offering features for object tracking, segmentation, and detection. The platform emphasizes its ability to label video datasets at a faster rate, claiming to be ten times more efficient. This efficiency is achieved through an advanced and feature-rich video labeling platform that supports a variety of annotation types.\
The video annotation types supported by Labellerr include semantic segmentation, instance segmentation, bounding box annotation, polygon annotation, skeletal annotation, key points annotation, bitmask annotation, custom annotation, and lane annotation. These annotation types cover a broad spectrum of use cases, allowing users to choose the most suitable method for their specific requirements.
Labellerr places a strong emphasis on the quality control aspects of annotation. It acknowledges the challenges associated with video annotation, where each frame requires meticulous labeling. To address this, the platform may incorporate automated quality assurance mechanisms. It encourages consensus between annotators to enhance accuracy, includes model-assisted quality assurance, and provides analytics tools to track progress, time spent per file, and annotation completion in real-time.
The platform also facilitates the import and export of data, allowing users to create batch runs for data processing in the cloud. This feature contributes to the overall efficiency of the annotation workflow.
Labellerr positions itself as an advanced video annotation service, offering a user-friendly interface, automation capabilities, and a variety of annotation types to cater to diverse machine learning and computer vision projects. Its focus on real-time collaboration, quality control, and analytics further reinforces its commitment to providing a comprehensive solution for video annotation needs.
2. Labelbox
Labelbox is a comprehensive data labeling platform that stands out for its customizable workflows, allowing users to design and tailor annotation processes to meet specific project requirements. This flexibility is particularly valuable for diverse annotation tasks. The platform supports collaborative efforts with features enabling multiple users to work simultaneously on the same project, fostering teamwork and efficiency. Additionally, Labelbox incorporates automation tools, including machine learning-assisted annotation, to accelerate the labeling process.
Labelbox caters to a broad spectrum of use cases, such as object detection, image segmentation, and video analysis. It finds applications in industries like autonomous vehicles, healthcare, and robotics, where accurate and efficient data annotation is crucial. In terms of integration, Labelbox seamlessly integrates with popular machine learning frameworks like TensorFlow and PyTorch, ensuring compatibility with existing ML workflows.
3. Supervisely
Supervisely distinguishes itself as an end-to-end platform covering data annotation, model training, and deployment, aiming to streamline the entire machine learning workflow. The platform supports video annotation, allowing users to label objects, actions, or events in video sequences. Supervisely further provides pre-trained models, enabling users to kickstart their projects with existing deep learning models.
Suitable for various computer vision tasks, including object detection, image segmentation, and video analysis, Supervisely caters to industries such as agriculture, retail, and manufacturing. Its integration capabilities include seamless connections with popular deep learning frameworks like TensorFlow and PyTorch, offering APIs for effortless integration into custom workflows.
4. VGG Image Annotator (VIA)
VGG Image Annotator (VIA) is an open-source project developed by the Visual Geometry Group at the University of Oxford. Its open-source nature allows users the flexibility to customize and extend the tool based on their specific needs. VIA supports custom attributes for annotations, providing a high level of flexibility for video and image annotation tasks.
Primarily suitable for research projects and scenarios where customization and extensibility are critical, VIA is commonly used in academic and research settings. As an open-source tool, VIA can be integrated into custom workflows and research pipelines, allowing users to contribute to the tool's development and improvement.
5. Scale AI
Scale AI offers a managed platform for data labeling, emphasizing high-quality annotations through a combination of human-in-the-loop and automation processes. The platform provides flexibility to handle various annotation tasks, including video annotation, catering to machine learning projects in need of high-quality labeled data.
Scale AI serves industries such as autonomous vehicles, e-commerce, and robotics, where precision in data labeling is crucial. Its integration capabilities extend to popular machine learning frameworks and platforms, with an API that facilitates seamless integration into custom workflows, ensuring adaptability to existing machine learning pipelines.
6. Amazon SageMaker Ground Truth
Amazon SageMaker Ground Truth is a fully managed data labeling service provided by AWS. As a fully managed platform, it handles the end-to-end process of data labeling, reducing the operational burden on users. The platform incorporates active learning, an iterative process involving model-driven sample selection for annotation, contributing to the reduction of annotation costs.
Suitable for a wide range of machine learning applications, including computer vision, natural language processing, and speech recognition, SageMaker Ground Truth caters to users across various industries leveraging AWS services. Its seamless integration with Amazon SageMaker and other AWS services provides a cohesive environment for machine learning workflows, benefiting from the scalability and flexibility of the broader AWS ecosystem.
7. Hive
Hive Data distinguishes itself as a platform offering annotation services tailored for both images and videos, encompassing critical tasks such as object detection, image segmentation, and video analysis. This capability makes it a versatile tool for a wide array of industries where annotated data plays a pivotal role in training machine learning models.
One notable feature of Hive Data is its commitment to maintaining annotation accuracy and overall data quality. Quality control mechanisms are likely integrated into the platform, ensuring that the annotations generated meet high standards. This commitment is crucial for the reliability and effectiveness of machine learning models trained on the annotated data.
The platform's use cases span across diverse industries, including but not limited to robotics, agriculture, and manufacturing. In these sectors, where precision and specificity in data annotation are paramount, Hive Data finds applicability as a solution for enhancing the capabilities of machine learning models.
Regarding integration, Hive Data understands the varying needs of different projects and industries. Depending on specific project requirements, the platform may offer options for exporting annotated data or direct integration with popular machine learning frameworks. This flexibility in integration showcases Hive Data's commitment to adaptability, allowing users to seamlessly incorporate annotated data into their existing machine learning workflows. Whether through exports or direct connections, the platform aims to facilitate a smooth integration process, acknowledging the diverse needs of its user base.
8. Dataturks
Dataturks is a versatile data labeling platform that excels in providing annotation services for a diverse range of data types, including images, videos, and text. This breadth of support makes it a comprehensive solution for projects involving different modalities of data. The platform boasts collaboration tools that enhance the efficiency of teams working on annotation projects, facilitating seamless cooperation and coordination among team members.
One of Dataturks' notable strengths lies in its support for various annotation types. Users can leverage the platform for tasks requiring bounding boxes, polygons, and key points, providing flexibility for a wide array of annotation needs. This versatility is particularly advantageous for addressing complex computer vision tasks, including object detection, image segmentation, and video analysis.
The use cases for Dataturks are diverse, with a specific focus on computer vision applications. Its suitability for tasks such as object detection and image segmentation underscores its relevance in training machine learning models for visual data interpretation. The platform caters to industries and projects where accurate and detailed annotation is crucial for achieving optimal model performance.
In terms of integration, Dataturks acknowledges the importance of seamlessly fitting into existing workflows. It may offer export options for annotated data, allowing users to efficiently incorporate the labeled data into their preferred downstream processes. Furthermore, Dataturks is designed to be integrated into custom workflows, providing adaptability to the unique requirements of different projects. This integration capability enhances its usability for users who seek a tailored and cohesive annotation solution.
9. iMerit
iMerit stands out as a prominent data annotation platform, offering a comprehensive suite of services for various data types, including images, videos, and other formats. The platform is distinguished by its commitment to delivering high-quality annotation services, catering to the specific needs of computer vision, natural language processing, and machine learning projects. This emphasis on quality makes iMerit a reliable solution for projects where precise and accurate annotations are paramount.
One of the notable features of iMerit is its versatility in supporting a wide range of annotation tasks. From object detection and image segmentation to video labeling, the platform addresses diverse requirements in the field of data annotation. This flexibility is particularly valuable for users working on complex machine learning projects that involve different types of data and annotation methodologies.
iMerit finds its applicability across various industries, with a focus on sectors where detailed annotation plays a pivotal role. Industries such as healthcare, autonomous vehicles, and retail benefit from iMerit's capability to provide accurate annotations. In healthcare, for instance, precise annotations are crucial for training models in medical imaging. In the autonomous vehicle industry, accurate annotations contribute to the development of robust perception systems, while in retail, detailed annotations aid in tasks like product recognition and inventory management.
Integration is a key consideration for any data annotation platform, and iMerit acknowledges this by potentially offering API access. This API access enhances the platform's compatibility with diverse workflows, enabling seamless integration into existing systems. This adaptability ensures that users can leverage iMerit's annotation services without disrupting their established processes, fostering a smooth and efficient workflow.
10. Appen
Appen distinguishes itself as a leading crowdsourcing platform specializing in data annotation services, catering to a variety of data types, including images, videos, and audio. What sets Appen apart is its utilization of a global crowd of workers, enabling diverse and scalable annotation tasks. This approach is particularly advantageous for projects with large-scale annotation needs, as it taps into a global workforce to handle tasks ranging from image and video annotation to more complex audio annotation.
The platform's support for various annotation types and its implementation of quality assurance measures ensure the delivery of accurate and reliable annotated datasets. This versatility allows Appen to address a wide range of annotation tasks, making it suitable for diverse machine learning applications. The quality assurance measures implemented by Appen contribute to the creation of high-quality datasets, essential for training machine learning models effectively.
Appen finds its suitability in projects that require expansive and diverse annotated datasets for training machine learning models. The platform's ability to handle large-scale annotation tasks makes it a valuable resource for industries such as artificial intelligence, where robust training data is crucial for the development of accurate and effective models.
Furthermore, Appen's commitment to integration is evident in its provision of APIs, facilitating seamless integration into machine learning workflows. This feature enhances the platform's accessibility and usability, allowing users to leverage its crowdsourcing capabilities without disrupting their existing machine learning pipelines. In essence, Appen's crowdsourcing model, global reach, and integration capabilities position it as a versatile solution for projects demanding scalable and diverse data annotation.
Conclusion
With options to suit a wide range of requirements and price points, the video annotation services market is broad and varied. The best service will rely on your unique project requirements and preferences. There are a variety of options available, from customisable choices like VIA and Scale AI to user-friendly, cloud-based platforms like Labellerr and Labelbox to the worldwide reach of crowdsourcing platforms like Appen. When making your decision, take into account aspects like scalability, quality control measures, automation capabilities, supported annotation kinds, convenience of usage, and integration choices. You can choose the video annotation service that gives you the ability to precisely and effectively train your machine learning models by carefully assessing your demands and looking through the available possibilities.
Frequently Asked Questions
1. What is video annotation software?
Video annotation software is a specialized tool used in computer vision and machine learning to label and annotate objects, actions, or events within video frames. It aids in training algorithms by providing contextual data, enhancing accuracy, and facilitating the identification of specific elements in videos.
These tools offer various annotation types such as bounding boxes, polygons, key points, and segmentation, streamlining the annotation process and enabling real-time collaboration for efficient model training. Automated features within these platforms further expedite the annotation workflow, contributing to time savings and cost reduction in machine learning projects.
2. How do video annotation services work?
Video annotation services work by allowing users to label and annotate objects, actions, or events within video frames, providing crucial contextual data for computer vision and machine learning algorithms. Users typically upload video datasets to the annotation platform, where they can employ various annotation tools such as bounding boxes, polygons, key points, or segmentation to mark and identify specific elements in each frame.
Some services integrate automated data labeling features, enhancing efficiency by automating parts of the annotation process. Real-time collaboration features enable multiple users to work simultaneously on annotation projects. Quality control mechanisms, analytics tools, and support for diverse annotation types further contribute to the accuracy and effectiveness of the annotation workflow. The annotated data can then be exported or seamlessly integrated into machine learning pipelines for model training.
3. How do I annotate video data?
To annotate video data, you can use specialized video annotation software or platforms. Begin by uploading your video dataset to the chosen platform, where you'll find annotation tools such as bounding boxes, polygons, key points, or segmentation. Select the appropriate annotation type based on your project requirements and use these tools to mark and label objects, actions, or events within each video frame.
Some platforms may offer automated features to expedite the process. Ensure consistency and accuracy in labeling throughout the video, and leverage collaborative features if multiple annotators are involved. Once annotated, the data can be exported or seamlessly integrated into your machine learning workflow for model training.
Book our demo with one of our product specialist
Book a Demo