Speed Up Medical Imaging Annotation With Labellerr
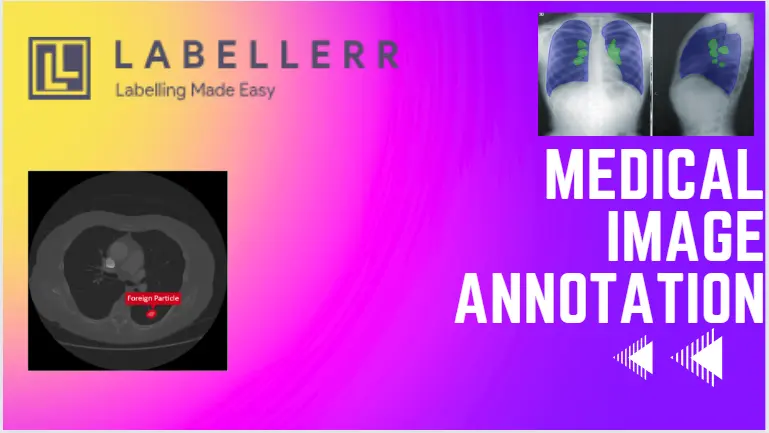
Table Of Content
Introduction
In healthcare industry, the accurate interpretation of medical imaging data, such as CT scans, is important for effective diagnosis and treatment planning.
By using advanced algorithms and machine learning techniques, computer vision and AI enable healthcare providers to detect subtle anomalies, identify disease patterns, and predict patient outcomes with remarkable accuracy.
Moreover, it enhances diagnostic efficiency, streamlines workflow processes, and allows clinicians to make better decisions, ultimately leading to improved patient care and outcomes.
However, the process of annotating CT scans can be complex and time-consuming, demanding a high level of expertise and precision.
This is where Labellerr, an annotation platform, comes in as a solution, revolutionizing the way CT scan analysis is conducted in the healthcare industry.
By using advanced algorithms and intuitive interfaces, Labellerr streamlines the annotation process, enabling healthcare professionals to annotate CT scans efficiently and accurately.
Challenge
One of the important challenges in CT scan analysis within the healthcare industry is the sheer volume of data that needs to be processed and interpreted accurately.
CT scans generate large datasets comprising numerous cross-sectional images, each of which must be examined properly for abnormalities or anomalies.
Manual annotation of these images is not only time-consuming but also prone to human error, leading to potential misdiagnosis or oversight of critical findings.
Additionally, the shortage of skilled people with expertise in medical image analysis further increases this challenge, resulting in delays in diagnosis and treatment.
Furthermore, the interpretation of CT scans often requires precise analysis of structures and regions of interest, which can be subjective and vary between different radiologists or medical professionals.
Achieving consistency and standardization in annotation is essential for ensuring reliable results.
Solution: Labellerr's Role in CT Scan Analysis
Intuitive Interface
Labellerr offers an intuitive user interface for annotating CT scans, simplifying the annotation process for healthcare professionals.
It has a user-friendly design and intuitive navigation, which enable users to annotate CT images efficiently and accurately, reducing the learning curve and enhancing productivity.
Robust Segmentation Features
Labellerr incorporates robust segmentation features tailored to the complexities of CT scan data.
Labellerr's advanced segmentation capabilities are powered by the Segment Anything Model (SAM), a versatile algorithm designed to annotate a wide array of objects and structures within images.
SAM excels in accurately outlining various elements, ranging from simple shapes to complex objects, with precision and efficiency. By leveraging SAM, Labellerr enables users to annotate images with high accuracy.
SAM's flexibility and accuracy make it an indispensable tool for a wide range of annotation tasks.
These advanced algorithms can accurately outline the anatomical structures, lesions, and abnormalities within CT images, providing precise annotations crucial for diagnostic accuracy and treatment planning.
Cost-Effective
Labellerr offers a cost-effective solution for CT scan annotation, minimizing the need for expensive software or outsourcing services.
Labellerss provides a scalable and affordable platform which enables healthcare organizations to optimize their resources and allocate budget more effectively towards patient care initiatives.
Custom Workflows
Labellerr supports custom workflows tailored to the specific requirements of CT scan analysis.
Users can define their annotation protocols, designate regions of interest, and customize labeling criteria to suit their unique needs.
This flexibility ensures that annotations are consistent, standardized, and aligned with clinical objectives.
Active Learning Based Labeling
Labellerr uses active learning techniques to optimize the annotation process for CT scan analysis.
By iteratively selecting the most informative samples for annotation, Labellerr maximizes the efficiency of data labeling, reducing the manual effort required while improving the performance of machine learning models trained on annotated data.
Automated Import and Export of Data
Labellerr streamlines the process of importing and exporting CT scan data with its automated functionalities.
Healthcare professionals can effortlessly upload large volumes of CT images into the platform, eliminating the need for manual data entry and saving valuable time.
Similarly, Labellerr facilitates the seamless export of annotated data, ensuring compatibility with various healthcare systems and research platforms.
Collaborative Annotation Pipeline
Labellerr allows collaboration among healthcare teams with its collaborative annotation pipeline.
Multiple users can work simultaneously on annotating CT scans, allowing for distributed workflows and real-time collaboration.
This collaborative approach enhances efficiency and accelerates the annotation process, enabling interdisciplinary teams to leverage their collective expertise for more comprehensive analysis.
Automated QA (Quality Assurance)
Labellerr integrates automated quality assurance mechanisms to ensure the accuracy and reliability of annotated CT scan data.
Advanced algorithms analyze annotations in real-time and flagging inconsistencies or errors for review by healthcare professionals.
By automating quality assurance processes, Labellerr enhances the overall reliability of annotated data, reducing the risk of diagnostic inaccuracies and improving patient care outcomes.
Conclusion
In summary, Labellerr plays an important role in the health care industry through CT scan analysis by providing an intuitive interface, robust segmentation features, cost-effective solutions, custom workflows, and active learning-based labeling capabilities.
By using these advanced functionalities, Labellerr allows healthcare professionals to annotate CT scans with precision and efficiency, ultimately enhancing diagnostic accuracy and patient care in the healthcare industry.
Frequently Asked Questions
Q1) How does AI improve diagnostic accuracy in healthcare?
AI algorithms can analyze vast amounts of medical data, including images from medical scans such as CT scans, MRIs, and X-rays, to detect patterns indicative of diseases or abnormalities.
AI can assist healthcare professionals in interpreting medical images more accurately and identifying conditions at earlier stages.
Q2) What is Labellerr, and how does it benefit CT scan analysis in the healthcare industry?
Labellerr is an advanced annotation platform designed to streamline the process of annotating CT scans and other medical imaging data.
It benefits healthcare professionals by providing them with intuitive interfaces, robust segmentation features, and collaborative workflows, which allows them to create high-quality annotated datasets, ultimately enhancing diagnostic accuracy and patient care.
Q3) Can multiple users collaborate on annotating CT scans using Labellerr?
Yes, Labellerr facilitates collaborative annotation workflows, allowing multiple users to work simultaneously on annotating CT scans.
This collaborative approach enhances efficiency and accelerates the annotation process, enabling interdisciplinary teams to leverage their collective expertise.
Book our demo with one of our product specialist
Book a Demo