9 Capabilities Of DALL-E That One Must Know
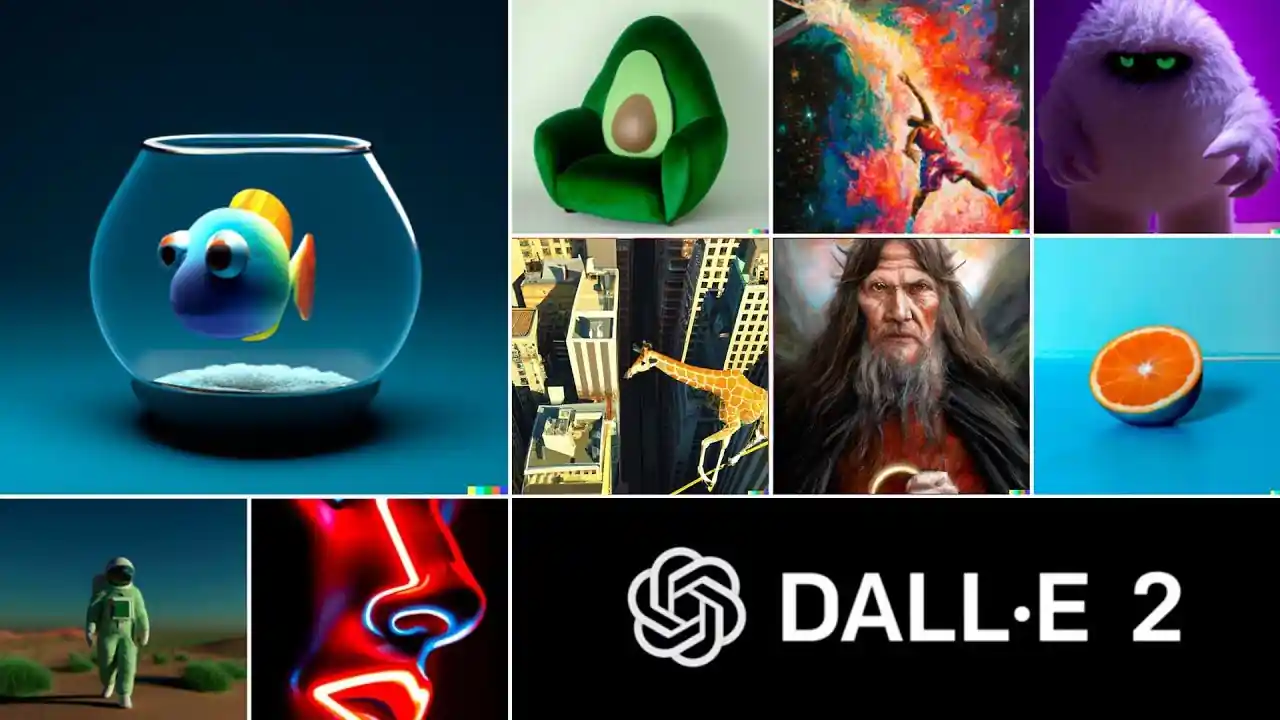
While there are other text-to-image systems (such as the tokens-based programs VQGAN+CLIP and CLIP-Guided Diffusion, which are accessible on Night Cafe), the most recent version of DALL-E is significantly better at producing coherent images.
The world and the connections between objects appear to be well understood by technology. DALL-E is able to produce stunning visuals that demonstrate the artistic potential of AI. Let’s get deeper into what DALL-E is and what its capabilities and limitations are.
About DALL-E and DALL-E 2
DALL-E and DALL-E 2 are deep learning techniques created by OpenAI that produce digital images from "prompts," or natural language descriptions. DALL-E, which makes use of a GPT-3 variant that has been altered to produce images, was unveiled by OpenAI in a blog article in January 2021.
In April 2022, OpenAI unveiled DALL-E 2, a replacement that "can mix concepts, traits, and styles" to produce more realistic graphics at higher resolutions.
Both models' source codes are not publicly available thanks to OpenAI. Invitations to the beta phase of DALL-E 2 were distributed to 1 million people on the waitlist on July 20, 2022.
People can create a set amount of photographs each month for free, and more images can be purchased. Because of worries about safety and ethics, accessibility had previously been limited to people who had been carefully chosen for a study preview.
DALL-E 2 became publicly accessible and the queue requirement was dropped on September 28, 2022.
DALL-E 2 was made available as an API by OpenAI at the beginning of November 2022, enabling programmers to incorporate the model within their own applications.
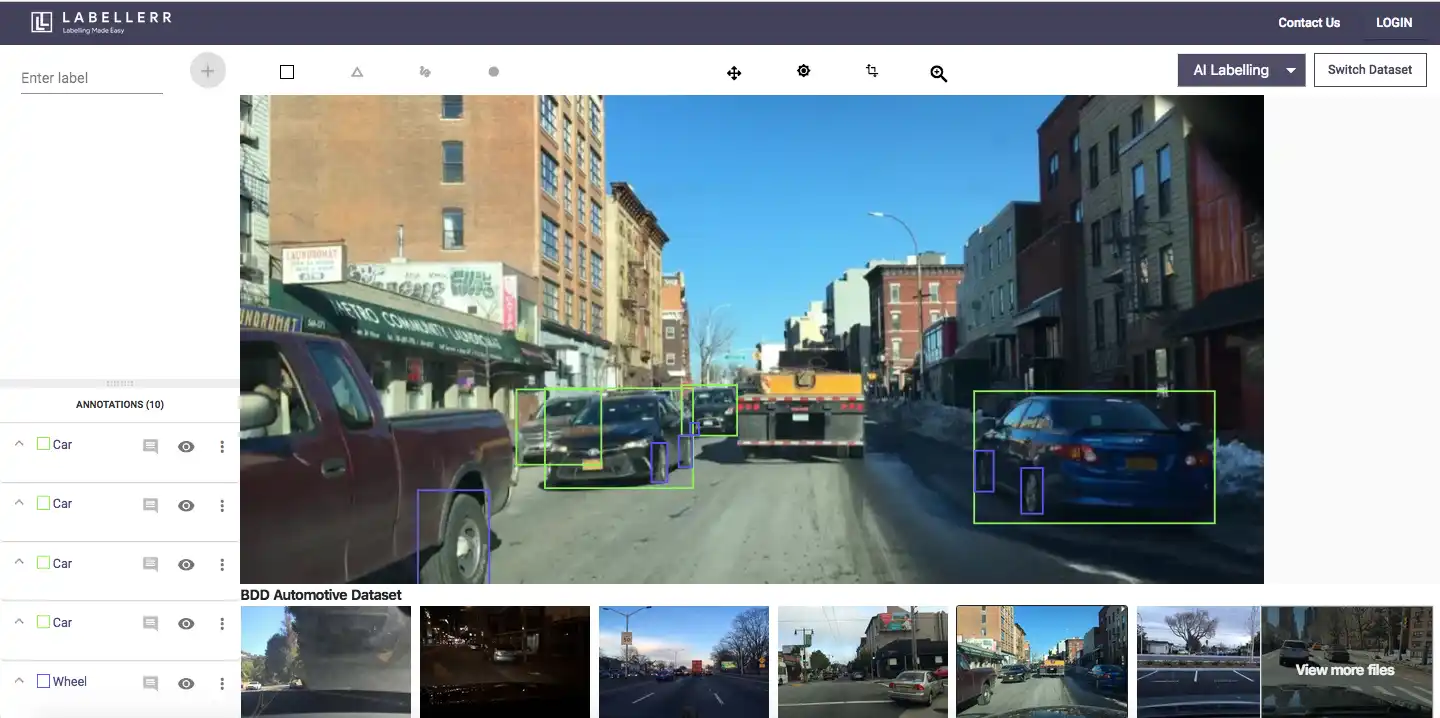
Image Labeling Using Synthetic Data
Labellerr annotation tool helps teams with small datasets to build production-ready AI with its synthetic data generator. Try now, no signup is required!
DALL-E 2 was implemented by Microsoft in their Designer app and the Image Creator feature found in Bing and Microsoft Edge. Among many other beta testers of the DALL-E 2 API is CALA and Mixtiles. The cost per image for the API varies depending on the resolution of the image.
Companies collaborating with OpenAI's enterprise team can take advantage of volume reductions.
Overview
The transformer language model DALL-E is similar to the GPT-3. It accepts the text and the image as a single data stream with up to 1280 tokens, and it is trained to produce each token one at a time using maximum likelihood.
OpenAI acknowledges the potential for major, widespread societal implications of work employing generative models. They intended to examine the relationship between models like DALL-E and social difficulties in the future, including the economic impact on particular work processes and professions, the possibility of bias in the model results, and the longer-term ethical challenges posed by this technology.
Capabilities
DALL-E is capable of producing images in a variety of genres, such as paintings, emojis, and photo-realistic artwork. Without explicit guidance, it can appropriately position design elements in creative compositions and "manipulate and rearrange" things in its images.
In his article for BoingBoing, Thom Dunn noted that DALL-E frequently drew the handkerchief, hands, and feet in credible locations when asked to draw a daikon radish trying to blow its nose, sipping a latte, or riding a unicycle.
DALL-E also demonstrated the ability to "fill in the blanks" to surmise appropriate details without specific prompts, such as by adding Christmas imagery to prompts usually associated with the holiday appropriately- DALL-E also demonstrates a thorough awareness of current trends in both aesthetics and design.
With only a few exceptions, DALL-E can create visuals for a wide range of arbitrary descriptions from different angles. Associate professor Mark Riedl of the Georgia Tech School of Interactive Computing discovered that DALL-E might combine ideas (described as a key element of human creativity).
It can solve Raven's Matrices using only its visual reasoning abilities (visual tests often administered to humans to measure intelligence).
1. Managing Attributes
The ability of DALL-E to change the number of times an object appears as well as the number of its properties.
2. Drawing a number of objects
A new issue arises when trying to handle many objects, their characteristics, and their spatial arrangement all at once. Think of the sentence "a hedgehog wearing a scarlet hat, yellow gloves, blue shirt, and green pants," as an example. DALL-E must accurately associate each article of clothing with the animal in order to successfully grasp this statement.
She must also make the connections (hat, red), (gloves, yellow), (shirt, blue), and (pants, green) without confusing them.
Variable binding is the term for this activity, which has been thoroughly researched in the literature.
17181920
For position determination, stacking objects, and managing many characteristics, Open-AI examined DALL-E's capability to do this.
Although DALL-E does provide some degree of maneuverability over the characteristics and locations of a select few items, the degree of success can vary depending on how the description is written.
The success rate rapidly declines as the number of objects is increased because DALL-E is prone to misinterpreting the relationships between both the objects and their colors. It should be emphasized that in these situations, DALL-E is fragile when it comes to rephrasing the caption because the alternative, semantically comparable captions frequently don't result in the right readings.
3. Three-dimensionality and perspective visualization
DALL-E further gives users control over a scene's viewpoint and 3D rendering style.
To take things a step further, Open-AI tests DALL-E's capacity to repeatedly sketch a famous figure's head at every angle from a series of evenly spaced angles and discovers that they are able to restore a fluid motion of the revolving head.
As seen by the choices "fisheye lens view" and "a spherical panorama," DALL-E looks to be able to apply certain optical distortions to scenes. This inspired us to investigate its capacity to produce reflections.
4. Visualizing Structure Both Internal and External
They looked deeper into DALL-E's capability to portray interior structure with cross-sectional perspectives and external structure with macro images as a result of the samples from the "extreme close-up view" and "x-ray" styles.
5. Speculating Contextual Information
The problem of converting words to images is not well defined because there are essentially an infinite number of credible images that can be selected from a single caption.
Take the phrase "a painting of a capybara sitting on a field at sunrise," for example. Though this information is never officially stated, it might be essential to generate a shadow depending on the capybara's orientation.
Changing style, place, and time; depicting the same object in a range of different contexts; and creating a picture of an object with precise text written on it are three scenarios in which we investigate DALL-E's capacity to address under specification.
DALL-E allows access to a subset of a 3D rendering engine through natural language, with variable degrees of reliability. It has a limited ability to autonomously manage how many objects there are, how they are ordered in relation to one another, and the qualities of a select few of those things.
Additionally, it has the ability to manage the viewpoint and angle from which a scene is created as well as create recognized objects that adhere to very specific lighting and viewpoint requirements.
Contrary to a 3D rendering engine, whose inputs must be unambiguously and in great detail specified, DALL-E is frequently able to "fill in the blanks" when the caption indicates that the image must contain a specific detail that is not expressly expressed.
6. Combining Divergent Ideas
Because language is composed, we can combine ideas to describe both actual and made-up objects. It was discovered that DALL-E also had the capacity to synthesize items, a few of which are highly improbable to exist in the actual world. Two examples of this skill were given: giving animals traits from different conceptions and developing items by drawing ideas from unconnected concepts.
7. Illustrations of animals
DALL-E's capacity to generate visuals of real-world objects by combining seemingly unrelated concepts in the preceding section. Here, the team examined this skill in relation to three types of illustrations: anthropomorphized representations of things and animals, animal chimeras, and emojis.
8. Immediately Visual Reasoning
Without any additional training, GPT-3 may be made to carry out a variety of activities simply by being given a description and a signal to produce the response specified in its prompt. For instance, GPT-3 responds "un homme qui promène son chien dans le parc" when asked to translate the phrase "here is the sentence "a person walking his dog in the park" into French." Zero-shot reasoning is the term for this skill. We discover that DALL-E extends this ability to the visual realm and, when appropriately prodded, is capable of carrying out a variety of image-to-image translation tasks.
9. Geographical Information
DALL-E has gained knowledge of geographical information, landmarks, and localities. Its understanding of these ideas is both remarkably accurate and incorrect in different ways.
Technical Limitations of DALL-E
Language comprehension in DALL-E 2 has its limitations. Sometimes it cannot tell the difference between "A yellow book and a red vase" and "A red book and a yellow vase," or between "A panda doing latte art" and "Panda's latte art."
When given the suggestion "a horse riding an astronaut," it produces visuals of "an astronaut riding a horse."
Additionally, it frequently fails to provide the right photos. Requesting more than three objects, using a negation, numbers, or related words may lead to errors, and the incorrect item may display object features.
Other drawbacks include the inability to handle scientific data, such as that related to astronomy or medical images, and managing writing, which, even with readable lettering, almost always produces nonsense that resembles dream-like language.
Conclusion
DALL-E is a straightforward decoder-only transformer that models each of the 1280 tokens—256 for the text and 1024 for the image—auto-regressive as it receives both the text and the picture as a single stream.
Each picture token is able to attend to every text token thanks to the attention mask at every one of its 64 self-attention layers. For the textual tokens, DALL-E uses the conventional causal mask, and for the image tokens, it employs sparse attention with either rows, columns, or convolutional concentration patterns, based on the layer.
Book our demo with one of our product specialist
Book a Demo