Accelerate Car Damage Annotation With Labellerr's Auto Labeling
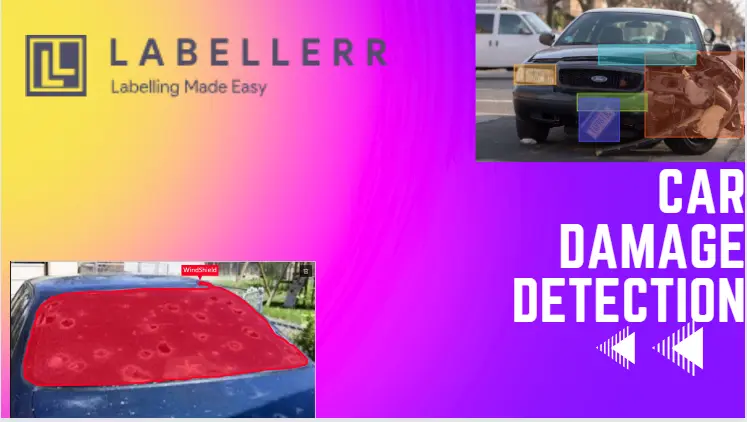
Introduction
In the automotive industry, assessing vehicle damage after accidents is a critical task for insurance claim processing, maintenance planning, and safety evaluations.
Traditional methods of inspecting car damage rely heavily on manual assessment by trained professionals, which can be time-consuming, subjective, and prone to errors.
However, with the advancement of Computer Vision technology, automated car damage detection is possible.
Computer Vision systems can analyze images of damaged vehicles with remarkable precision and efficiency.
Challenge
One of the primary challenges in car damage detection using Computer Vision is the variability and complexity of damage types and patterns.
In car damage segmentation, we're interested in going beyond simply identifying that a car is present in an image.
The focus here is on pinpointing the exact areas of damage to the vehicle. This could include dents, scratches, cracks, or even broken windows.
By segmenting the image, we aim to create a mask that precisely outlines the damaged regions.
This pixel-level breakdown allows for a detailed understanding of the car's condition, providing valuable information for tasks like insurance claims processing, and auto body repair estimates.
Moreover, environmental factors such as lighting conditions and camera angles can further complicate the analysis process, making it challenging to develop robust and accurate Computer Vision models for car damage detection.
Another challenge is the need for large and diverse annotated datasets to train and validate Computer Vision models effectively.
Manual annotation methods are labor-intensive and time-consuming, hindering the scalability and efficiency of dataset creation.
Additionally, ensuring consistency and quality in annotations across different annotators and datasets poses a significant challenge, impacting the reliability and performance of trained models.
Solution: Labellerr's Role in Car damage Detection
Intuitive Interface
Labellerr offers an intuitive user interface designed specifically for car damage detection, simplifying the annotation process for users.
With its user-friendly design and intuitive navigation, Labellerr ensures that annotators can easily label car damage images with precision and accuracy.
The intuitive interface reduces the learning curve for users, enabling them to focus on the task at hand and streamline the annotation process effectively.
Robust Segmentation Features
Labellerr incorporates robust segmentation features tailored to the complexities of car damage images.
Labellerr's uses the cutting-edge Segment Anything Model (SAM), a state-of-the-art algorithm designed to annotate a wide range of objects and structures within car images.
SAM excels at accurately delineating various types of car damage, including scratches, dents, and structural deformations, making it a versatile tool for car damage detection tasks.
With SAM's advanced capabilities, Labellerr allows users to annotate car damage images with precision and efficiency, driving innovation and advancement in the automotive industry.
Labellerr's segmentation capabilities allow annotators to easily highlight different types of damage, enhancing the accuracy and reliability of annotated datasets for training Computer Vision models.
Automated QA (Quality Assurance)
Labellerr incorporates automated quality assurance mechanisms to ensure the accuracy and reliability of annotated car damage images.
Advanced algorithms analyze annotations in real-time, flagging inconsistencies or errors for review by annotators.
Labellerr enhances the overall reliability of annotated data, reducing the risk of false positives and improving the performance of trained Computer Vision models.
Collaborative Annotation Pipeline
Labellerr fosters collaboration among annotators with its collaborative annotation pipeline.
Multiple users can work simultaneously on annotating car damage images, allowing for distributed workflows and real-time collaboration.
This collaborative approach enhances productivity and accelerates dataset creation, enabling organizations to build comprehensive annotated datasets for training and validating Computer Vision models.
Automated Import and Export of Data
Labellerr streamlines the process of importing and exporting car damage image data with its automated functionalities.
Users can effortlessly upload large volumes of images into the platform and export annotated data for compatibility with various Computer Vision frameworks and analysis tools.
By automating data import and export processes, Labellerr ensures seamless integration with existing workflows and maximizes workflow efficiency.
Active Learning Based Labeling
Labellerr leverages active learning techniques to optimize the annotation process for car damage detection.
Labellerr maximizes the efficiency of data labeling and reduces the manual effort while improving the performance of trained Computer Vision models.
This active learning-based approach enables organizations to prioritize labeling efforts on images that are most beneficial for model training, enhancing the overall accuracy and effectiveness of car damage detection algorithms.
Custom Workflows
Labellerr provides customizable workflows tailored to the specific requirements of car damage detection applications.
Users can define their annotation protocols, designate damage categories, and customize labeling criteria to suit their unique needs.
This flexibility ensures that annotations are consistent, standardized, and aligned with the specific use case, ultimately leading to more reliable and accurate Computer Vision models for car damage detection.
Cost-Effective
Labellerr offers a cost-effective solution for car damage detection annotation, minimizing the need for expensive proprietary software or outsourcing services.
By providing a scalable and affordable platform, Labellerr enables organizations to optimize their resources and allocate budget more effectively towards developing robust Computer Vision models for car damage detection.
The cost-effectiveness of Labellerr makes it accessible to a wide range of users, including small businesses, insurance providers, and automotive manufacturers, facilitating widespread adoption and implementation of car damage detection solutions.
Conclusion
In conclusion, Labellerr emerges as an invaluable tool in the domain of car damage detection, offering a comprehensive solution for annotating images with precision and efficiency.
Labellerr allows users to streamline the annotation process, enhance the accuracy of annotated datasets, and accelerate the development of computer vision models for car damage detection.
By using Labellerr, organizations can unlock new opportunities for improving efficiency, enhancing customer satisfaction, and ultimately, improving the way car damage is assessed and managed.
Frequently Asked Questions
Q1) What is car damage detection using Computer Vision?
Car damage detection using computer vision involves the use of advanced algorithms and image analysis techniques to automatically identify and assess damage to vehicles from visual data such as images or videos.
By analyzing features such as scratches, dents, or structural deformations, computer vision models can accurately detect and classify different types of damage, aiding in insurance claim processing, vehicle repairs, and safety inspections.
Q2) What is Labellerr, and how does it contribute to car damage detection?
Labellerr is an annotation platform designed to streamline the process of annotating images for computer vision models. It can accurately help in labeling of damaged areas, scratches, and dents, contributing to the development of robust algorithms for car damage detection.
Book our demo with one of our product specialist
Book a Demo