Manual or automated data labeling, how to decide?
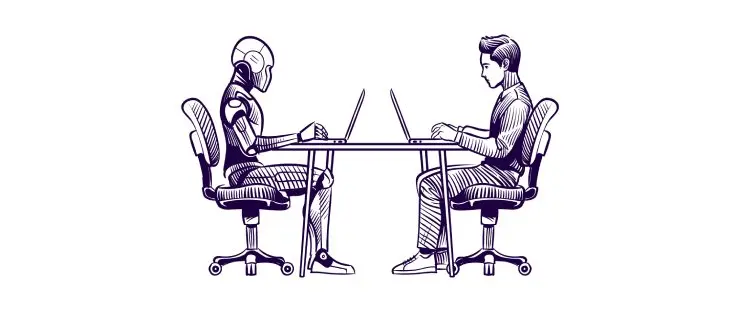
The foundation for machine learning and deep learning revolutions is accurately labeled datasets. In order to attain an efficient Ai model, enormous amounts of trained datasets are needed (AI) for a model to be accurate. Computer vision systems can consistently discern between a pedestrian and a stop sign with the help of properly tagged images. Another option is to choose between a car and a bus.
How can we create the precise, scalable datasets that the market demands? We must first compare manual vs. automated data labeling before we can begin to respond to this question.
In this blog, we have listed the pros and cons of both types of labeling options. Let’s check them out.
What is manual data labeling?
During segmentation in image processing, manual data labeling often refers to individual annotators identifying items in image or video frames. These experts go through tens of thousands of photographs in an effort to compile thorough, high-quality training data for AI.
Depending on the requirements of every image annotation project, specific labeling approaches and types of picture annotation are used on the raw data. These methods consist of:
Annotation tool for bounding boxes: To help an AI recognize and avoid an object in an image, a rectangle is painted around it. Due to its greater simplicity and hence higher cost-effectiveness in real-time, this approach is more popular.
Annotation of polygons: In this situation, the annotator must plot the vertices surrounding an object to more precisely capture its outline.
Semantic segmentation: This method is used to group objects in an image together, such as dividing roads from buildings. Because it requires more precision, this kind of labeling is also more challenging.
Manual data labeling could require a fair amount of labor. It could take a few seconds to label each item. Having hundreds of photographs may have a variable that can be manipulated by building up a backlog and delaying a project.
Manual data labeling
What are some pros of manual data labeling?
More precise outcomes
Human annotators are your go-to resources for any firm when it comes to labeling data with accuracy and quality. These professionals have years of experience labeling data and comprehending the needs of various machine learning models. They can also spot irregularities that automated systems might otherwise miss. Labeled features that are consistent with actual environmental conditions will be more accurate when used to create computer vision or natural language processing (NLP) models.
Easier to modify
Human expertise in data labeling and annotation is better able to adapt to changing company needs and goals. They can therefore adapt to product changes, data model changes, and adjustments that are tailored to the demands of your end customers. Due to their adaptability, they can easily switch gears and take on data annotation projects that are tailored to your unique business requirements.
Improved data quality control
The most important factor in determining the precision of the labeling is data quality. Only the authorized items are released for analysis after being reviewed by skilled individual data labellers for quality. This always guarantees the accuracy and quality of model training datasets. Consider the task of labeling the many parts of a car as an illustration. Automated labeling tools would miss the object's edge cases, whereas manual labeling methods are better suited to do so.
More robust data security
Organizations can maximize data security using in-house data labeling since they maintain control over their data. The risk of data leakage for your company is greatly reduced with an appropriate and effective security system and protocol.
What are some cons of manual data labeling?
Slower
When your business relies on human specialists, labeling large data takes time and effort. One of the main obstacles stopping businesses from manually labeling data is this. Consider the scenario if your business wanted to analyze the sentiment in the online reviews left by its clients. Imagine that your business wants to create a correct data model using 90,000 reviews. A labeler will need 750 hours to complete the work if they take 30 seconds to label each comment.
What is automated data labeling?
Simply put, automated data labeling is labeling that is not done by a person. The labels that should be applied to which data points are recognized by machine learning models through self-training. The labeling guidelines for both objects and data sets must be learned by the model on its own.
These models can sense, reason, act, and adjust based on experience using machine learning algorithms, closely resembling the functioning of the human brain. To identify customer segments with comparable combinations of features and treat them equally in marketing campaigns, automated data labeling, for example, can be used with any unstructured consumer data or content.
Automated data labeling
What are some pros of automated data labeling?
Speedy and cost saving
Automated data labeling requires minimal (or no) human involvement, saving firms a lot of money on operational expenses and time that would otherwise be spent on hiring technical consultants or assembling an internal team.
Improved accuracy in learning and development
Automated data labeling produces extremely accurate data annotation through active learning, a semi-supervised method. The labeler must first choose a sample from the unlabeled data for active learning, then subsequently label further data in response to the findings. Additionally, you can use automation to keep enhancing and bettering your human data labeling procedures.
What are some cons of automated data labeling?
Difficulties labeling data that is not visible
When you only employ automatic labeling, machine learning models are trained using the sample datasets that are readily available. External to the sample set items and data points may not have accurate labels. Such unexpected or unprepared scenarios can be handled by human professionals.
Chance of future mistakes
Future mistakes sometimes happen and go undiscovered when a data point is improperly classified since the machine learning algorithm is being trained using inaccurate results from the past. The effectiveness of downstream operations and the precision of predictive models will suffer as a result.
We understand that you are confused about which option to choose. The first, and most important factor when it comes to training a model is accuracy, and the second most is effectiveness. Whichever option you feel is suitable according to your requirements, you should go for that option. We at Labellerr offer you accuracy and effectiveness at the same time. Our platform helps ML team to chose the best option suited for their requirement. Along with automation and optimization to the human-in-the-loop component.
To know more about us, visit our website.
Book our demo with one of our product specialist
Book a Demo