Accelerate Lane Marker Detection Annotation With Labellerr
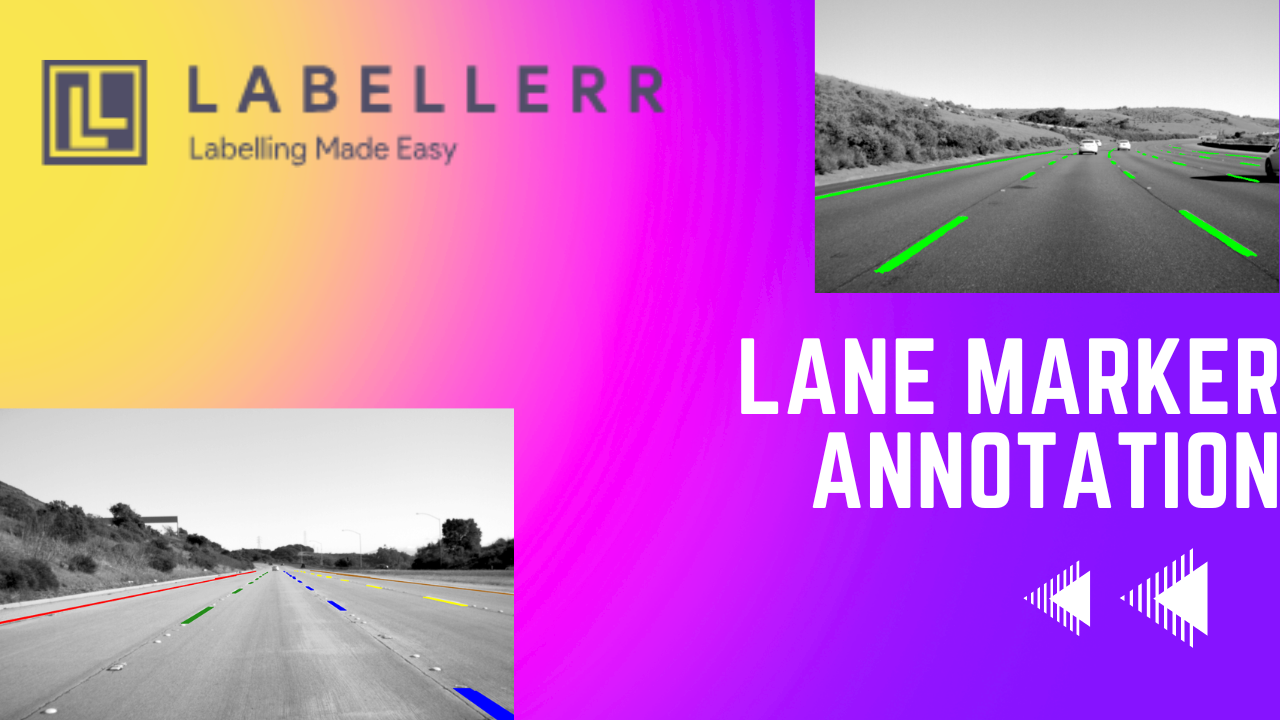
Introduction
In transportation and autonomous driving, accurate identification of road lanes is critical for ensuring vehicle safety and facilitating navigation.
Road lane detection involves the use of computer vision algorithms to analyze visual data captured by cameras mounted on vehicles and identify lane markings on the road surface.
This information is essential for various applications, including lane-keeping assistance systems, autonomous vehicle navigation, and traffic management.
Labellerr plays a crucial role in the development of road lane detection systems by providing advanced annotation capabilities for labeling lane markings in image and video data.
Challenge
Identifying road lanes using computer vision presents lots of challenges, starting with the complexity and variability of real-world road environments.
One of the primary difficulties lies in the variability of lane markings, which can consist of a diverse array of configurations such as solid lines, dashed lines, double lines, arrows, and road edge markings.
Additionally, the complexity of road layouts, including multiple lanes, intersections, merges, and diverges, poses a significant challenge for algorithms to identify lane boundaries accurately.
Annotating every image pixel-by-pixel takes significant time, and achieving perfect accuracy for every twist and turn of the lane is challenging.
Manual annotation of road lane data also presents challenges in terms of consistency and scalability, necessitating robust annotation tools and workflows to ensure accurate labeling across large datasets.
Overcoming these challenges requires advanced annotation platforms like Labellerr to streamline the annotation process, enhance accuracy, and facilitate the development of reliable road lane detection systems for safer and more efficient transportation.
Solution: Labellerr's Role in Lane Detection
Intuitive Interface
Labellerr's automated data labeling tool provides an intuitive user interface designed to simplify the annotation process for annotators working on road lane identification tasks.
Its user-friendly design and navigation tools enable annotators to label lane markings with ease, reducing the learning curve and enhancing productivity.
Using Labellerr's intuitive interface, annotators can focus on labeling tasks without being overwhelmed by the complexity of the road environment.
Robust Segmentation Features
Labellerr incorporates robust segmentation features that enable accurate and precise annotation of lane markings in complex road environments.
Its advanced algorithms can effectively delineate lane boundaries, even in challenging scenarios with noise, and adverse weather conditions.
By using Labellerr's segmentation capabilities, annotators can ensure the accuracy and reliability of labeled road lane data, enhancing the performance of machine learning models trained on these datasets.
Cost Savings
Labellerr offers significant cost savings for road lane identification tasks by streamlining the annotation process and reducing manual labor costs.
Labellerr's advanced automation features minimize the need for human intervention, allowing organizations to annotate large volumes of road lane data more efficiently and cost-effectively.
Custom Workflows
Labellerr supports customizable workflows needed to meet the specific requirements of road lane identification tasks.
Organizations can define their annotation protocols, designate lane marking types, and customize labeling criteria to suit their unique needs.
This flexibility ensures that annotations are consistent, standardized, and aligned with the objectives of the project, ultimately leading to more reliable and accurate labeled road lane datasets.
Active Learning Based Labeling
Labellerr implements active learning techniques to optimize the road lane identification process.
By intelligently selecting the most informative samples for annotation, Labellerr maximizes the efficiency of data labeling, reducing manual effort while improving the performance of trained models.
This active learning-based approach enables organizations to prioritize labeling efforts on data points that are most beneficial for model training, ultimately leading to more accurate and effective road lane detection systems.
Automated Import and Export of Data
Labellerr streamlines the process of importing and exporting data for road lane identification tasks with its automated functionalities.
Organizations can seamlessly upload large volumes of road imagery into the platform and export annotated data for compatibility with various analysis tools and frameworks.
By automating data import and export processes, Labellerr ensures seamless integration with existing workflows, maximizing workflow efficiency and productivity.
Collaborative Annotation Pipeline
Labellerr facilitates collaboration among annotators with its collaborative annotation pipeline.
Multiple users can work simultaneously on annotating road lane data, allowing for distributed workflows and real-time collaboration.
This collaborative approach enhances productivity and ensures consistency and accuracy in the labeled dataset, ultimately improving the performance of road lane detection models.
Automated QA (Quality Assurance):
Labellerr integrates automated quality assurance mechanisms to ensure the accuracy and reliability of annotated road lane data.
Advanced algorithms analyze annotations in real-time, flagging inconsistencies or errors for review by annotators.
By automating quality assurance processes, Labellerr maintains the quality of the labeled dataset, reducing the risk of errors and ensuring the high performance of trained models in real-world road environments.
Conclusion
In conclusion, Labellerr emerges as an invaluable solution for enhancing road lane identification through precise and efficient data annotation.
With its intuitive interface, robust segmentation features, and advanced automation capabilities, Labellerr streamlines the annotation process, enhances collaboration among annotators, and ensures the quality and consistency of labeled data.
Labellerr accelerates progress in the fields of transportation and autonomous driving, enabling the development of safer and more efficient road lane detection systems.
By leveraging the power of Labellerr, organizations can unlock new capabilities in vehicle navigation, lane-keeping assistance, and traffic management, ultimately contributing to safer roads and enhanced mobility for all.
Frequently Asked Questions
Q1) What is road lane identification, and why is it important?
Road lane identification is the process of detecting and delineating lane markings on road surfaces, which is crucial for tasks such as lane-keeping assistance, autonomous vehicle navigation, and traffic management. Accurate lane identification enhances road safety and facilitates efficient vehicle operation.
Q2) How does Labellerr assist in annotating road lane data?
Labellerr provides advanced annotation tools and features tailored specifically for road lane identification tasks. Its intuitive interface, robust segmentation capabilities, and automation features streamline the annotation process, enabling annotators to label lane markings accurately and efficiently.
Book our demo with one of our product specialist
Book a Demo