How Computer Vision Based Vehicle Surveillance Enhance Road Safety
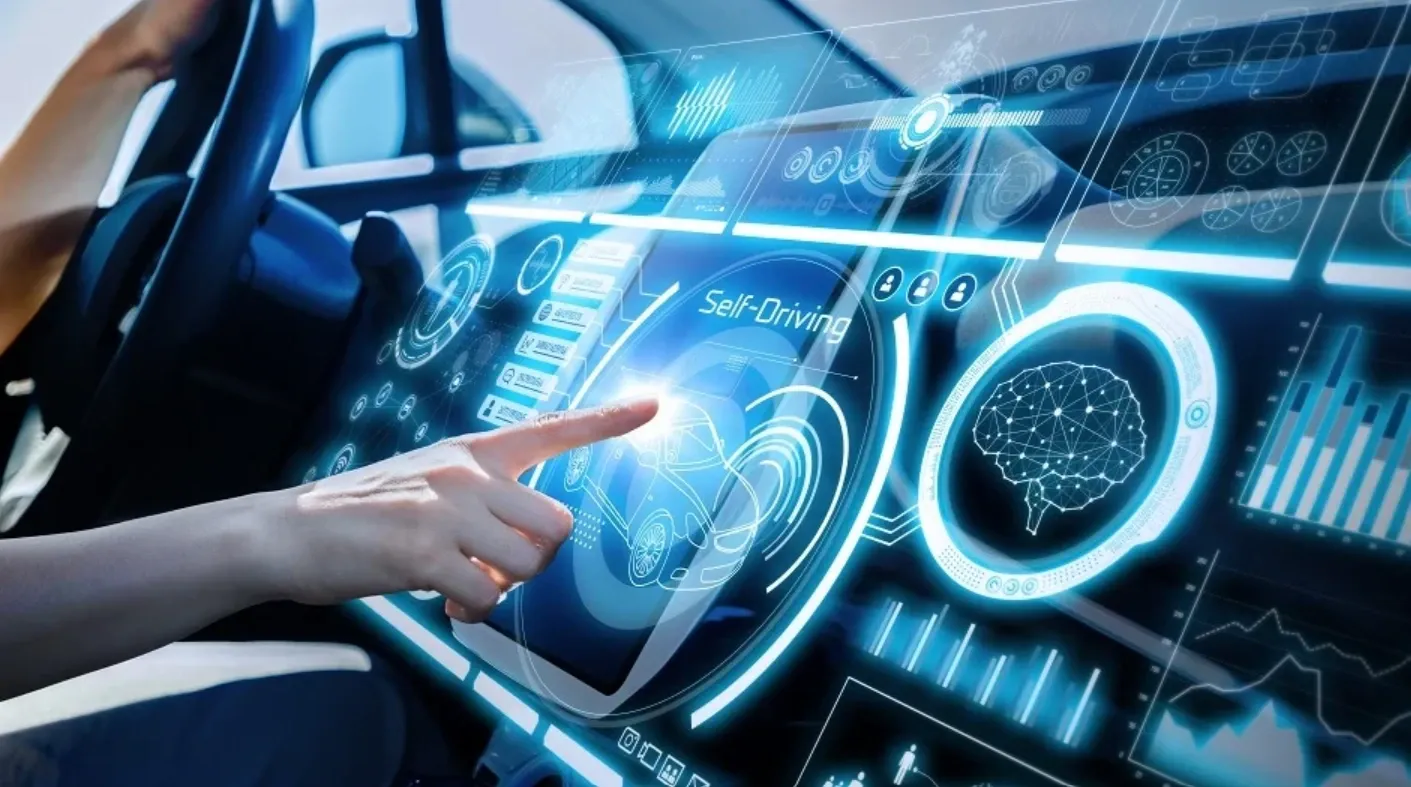
Introduction
Making sure our roads are safe is a big job, just like how we keep our play area safe.
Did you know that sometimes, it can be a bit tricky to make sure everyone is driving safely on the roads?
The challenge we face is that there are many cars, and we need to make sure they all follow the rules. That's where something called "Computer Vision" comes in to help!
When vehicle surveillance used computer vision, the number of accidents was reduced by almost 30%. It's like making the roads three times safer!
And not just that – computer vision can recognize things on the road with amazing accuracy, up to 90% of the time!
This means it can identify if a person is crossing the road or if there's an obstacle in the way. This helps the cars make quick decisions to avoid accidents.
When you're in a car, there's a smart system powered with the help of computer vision inside it. This system can see things on the road, just like you can see your toys in your room.
It helps the car know if there's something in the way, like another car or a big object.
But it's not just one car that can do this. Many cars and even traffic lights can use computer vision.
They talk to each other, like friends sharing information, to make sure everyone knows what's happening on the road. This way cars can drive without bumping into each other, making the roads safer.
So, by using Computer Vision, we can make our roads safer and help cars be more efficient.
This blog meets the demands of efficiency-driven product managers, cutting-edge ML subject matter experts, ML Engineers and explores how Labellerr helps to create a large volume of training data necessary to build high high-performing ML model to make it possible.
Table of Contents:
(i) The Intersection of Vehicle Surveillance and Computer Vision
(ii) Benefits of Integrating Computer Vision in Vehicle Surveillance
(iii) Applications of Computer Vision in Vehicle Surveillance
(iv) Importance of Vehicle Surveillance Techniques
(v) How to Use Vehicle Surveillance Techniques
(vi) Synergizing Vehicle Surveillance and Computer Vision for HUMINT
(vii) How Labellerr Helps
The Intersection of Vehicle Surveillance and Computer Vision
Computer vision, a field within artificial intelligence, empowers machines to interpret and make decisions based on visual data.
This refers to the capacity to interpret and evaluate visual data obtained from cameras and other imaging equipment in the context of vehicle surveillance.
Computer vision algorithms can identify and track vehicles, discern patterns of movement, and even recognize license plates or other distinguishing features.
This level of automation and analysis is a game-changer in intelligence gathering, providing a more efficient and precise means of monitoring and extracting information from vehicular activities.
Benefits of Integrating Computer Vision in Vehicle Surveillance
(i) Automated Object Recognition
Computer vision enables automated recognition of vehicles, streamlining the surveillance process and reducing the manual workload.
This allows for more extensive coverage and faster response times.
(ii) Behavioral Analysis
Advanced computer vision algorithms can analyze the behavior of vehicles, detecting anomalies or suspicious patterns.
This proactive approach enhances the ability to identify potential threats or criminal activities in real-time.
(iii) LPR technology
LPR is a subset of computer vision that can automatically read and interpret license plate numbers.
This feature is invaluable in tracking specific vehicles of interest and establishing connections in complex intelligence operations.
(iv) Enhanced Data Analytics
Computer vision facilitates the extraction of meaningful insights from large volumes of visual data.
Patterns, trends, and correlations can be identified, contributing to more informed decision-making processes.
Applications of Computer Vision in Vehicle Surveillance
(i) Smart Traffic Monitoring
Computer vision algorithms can monitor traffic flow, identify congested areas, and optimize traffic management systems.
This not only aids in intelligence collection but also contributes to urban planning and public safety.
(ii) Border Security
In border surveillance, computer vision enhances the monitoring of vehicular movements, enabling authorities to detect and respond to illegal border crossings or suspicious activities more effectively.
(iii) Critical Infrastructure Protection
Computer vision can be employed to safeguard critical infrastructure such as airports, seaports, and government facilities.
The technology can identify unauthorized vehicles and assess potential security threats.
(iv) Event Security
During large-scale events, computer vision-equipped surveillance systems can analyze crowd movements, identify potential security risks, and enhance overall event security.
Importance of Vehicle Surveillance Techniques
Vehicle surveillance techniques play a pivotal role in intelligence gathering and collection operations.
Tracking the travels of people or organizations engaged in illegal operations, such as drug trafficking, terrorism, and money laundering, becomes critical in the field of HUMINT.
These techniques empower law enforcement agencies to detect and prevent crimes before they occur.
One of the key advantages of vehicle surveillance is its ability to gather evidence without alerting suspects.
Deploying well-placed cameras or GPS trackers on suspect vehicles provides valuable information about their whereabouts and activities.
Additionally, vehicle surveillance enables simultaneous monitoring of multiple targets, enhancing the efficiency of intelligence operations while minimizing manpower requirements.
Real-time updates on target locations and behaviors further amplify the significance of vehicle surveillance.
This agility allows investigators to respond swiftly when necessary, enhancing situational awareness and mitigating risks associated with traditional undercover operations.
How to Use Vehicle Surveillance Techniques
Effectively utilizing vehicle surveillance techniques involves strategic considerations. Understanding the purpose of surveillance is very important in determining the best methods for gathering information.
Blending in with traffic, driving inconspicuous vehicles, and obeying traffic laws help avoid drawing attention. In multi-vehicle operations, one can follow the target, while another maintains distance or acts as a backup.
Placing GPS trackers on the target's vehicle allows remote monitoring without risking detection. Vigilance is key, with constant scanning of surroundings for potential threats or obstacles.
Organization and meticulous documentation of observations are essential for successful intelligence gathering through human intelligence.
Synergizing Vehicle Surveillance and Computer Vision for HUMINT
The combination of vehicle surveillance and computer vision creates a powerful synergy for HUMINT operations.
As vehicles move through urban landscapes or remote locations, computer vision technologies can track and analyze their movements seamlessly.
This real-time analysis provides intelligence agencies with immediate insights, allowing for quicker response times and more informed decision-making.
Moreover, the integration of computer vision in vehicle surveillance contributes to the discreet nature of intelligence operations.
Automated processes reduce the risk of human error and enhance the ability to covertly monitor targets without raising suspicion.
The result is a more agile and effective intelligence-gathering process that aligns with the ever-evolving demands of the modern world.
How Labellerr Helps
As the above picture illustrates, Labellerr excels at identifying vehicles (car and truck) for road safety and surveillance in pictures.
Using its smart labeling engine, Labellerr has analyzed the image, accurately identified the car and truck.
Labellerr's foundation model based auto labeling capability helps it to spot car, bikes, trucks etc. ensuring road safety.
By harnessing the foundation model's deep learning capabilities, Labellerr ensures that its assessments are not only fast but also highly reliable.
Labellerr offers the flexibility of manual annotation for situations where higher accuracy is crucial.
This combination of automated efficiency and the option for manual refinement makes it a versatile and powerful platform.
Labellerr's data engine helps ML teams to create a large volume of high quality training data that need to train the AI model to detect road safety.
It provide a comprehensive solution which include pipeline management, workflow automation, pre-trained models and efficient human in the loop to generate labels at much faster speed.
Speed and quality of labels ensure organization developing surveillance models to launch their product at a much faster speed.
Here's in-depth how it works:
(i) Connect Data
Labellerr can take all the pictures and videos you have, like from cameras on the road, and put them in one place on the internet.
It can use popular cloud services like AWS, GCP, or Azure.
(ii) Creating and Managing Projects
It helps to organize pictures and videos into different projects. It makes it easy to work on many tasks without getting confused.
(iii) Labeling Engine
Labellerr has a smart labeling engine. It can quickly look at pictures and videos and point out important things for vehicle surveillance.
It helps in identifying license plates on vehicles, the distance between both objects and vehicles, road damage inspection in the pictures which aid in road safety.
This helps a lot because you don't have to look at every single picture yourself.
(iv) Easy to Understand
It can show the results in simple ways, like lists or pictures, so you can easily understand what's happening.
(v) Reducing Time and Costs
Labellerr is super fast and makes the work easier. It helps people do their tasks 10 times quicker, which saves a lot of time and money.
(vi) Helping All Types of Data
It can work with all sorts of things - pictures, videos, PDFs, text, and even sounds! You don't need different tools for different jobs.
Conclusion
Integrating computer vision technologies and vehicle surveillance tactics represents an innovative leap in intelligence collection.
These two components work in conjunction to improve intelligence agencies' capabilities and give improved and effective HUMINT operations.
With the advent of the digital age, we must make responsible use of these developments, protecting people's right to privacy and making sure new technologies are used in a morally and legally responsible manner.
The seamless fusion of conventional surveillance techniques with the revolutionary potential of computer vision represents the intelligence gathering of the future.
Frequently Asked Questions
1. What is vision-based vehicle detection system for intelligent car driving?
A vision-based vehicle detection system for intelligent car driving relies on computer vision technologies to analyze visual data captured by cameras mounted on the vehicle.
This system employs algorithms to detect and identify surrounding vehicles in real-time, enabling the vehicle to make informed decisions based on its awareness of the traffic environment.
The technology involves object recognition, tracking, and often includes features like lane departure warnings and collision avoidance.
By interpreting visual cues, this system enhances the vehicle's situational awareness, contributing to the development of autonomous driving capabilities and improving overall road safety.
2. How can video surveillance improve traffic safety?
Video surveillance can significantly enhance traffic safety by providing real-time monitoring and analysis of road conditions.
Cameras strategically placed at intersections, highways, and busy thoroughfares enable authorities to detect traffic violations, accidents, or congestion promptly.
This information allows for swift response measures, such as dispatching emergency services or adjusting traffic signal timings to alleviate congestion.
Video surveillance also serves as a deterrent to reckless driving and can aid in post-incident investigations by providing visual evidence.
Overall, the continuous monitoring facilitated by video surveillance systems contributes to proactive traffic management, reduces response times to incidents, and promotes a safer road environment.
3. Can CNN-based vehicle detection system improve traffic safety?
Yes, a Convolutional Neural Network (CNN)-based vehicle detection system can significantly enhance traffic safety.
CNNs excel at image recognition tasks, making them well-suited for analyzing visual data from cameras to detect and track vehicles on the road.
By leveraging deep learning techniques, these systems can accurately identify various aspects of traffic, such as vehicle positions, speeds, and potential hazards.
Implementing a CNN-based vehicle detection system enables real-time analysis of traffic conditions, allowing for immediate response to incidents, adaptive traffic signal control, and the potential for advanced driver assistance systems.
This technology contributes to proactive traffic management, reducing the risk of accidents and improving overall road safety.
Book our demo with one of our product specialist
Book a Demo